Synthetic And Private Smart Health Care Data Generation Using Gans
30TH INTERNATIONAL CONFERENCE ON COMPUTER COMMUNICATIONS AND NETWORKS (ICCCN 2021)(2021)
摘要
With the rapid advancements in machine learning, the health care paradigm is shifting from treatment towards prevention. The smart health care industry relies on the availability of large-scale health datasets in order to benefit from machine learning-based services. As a consequence, preserving the individuals' privacy becomes vital for sharing sensitive personal information. Synthetic datasets with generative models are considered to be one of the most promising solutions for privacy-preserving data sharing. Among the generative models, generative adversarial networks (GANs) have emerged as the most impressive models for synthetic data generation in recent times. However, smart health care data is attributed with unique challenges such as volume, velocity, and various data types and distributions. We propose a GAN coupled with differential privacy mechanisms for generating a realistic and private smart health care dataset. The proposed approach is not only able to generate realistic synthetic data samples but also the differentially private data samples under different settings: learning from a noisy distribution or noising the learned distribution. We tested and evaluated our proposed approach using a real-world Fitbit dataset. Our results indicate that our proposed approach is able to generate quality synthetic and differentially private dataset that preserves the statistical properties of the original dataset.
更多查看译文
关键词
Generative adversarial networks, differential privacy, synthetic data generation, smart health care, fitness trackers
AI 理解论文
溯源树
样例
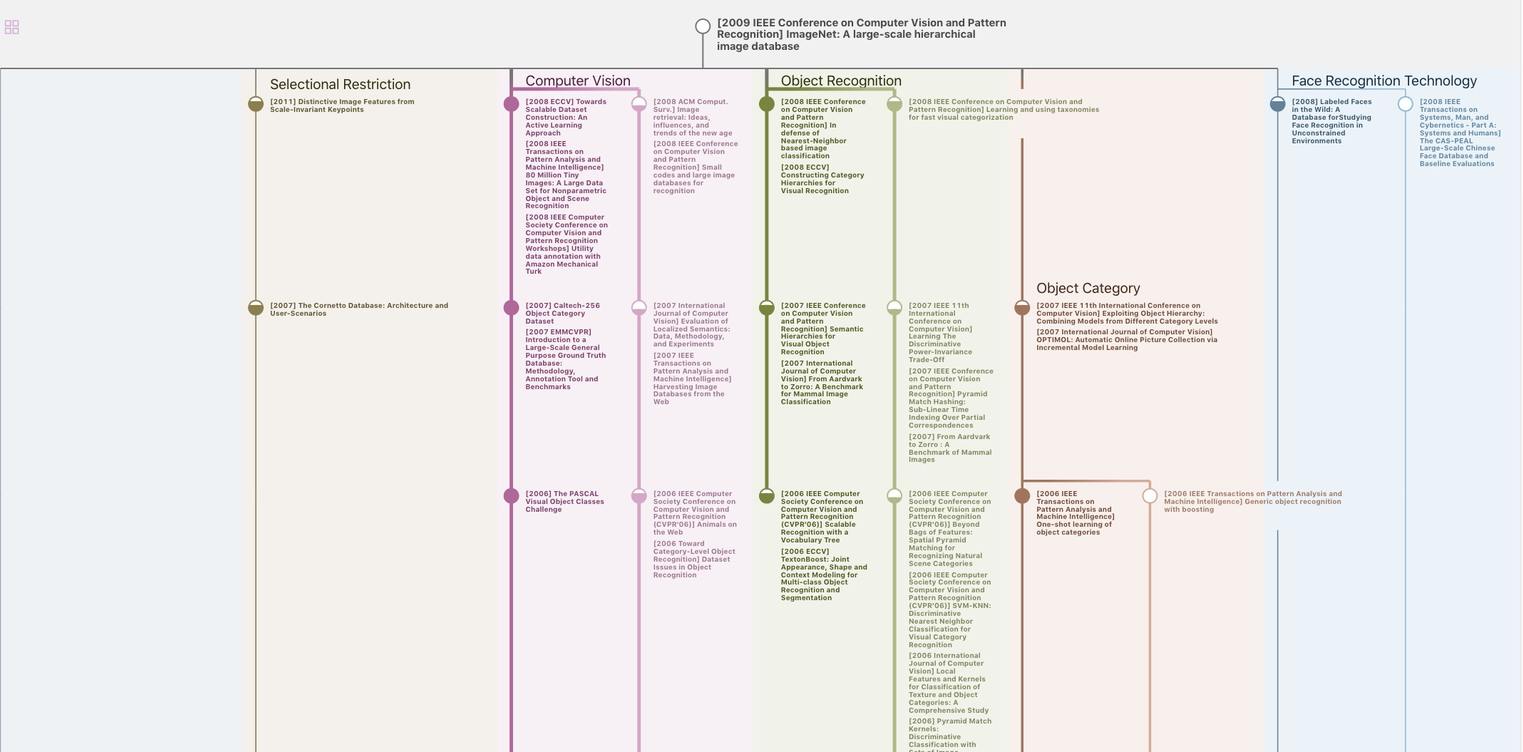
生成溯源树,研究论文发展脉络
Chat Paper
正在生成论文摘要