Towards Learning a Joint Representation from Transformer in Multimodal Emotion Recognition
BRAIN INFORMATICS, BI 2021(2021)
摘要
Emotion recognition has been extensively studied in a single modality in the last decade. However, humans express their emotions usually through multiple modalities like voice, facial expressions, or text. This paper proposes a new method to learn a joint emotion representation for multimodal emotion recognition. Emotion-based feature for speech audio is learned by an unsupervised triplet-loss objective, and a text-to-text transformer network is used to extract text embedding for latent emotional meaning. Transfer learning provides a powerful and reusable technique to help fine-tune emotion recognition models trained on mega audio and text datasets respectively. The extracted emotional information from speech audio and text embedding are processed by dedicated transformer networks. The alternating co-attention mechanism is used to construct a deep transformer network. Multimodal fusion is implemented by a deep co-attention transformer network. Experimental results show the proposed method for learning a joint emotion representation achieves good performance in multimodal emotion recognition.
更多查看译文
关键词
Multimodal emotion recognition, Multimodal fusion, Transformer network
AI 理解论文
溯源树
样例
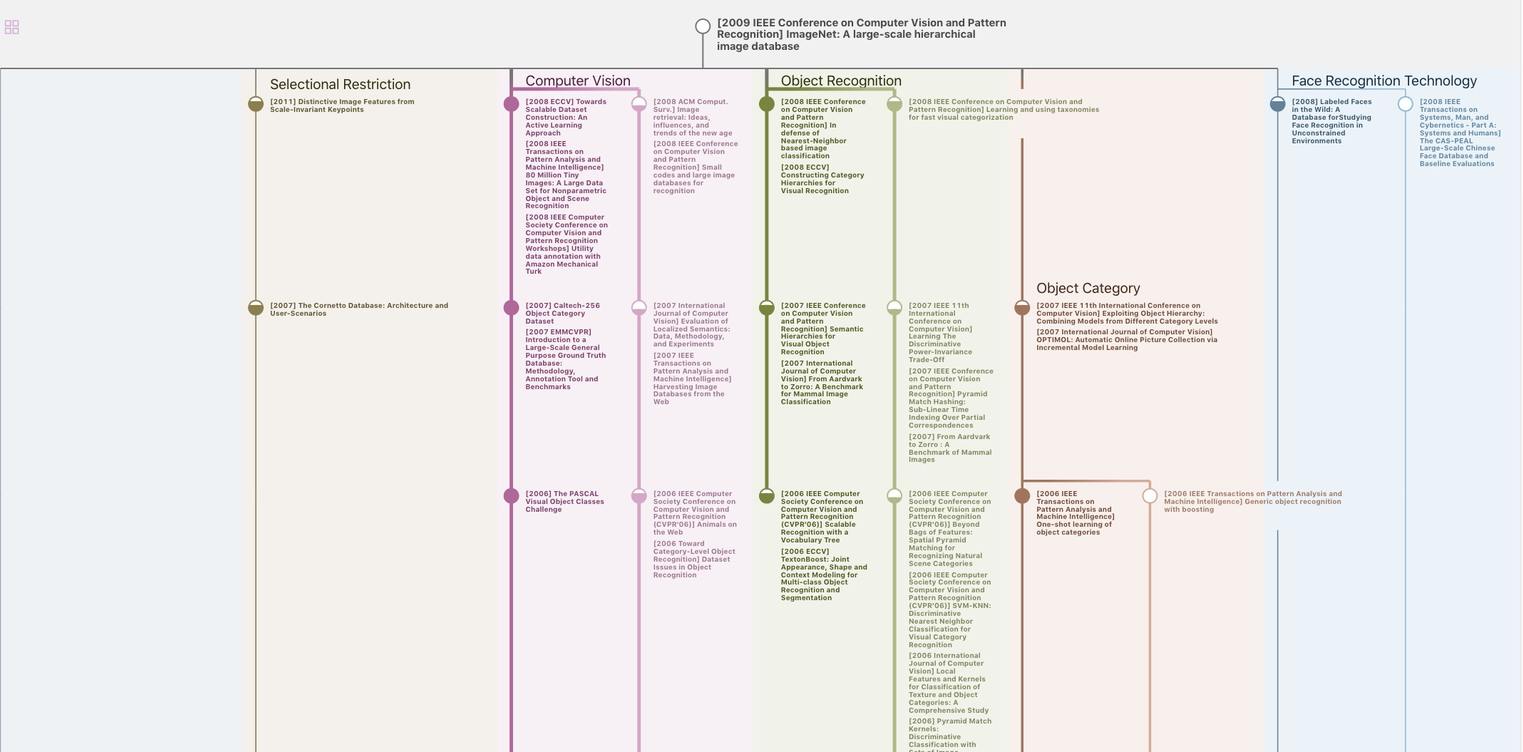
生成溯源树,研究论文发展脉络
Chat Paper
正在生成论文摘要