The Weak Supervision Approach for Question Answering over Text Using Triplets Recovering with QA-Based Rankers
16TH INTERNATIONAL CONFERENCE ON SOFT COMPUTING MODELS IN INDUSTRIAL AND ENVIRONMENTAL APPLICATIONS (SOCO 2021)(2022)
摘要
Nowadays, question answering systems have applications in many areas and deep learning models now show the best results, especially in the task of obtaining an answer based on a question and some text. To train such models, a large number of question-answer-text triplets (QAT) are required. We offer a weak-supervision approach that allows recovering QAT triplets for training from the question and answer pairs (QA) using text ranking instead of manual matching. Evaluation of the approach is performed on MS MARCO and SQuAD datasets for various parameters and models. The results show that this approach allows us to effectively recover QAT-triplets and train models: QAT-models got the drop in their quality no more than 10% while requiring only a small fraction of manually matched QAT-triplets.
更多查看译文
关键词
Information retrieval, Weak supervision, Question answering, QAT, QA, BERT, Extractive models, Generative models
AI 理解论文
溯源树
样例
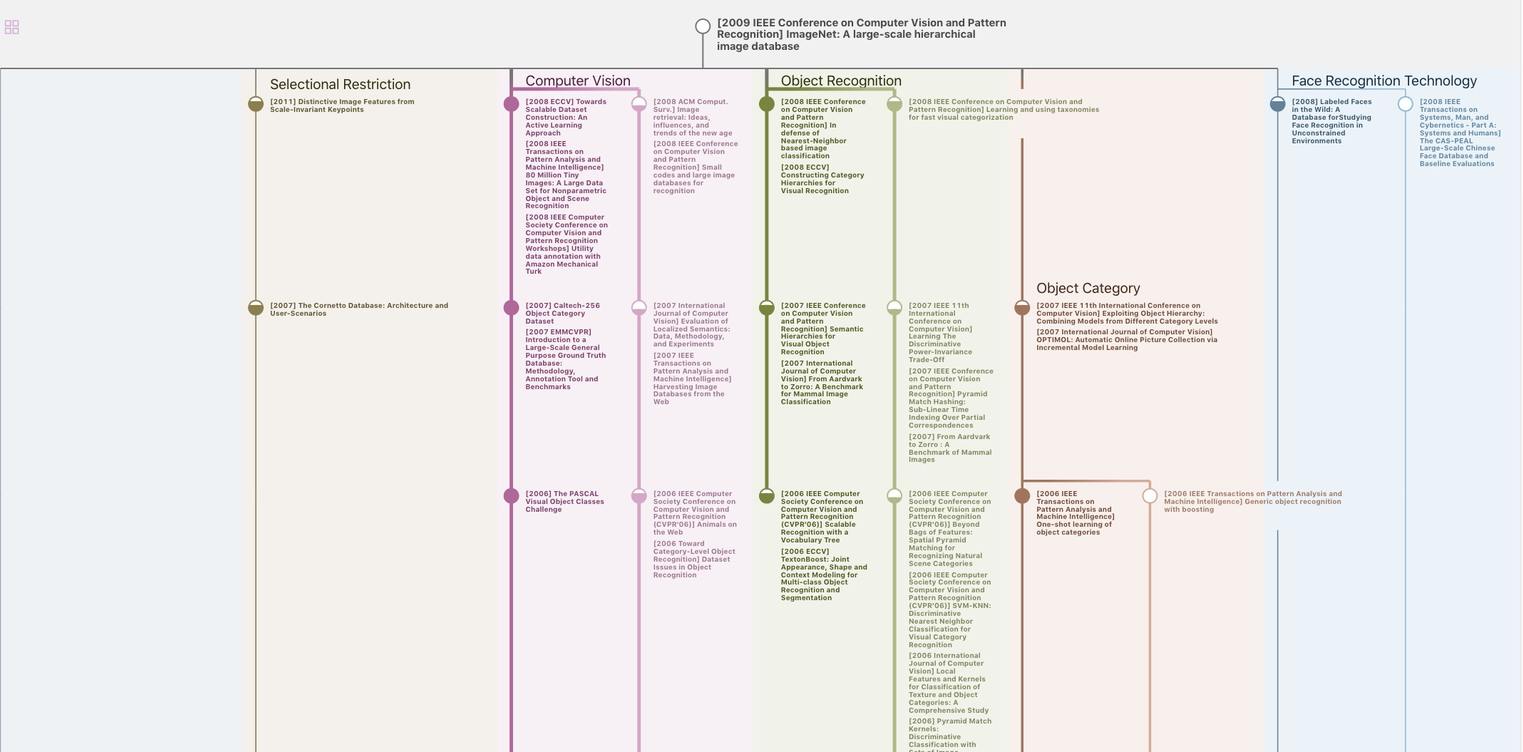
生成溯源树,研究论文发展脉络
Chat Paper
正在生成论文摘要