Graph Attention Feature Fusion Network For Als Point Cloud Classification
SENSORS(2021)
摘要
Classification is a fundamental task for airborne laser scanning (ALS) point cloud processing and applications. This task is challenging due to outdoor scenes with high complexity and point clouds with irregular distribution. Many existing methods based on deep learning techniques have drawbacks, such as complex pre/post-processing steps, an expensive sampling cost, and a limited receptive field size. In this paper, we propose a graph attention feature fusion network (GAFFNet) that can achieve a satisfactory classification performance by capturing wider contextual information of the ALS point cloud. Based on the graph attention mechanism, we first design a neighborhood feature fusion unit and an extended neighborhood feature fusion block, which effectively increases the receptive field for each point. On this basis, we further design a neural network based on encoder-decoder architecture to obtain the semantic features of point clouds at different levels, allowing us to achieve a more accurate classification. We evaluate the performance of our method on a publicly available ALS point cloud dataset provided by the International Society for Photogrammetry and Remote Sensing (ISPRS). The experimental results show that our method can effectively distinguish nine types of ground objects. We achieve more satisfactory results on different evaluation metrics when compared with the results obtained via other approaches.
更多查看译文
关键词
ALS point cloud, classification, deep learning, graph attention mechanism, receptive field
AI 理解论文
溯源树
样例
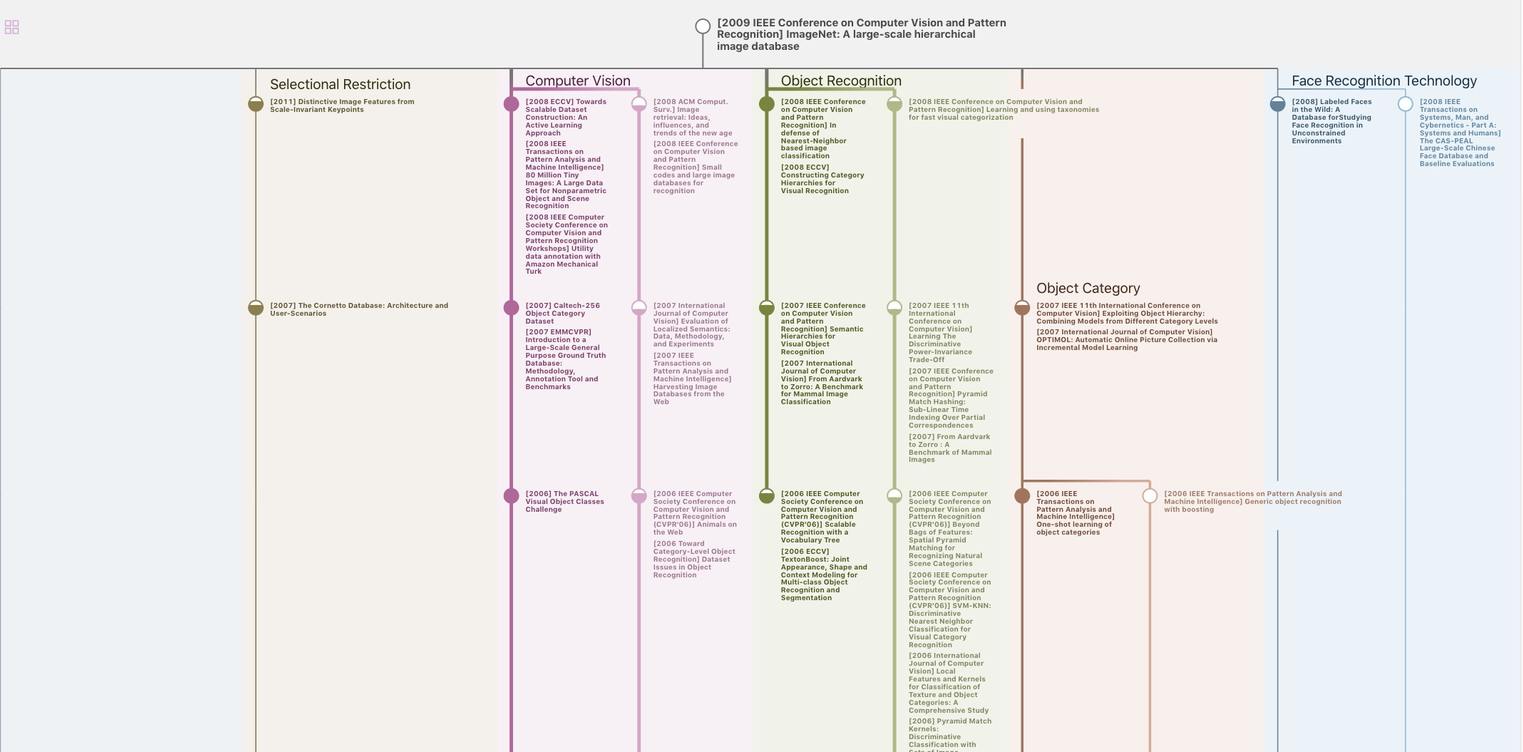
生成溯源树,研究论文发展脉络
Chat Paper
正在生成论文摘要