Using machine learning for predicting intensive care unit resource use during the COVID-19 pandemic in Denmark
SCIENTIFIC REPORTS(2021)
摘要
The COVID-19 pandemic has put massive strains on hospitals, and tools to guide hospital planners in resource allocation during the ebbs and flows of the pandemic are urgently needed. We investigate whether machine learning (ML) can be used for predictions of intensive care requirements a fixed number of days into the future. Retrospective design where health Records from 42,526 SARS-CoV-2 positive patients in Denmark was extracted. Random Forest (RF) models were trained to predict risk of ICU admission and use of mechanical ventilation after n days ( n = 1, 2, …, 15). An extended analysis was provided for n = 5 and n = 10. Models predicted n -day risk of ICU admission with an area under the receiver operator characteristic curve (ROC-AUC) between 0.981 and 0.995, and n -day risk of use of ventilation with an ROC-AUC between 0.982 and 0.997. The corresponding n -day forecasting models predicted the needed ICU capacity with a coefficient of determination (R 2 ) between 0.334 and 0.989 and use of ventilation with an R 2 between 0.446 and 0.973. The forecasting models performed worst, when forecasting many days into the future (for large n ). For n = 5, ICU capacity was predicted with ROC-AUC 0.990 and R 2 0.928, and use of ventilator was predicted with ROC-AUC 0.994 and R 2 0.854. Random Forest-based modelling can be used for accurate n -day forecasting predictions of ICU resource requirements, when n is not too large.
更多查看译文
关键词
Computational biology and bioinformatics,Virology,Science,Humanities and Social Sciences,multidisciplinary
AI 理解论文
溯源树
样例
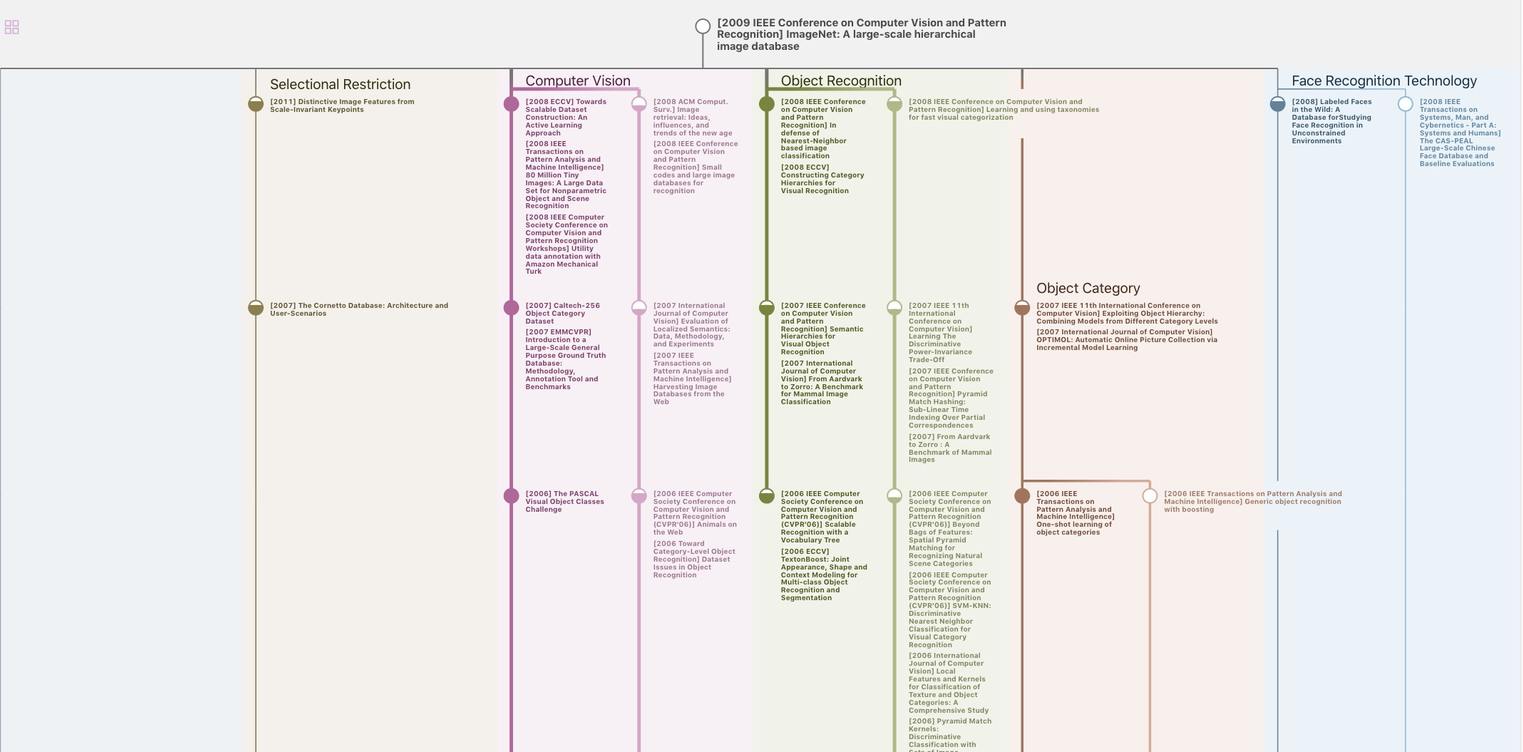
生成溯源树,研究论文发展脉络
Chat Paper
正在生成论文摘要