Experimental And Computational Investigation Of Enzyme Functional Annotations Uncovers Misannotation In The Ec 1.1.3.15 Enzyme Class
PLOS COMPUTATIONAL BIOLOGY(2021)
Abstract
Only a small fraction of genes deposited to databases have been experimentally characterised. The majority of proteins have their function assigned automatically, which can result in erroneous annotations. The reliability of current annotations in public databases is largely unknown; experimental attempts to validate the accuracy within individual enzyme classes are lacking. In this study we performed an overview of functional annotations to the BRENDA enzyme database. We first applied a high-throughput experimental platform to verify functional annotations to an enzyme class of S-2-hydroxyacid oxidases (EC 1.1.3.15). We chose 122 representative sequences of the class and screened them for their predicted function. Based on the experimental results, predicted domain architecture and similarity to previously characterised S-2-hydroxyacid oxidases, we inferred that at least 78% of sequences in the enzyme class are misannotated. We experimentally confirmed four alternative activities among the misannotated sequences and showed that misannotation in the enzyme class increased over time. Finally, we performed a computational analysis of annotations to all enzyme classes in the BRENDA database, and showed that nearly 18% of all sequences are annotated to an enzyme class while sharing no similarity or domain architecture to experimentally characterised representatives. We showed that even well-studied enzyme classes of industrial relevance are affected by the problem of functional misannotation.Author summaryCorrect annotation of genomes is crucial for our understanding and utilization of functional gene diversity, yet the reliability of current protein annotations in public databases is largely unknown. In our work we validated annotations to an S-2-hydroxyacid oxidase enzyme class (EC 1.1.3.15) by assessing activity of 122 representative sequences in a high-throughput screening experiment. From this dataset we inferred that at least 78% of the sequences in the enzyme class are misannotated, and confirmed four alternative activities among the misannotated sequences. We showed that the misannotation is widespread throughout enzyme classes, affecting even well-studied classes of industrial relevance. Overall, our study highlights the value of experimental and computational validation of predicted functions within individual enzyme classes.
MoreTranslated text
Key words
enzyme,functional annotations uncovers
AI Read Science
Must-Reading Tree
Example
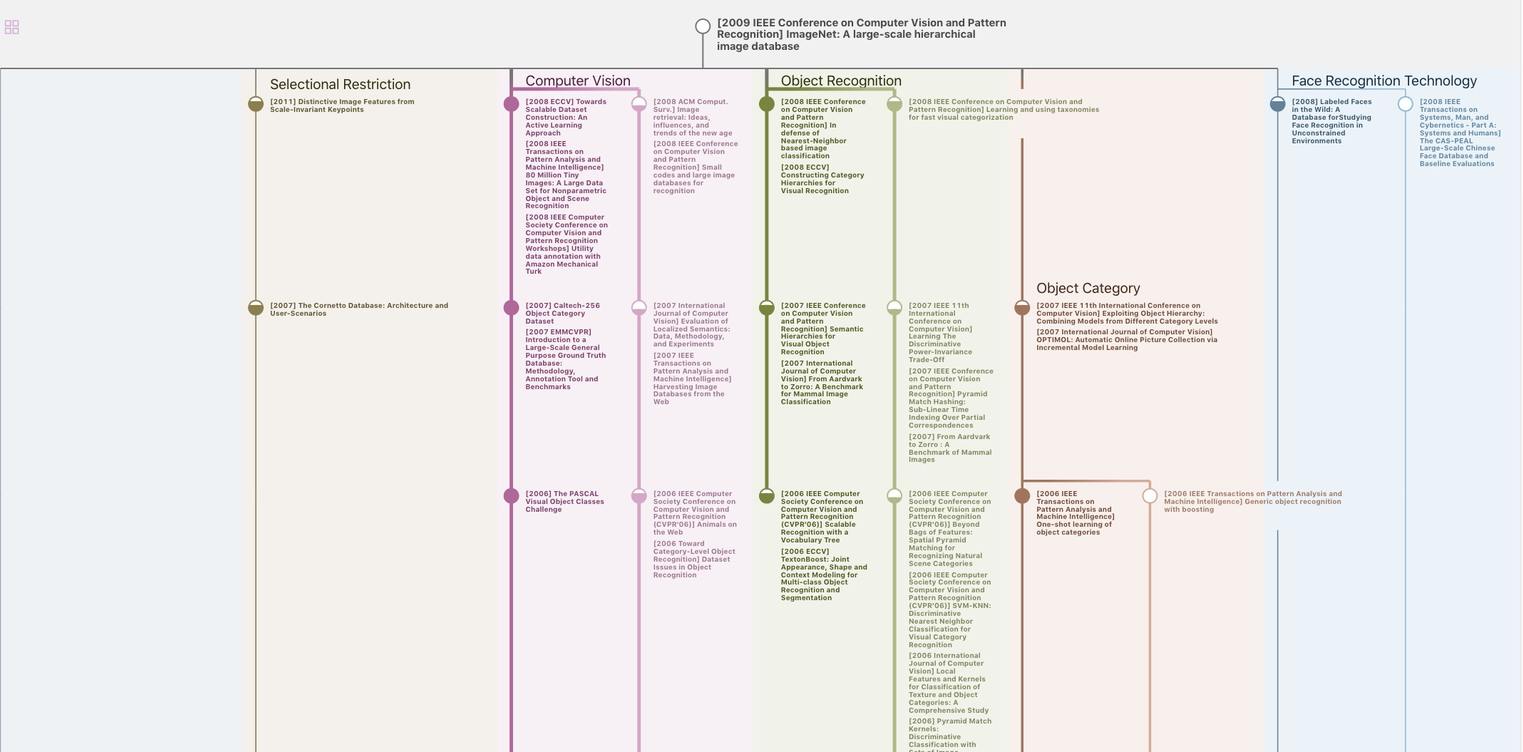
Generate MRT to find the research sequence of this paper
Chat Paper
Summary is being generated by the instructions you defined