Turning old models fashion again: Recycling classical CNN networks using the Lattice Transformation
arxiv(2021)
摘要
In the early 1990s, the first signs of life of the CNN era were given: LeCun et al. proposed a CNN model trained by the backpropagation algorithm to classify low-resolution images of handwritten digits. Undoubtedly, it was a breakthrough in the field of computer vision. But with the rise of other classification methods, it fell out fashion. That was until 2012, when Krizhevsky et al. revived the interest in CNNs by exhibiting considerably higher image classification accuracy on the ImageNet challenge. Since then, the complexity of the architectures are exponentially increasing and many structures are rapidly becoming obsolete. Using multistream networks as a base and the feature infusion precept, we explore the proposed LCNN cross-fusion strategy to use the backbones of former state-of-the-art networks on image classification in order to discover if the technique is able to put these designs back in the game. In this paper, we showed that we can obtain an increase of accuracy up to 63.21% on the NORB dataset we comparing with the original structure. However, no technique is definitive. While our goal is to try to reuse previous state-of-the-art architectures with few modifications, we also expose the disadvantages of our explored strategy.
更多查看译文
AI 理解论文
溯源树
样例
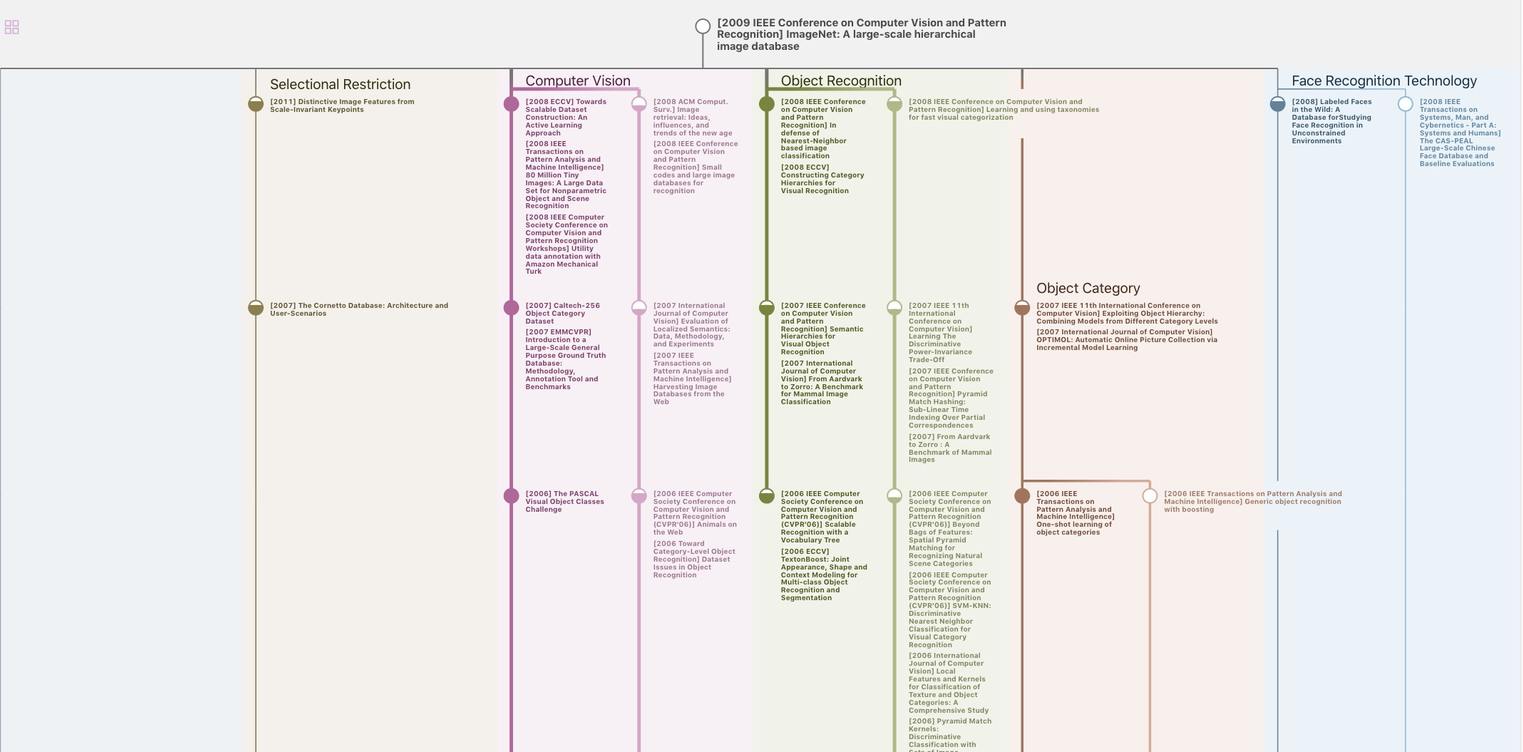
生成溯源树,研究论文发展脉络
Chat Paper
正在生成论文摘要