Diffusion-Based Voice Conversion with Fast Maximum Likelihood Sampling Scheme
International Conference on Learning Representations (ICLR)(2022)
摘要
Voice conversion is a common speech synthesis task which can be solved in different ways depending on a particular real-world scenario. The most challenging one often referred to as one-shot many-to-many voice conversion consists in copying the target voice from only one reference utterance in the most general case when both source and target speakers do not belong to the training dataset. We present a scalable high-quality solution based on diffusion probabilistic modeling and demonstrate its superior quality compared to state-of-the-art one-shot voice conversion approaches. Moreover, focusing on real-time applications, we investigate general principles which can make diffusion models faster while keeping synthesis quality at a high level. As a result, we develop a novel Stochastic Differential Equations solver suitable for various diffusion model types and generative tasks as shown through empirical studies and justify it by theoretical analysis.
更多查看译文
关键词
speech,voice conversion,diffusion models,stochastic differential equations
AI 理解论文
溯源树
样例
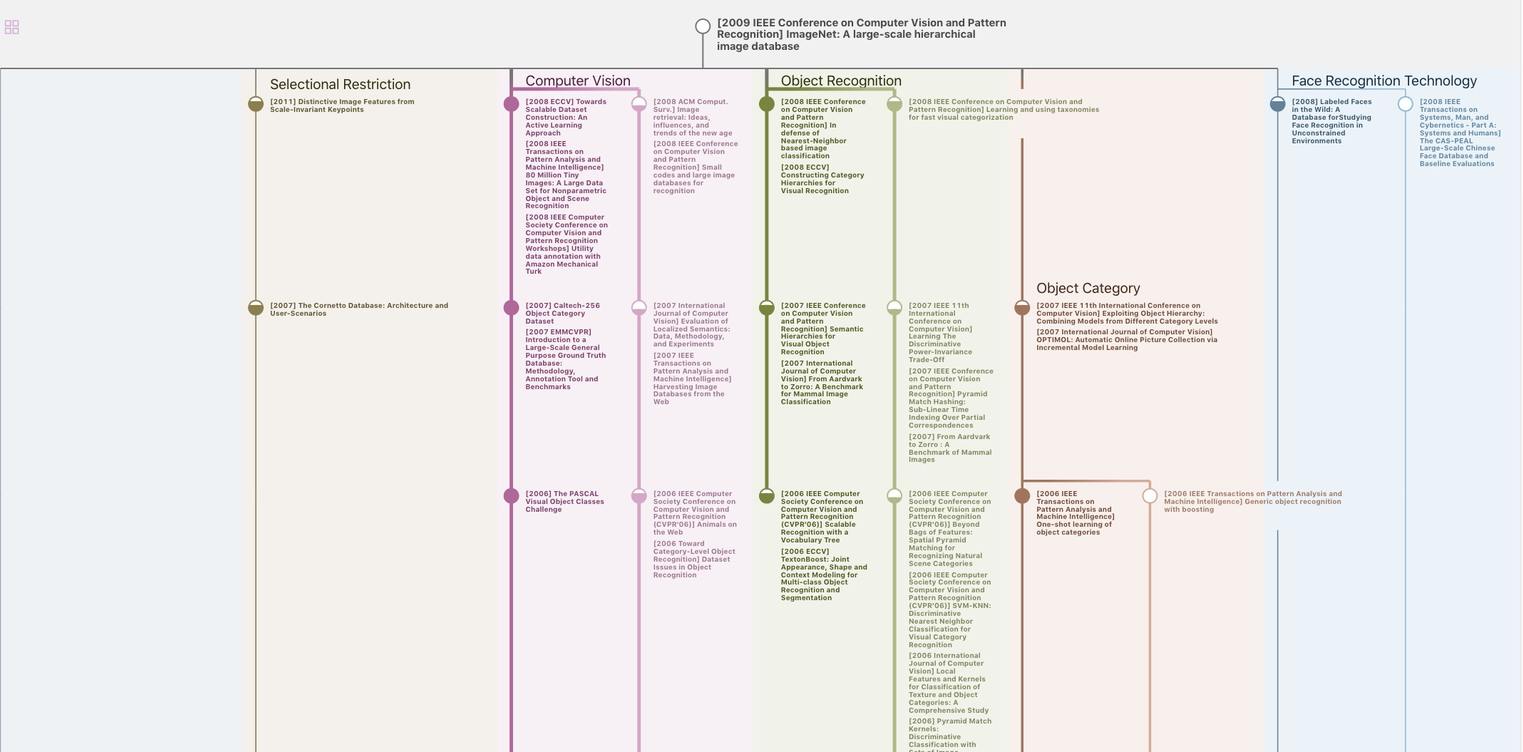
生成溯源树,研究论文发展脉络
Chat Paper
正在生成论文摘要