Stable training of autoencoders for hyperspectral unmixing
Image Analysis and Processing – ICIAP 2022(2021)
Abstract
Neural networks, autoencoders in particular, are one of the most promising solutions for unmixing hyperspectral data, i.e. reconstructing the spectra of observed substances (endmembers) and their relative mixing fractions (abundances). Unmixing is needed for effective hyperspectral analysis and classification. However, as we show in this paper, the training of autoencoders for unmixing is highly dependent on weights initialisation. Some sets of weights lead to degenerate or low performance solutions, introducing negative bias in expected performance. In this work we present the results of experiments investigating autoencoders' stability, verifying the dependence of reconstruction error on initial weights and exploring conditions needed for successful optimisation of autoencoder parameters.
MoreTranslated text
Key words
Autoencoders, Hyperspectral unmixing, Training stability, Network reinitialisation
AI Read Science
Must-Reading Tree
Example
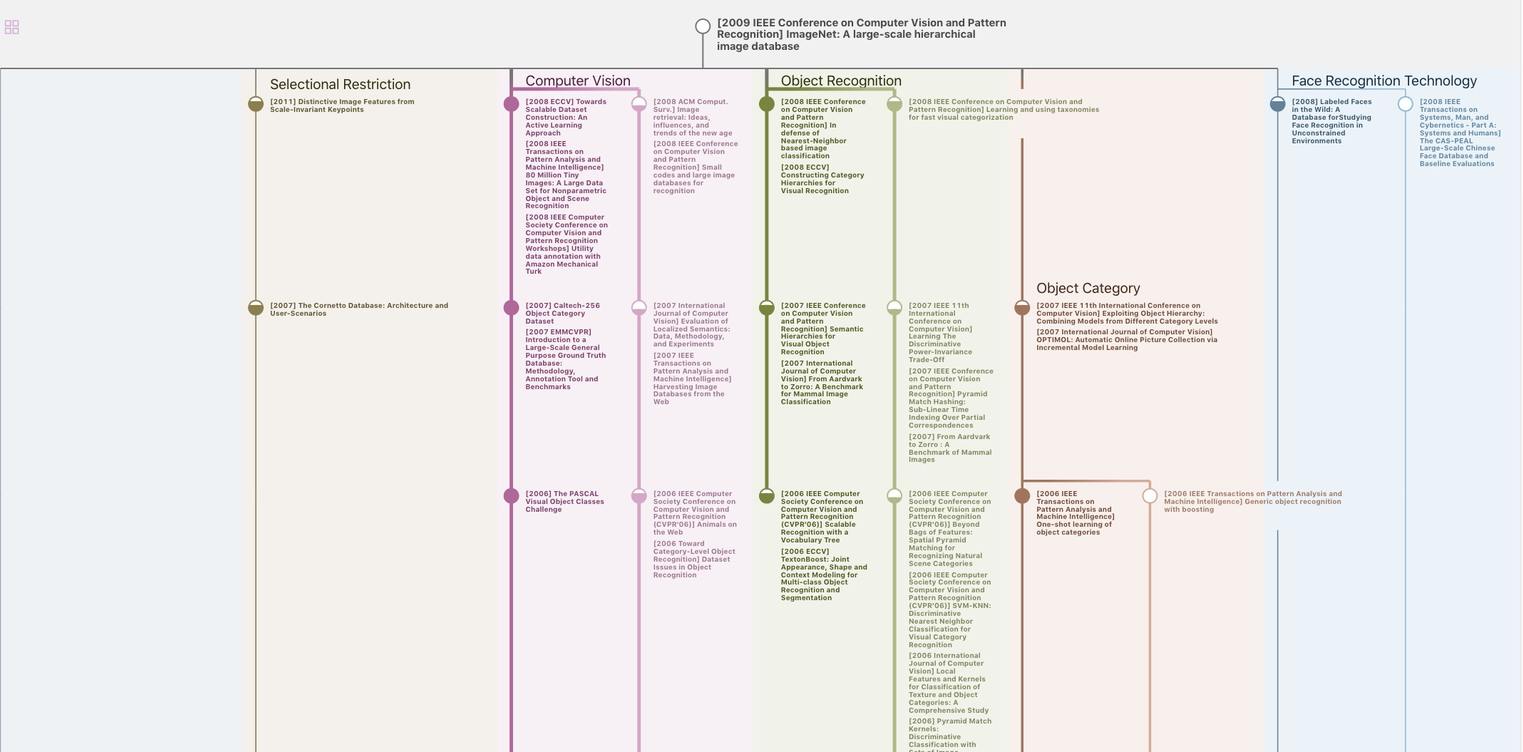
Generate MRT to find the research sequence of this paper
Chat Paper
Summary is being generated by the instructions you defined