Conditional cross-design synthesis estimators for generalizability in Medicaid
Biometrics(2023)
摘要
While much of the causal inference literature has focused on addressing internal validity biases, both internal and external validity are necessary for unbiased estimates in a target population of interest. However, few generalizability approaches exist for estimating causal quantities in a target population that is not well-represented by a randomized study but is reflected when additionally incorporating observational data. To generalize to a target population represented by a union of these data, we propose a novel class of conditional cross-design synthesis estimators that combine randomized and observational data, while addressing their estimates' respective biases-lack of overlap and unmeasured confounding. These methods enable estimating the causal effect of managed care plans on health care spending among Medicaid beneficiaries in New York City, which requires obtaining estimates for the 7% of beneficiaries randomized to a plan and 93% who choose a plan, who do not resemble randomized beneficiaries. Our new estimators include outcome regression, propensity weighting, and double robust approaches. All use the covariate overlap between the randomized and observational data to remove potential unmeasured confounding bias. Applying these methods, we find substantial heterogeneity in spending effects across managed care plans. This has major implications for our understanding of Medicaid, where this heterogeneity has previously been hidden. Additionally, we demonstrate that unmeasured confounding rather than lack of overlap poses a larger concern in this setting.
更多查看译文
关键词
causal inference,external validity,selection bias,transportability,unmeasured confounding
AI 理解论文
溯源树
样例
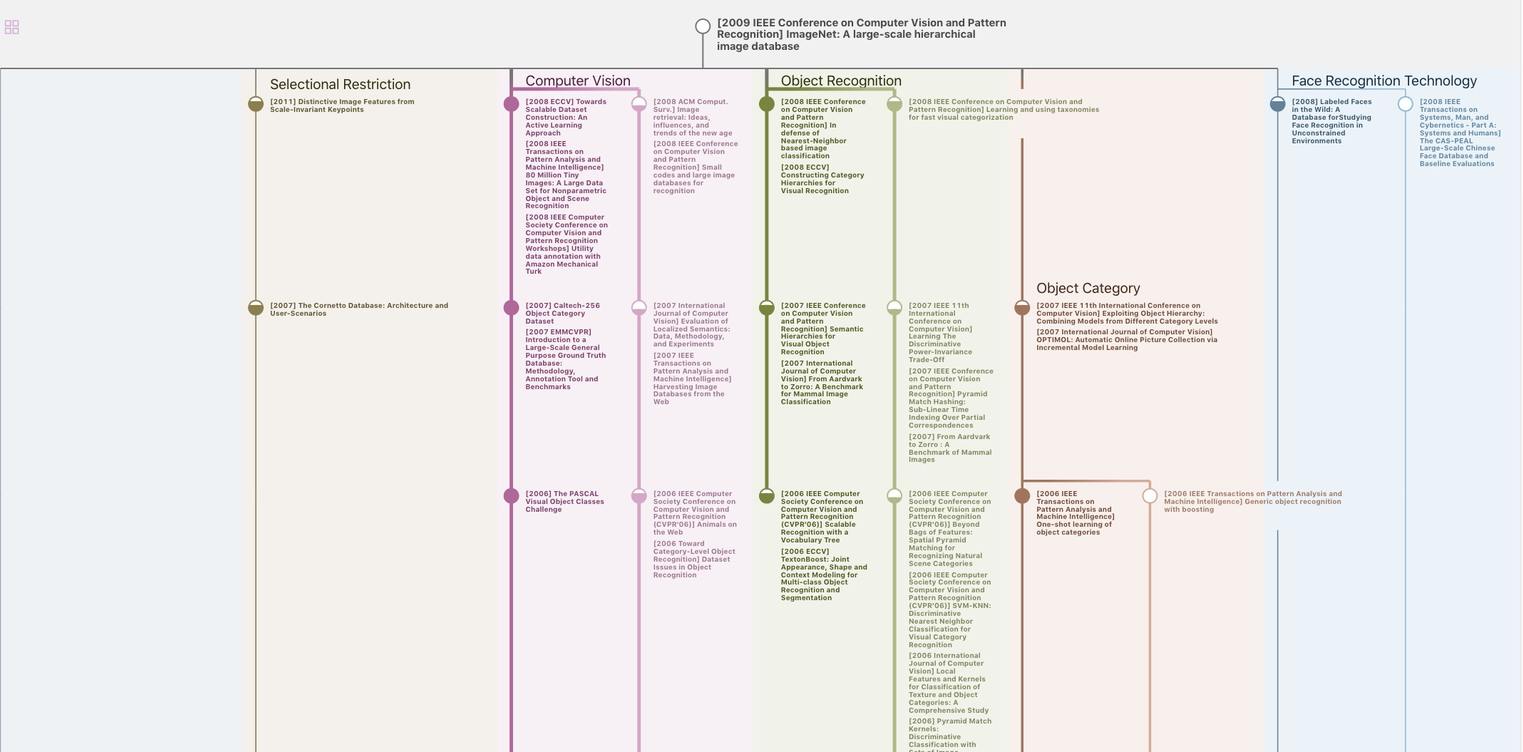
生成溯源树,研究论文发展脉络
Chat Paper
正在生成论文摘要