Deep Learning for Multifidelity Aerodynamic Distribution Modeling from Experimental and Simulation Data
AIAA JOURNAL(2022)
Abstract
Wind-tunnel experiment plays a critical role in the design and development phases of modern aircraft, which is always limited by prohibitive cost. In contrast, numerical simulation, as an important alternative research paradigm, mimics complex flow behaviors but is less accurate compared to experiments. This leads to the recent development and emerging interest in applying data fusion for aerodynamic prediction. In particular, the accurate prediction of aerodynamics with lower computational cost can be achieved by fusing experimental (high-fidelity) and computational (low-fidelity) aerodynamic data. Currently, existing works on aerodynamic models using data fusion mainly concern integrated data, like lift, drag, etc. In this paper, a multifidelity aerodynamic model based on deep neural network (DNN) is developed for aerodynamic distribution over the wing surface, where both numerical and experimental data are introduced in the loss function with a proper weighting factor to balance the overall accuracy. The proposed approach is illustrated by modeling the surface pressure distribution of the ONERA M6 wing in transonic flow, including different scenarios where the flow condition varies or a small number of high-fidelity data are available. The results demonstrate that the proposed model trained based on a decent number of low-fidelity data and a few high-fidelity data can accurately predict the surface pressure distribution on the transonic wing. The outperformance of the proposed model over other DNNs with a single fidelity has been reported.
MoreTranslated text
AI Read Science
Must-Reading Tree
Example
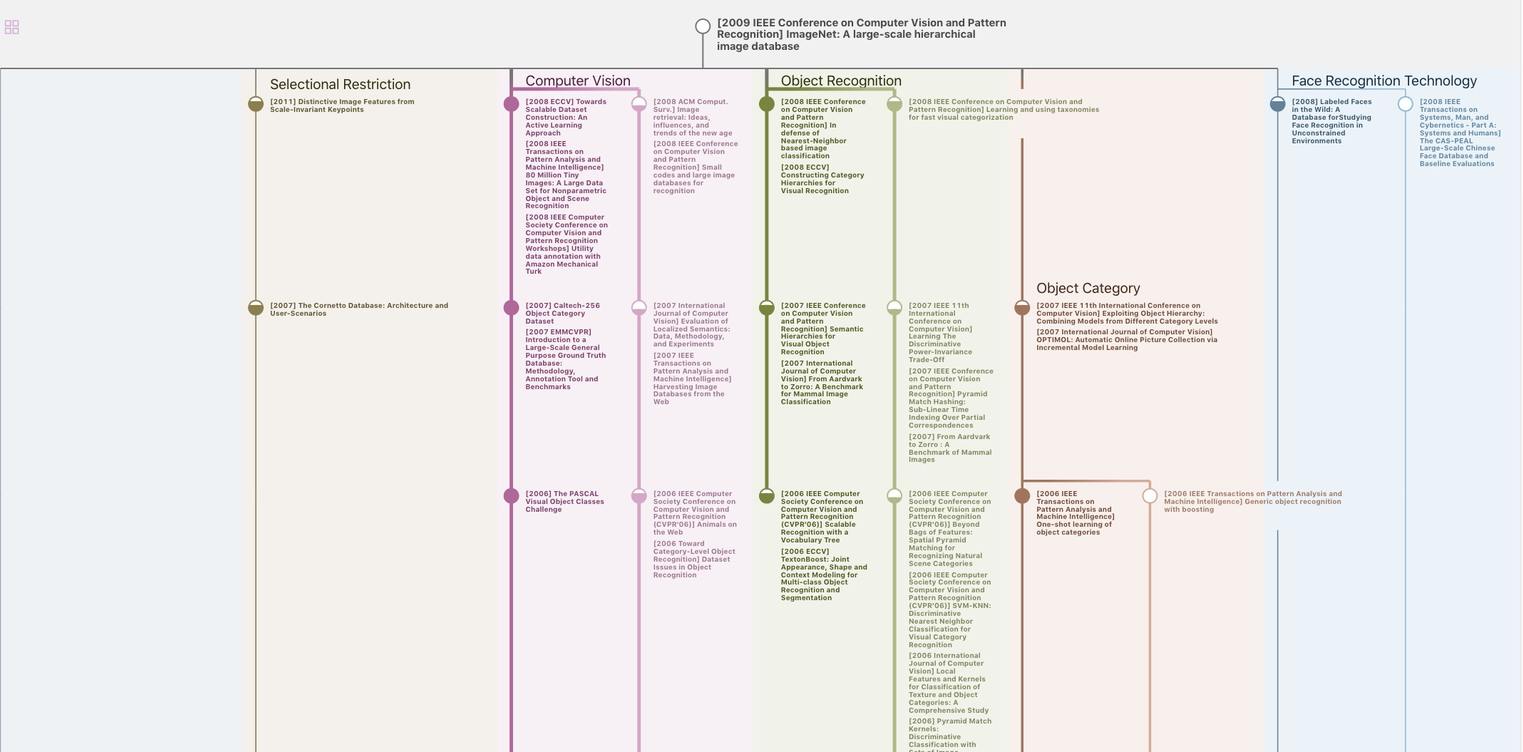
Generate MRT to find the research sequence of this paper
Chat Paper
Summary is being generated by the instructions you defined