Nesterov Accelerated ADMM for Fast Diffeomorphic Image Registration
MEDICAL IMAGE COMPUTING AND COMPUTER ASSISTED INTERVENTION - MICCAI 2021, PT IV(2021)
摘要
Deterministic approaches using iterative optimisation have been historically successful in diffeomorphic image registration (DiffIR). Although these approaches are highly accurate, they typically carry a significant computational burden. Recent developments in stochastic approaches based on deep learning have achieved sub-second runtimes for DiffIR with competitive registration accuracy, offering a fast alternative to conventional iterative methods. In this paper, we attempt to reduce this difference in speed whilst retaining the performance advantage of iterative approaches in DiffIR. We first propose a simple iterative scheme that functionally composes intermediate non-stationary velocity fields to handle large deformations in images whilst guaranteeing diffeomorphisms in the resultant deformation. We then propose a convex optimisation model that uses a regularisation term of arbitrary order to impose smoothness on these velocity fields and solve this model with a fast algorithm that combines Nesterov gradient descent and the alternating direction method of multipliers (ADMM). Finally, we leverage the computational power of GPU to implement this accelerated ADMM solver on a 3D cardiac MRI dataset, further reducing runtime to less than 2 s. In addition to producing strictly diffeomorphic deformations, our methods outperform both state-of-the-art deep learning-based and iterative DiffIR approaches in terms of dice and Hausdorff scores, with speed approaching the inference time of deep learning-based methods.
更多查看译文
关键词
Image registration, Diffeomorphism, ADMM
AI 理解论文
溯源树
样例
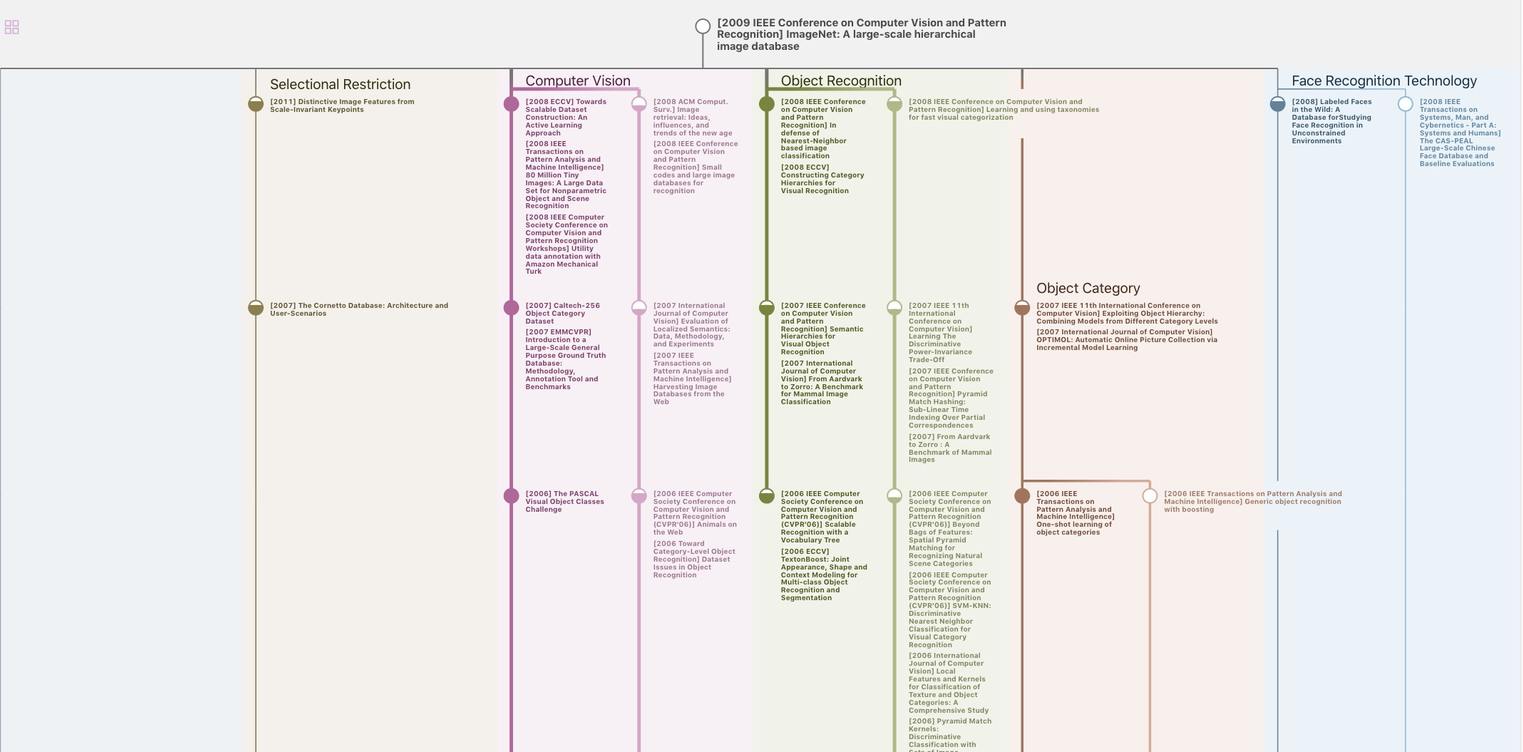
生成溯源树,研究论文发展脉络
Chat Paper
正在生成论文摘要