Joint magnitude estimation and phase recovery using cycle-in-cycle gan for non-parallel speech enhancement
IEEE International Conference on Acoustics, Speech, and Signal Processing (ICASSP)(2022)
Abstract
For the lack of adequate paired noisy-clean speech corpus in many real scenarios, non-parallel training is a promising task for DNN-based speech enhancement methods. However, because of the severe mismatch between input and target speeches, many previous studies only focus on the magnitude spectrum estimation and remain the phase unaltered, resulting in the degraded speech quality under low signal-to-noise ratio conditions. To tackle this problem, we decouple the difficult target w.r.t. original spectrum optimization into spectral magnitude and phase, and a novel Cycle-in-Cycle generative adversarial network (dubbed CinCGAN) is proposed to jointly estimate the spectral magnitude and phase information stage by stage under unpaired data. In the first stage, we pretrain a magnitude CycleGAN to coarsely estimate the spectral magnitude of clean speech. In the second stage, we incorporate the pretrained CycleGAN with a complex-valued CycleGAN as a cycle-in-cycle structure to simultaneously recover phase information and refine the overall spectrum. Experimental results demonstrate that the proposed approach significantly outperforms previous baselines under non-parallel training. The evaluation on training the models with standard paired data also shows that CinCGAN achieves remarkable performance especially in reducing background noise and speech distortion.
MoreTranslated text
Key words
speech enhancement,non-parallel,Cycle-in-Cycle GAN,magnitude spectrum estimation,phase recovery
AI Read Science
Must-Reading Tree
Example
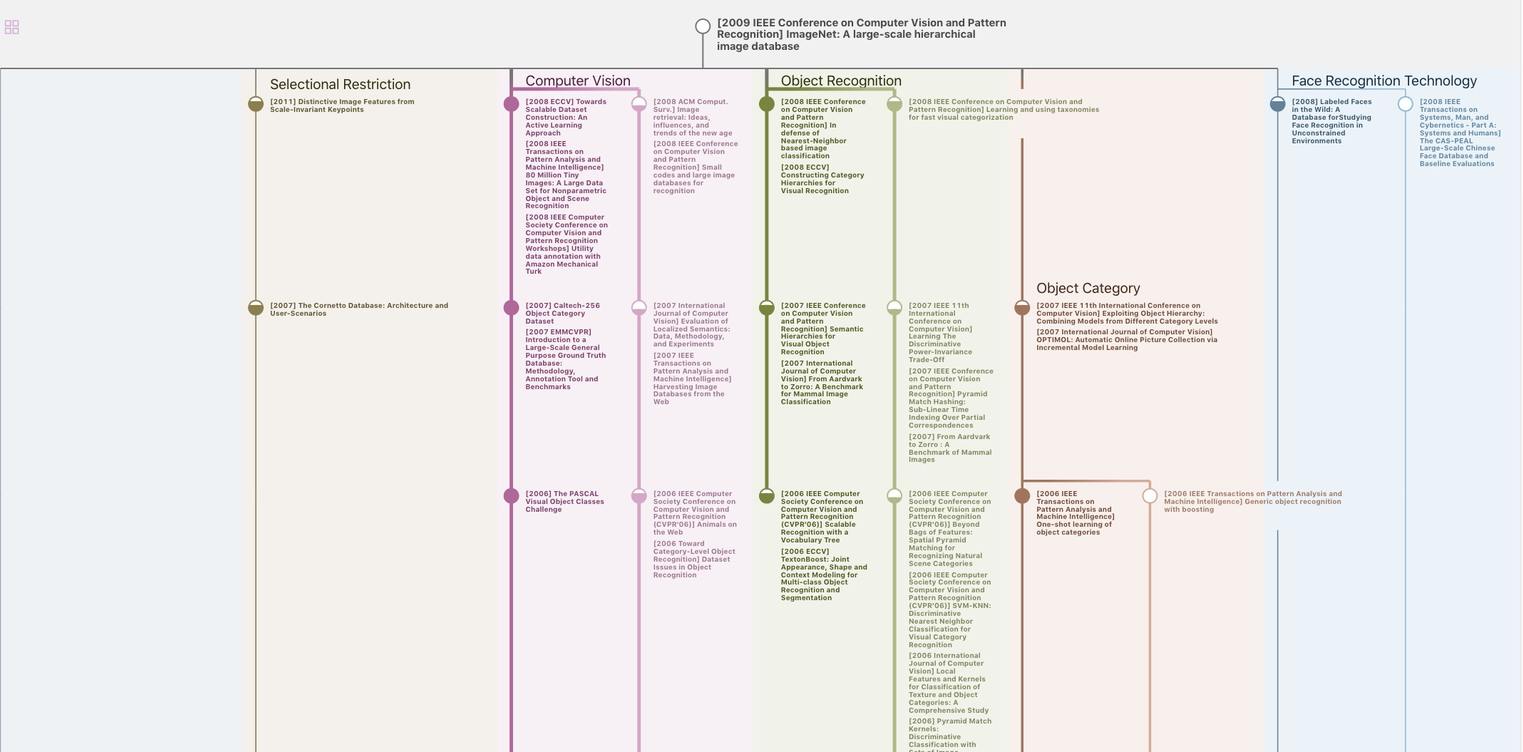
Generate MRT to find the research sequence of this paper
Chat Paper
Summary is being generated by the instructions you defined