Non-Euclidean Self-Organizing Maps
arxiv(2021)
摘要
Self-Organizing Maps (SOMs, Kohonen networks) belong to neural network models of the unsupervised class. In this paper, we present the generalized setup for non-Euclidean SOMs. Most data analysts take it for granted to use some subregions of a flat space as their data model; however, by the assumption that the underlying geometry is non-Euclidean we obtain a new degree of freedom for the techniques that translate the similarities into spatial neighborhood relationships. We improve the traditional SOM algorithm by introducing topology-related extensions. Our proposition can be successfully applied to dimension reduction, clustering or finding similarities in big data (both hierarchical and non-hierarchical).
更多查看译文
关键词
maps,non-euclidean,self-organizing
AI 理解论文
溯源树
样例
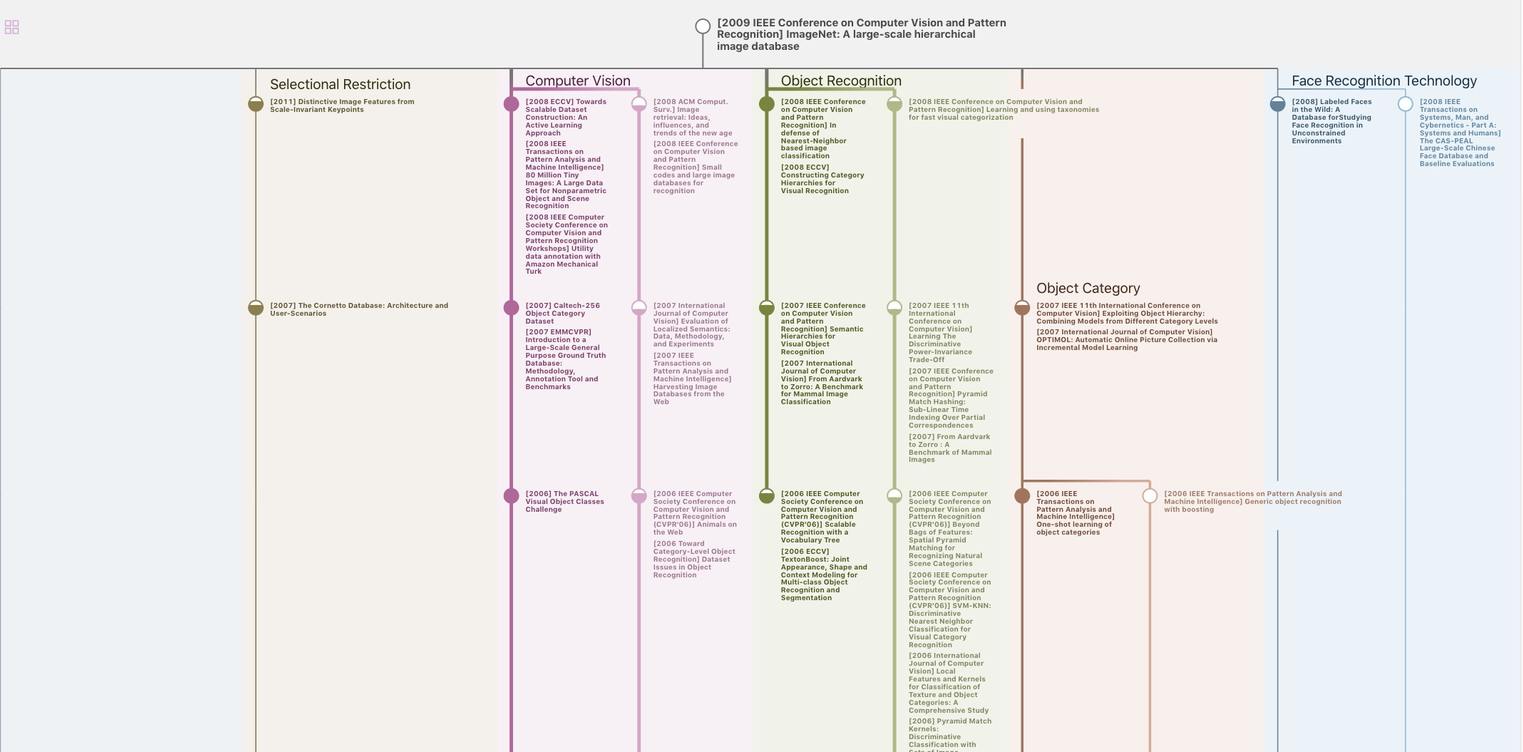
生成溯源树,研究论文发展脉络
Chat Paper
正在生成论文摘要