Segmentation of Static and Dynamic Atomic-Resolution Microscopy Data Sets with Unsupervised Machine Learning Using Local Symmetry Descriptors
MICROSCOPY AND MICROANALYSIS(2021)
摘要
We present an unsupervised machine learning approach for segmentation of static and dynamic atomic-resolution microscopy data sets in the form of images and video sequences. In our approach, we first extract local features via symmetry operations. Subsequent dimension reduction and clustering analysis are performed in feature space to assign pattern labels to each pixel. Furthermore, we propose the stride and upsampling scheme as well as separability analysis to speed up the segmentation process of image sequences. We apply our approach to static atomic-resolution scanning transmission electron microscopy images and video sequences. Our code is released as a python module that can be used as a standalone program or as a plugin to other microscopy packages.
更多查看译文
关键词
HAADF-STEM, segmentation, symmetry descriptors, unsupervised learning
AI 理解论文
溯源树
样例
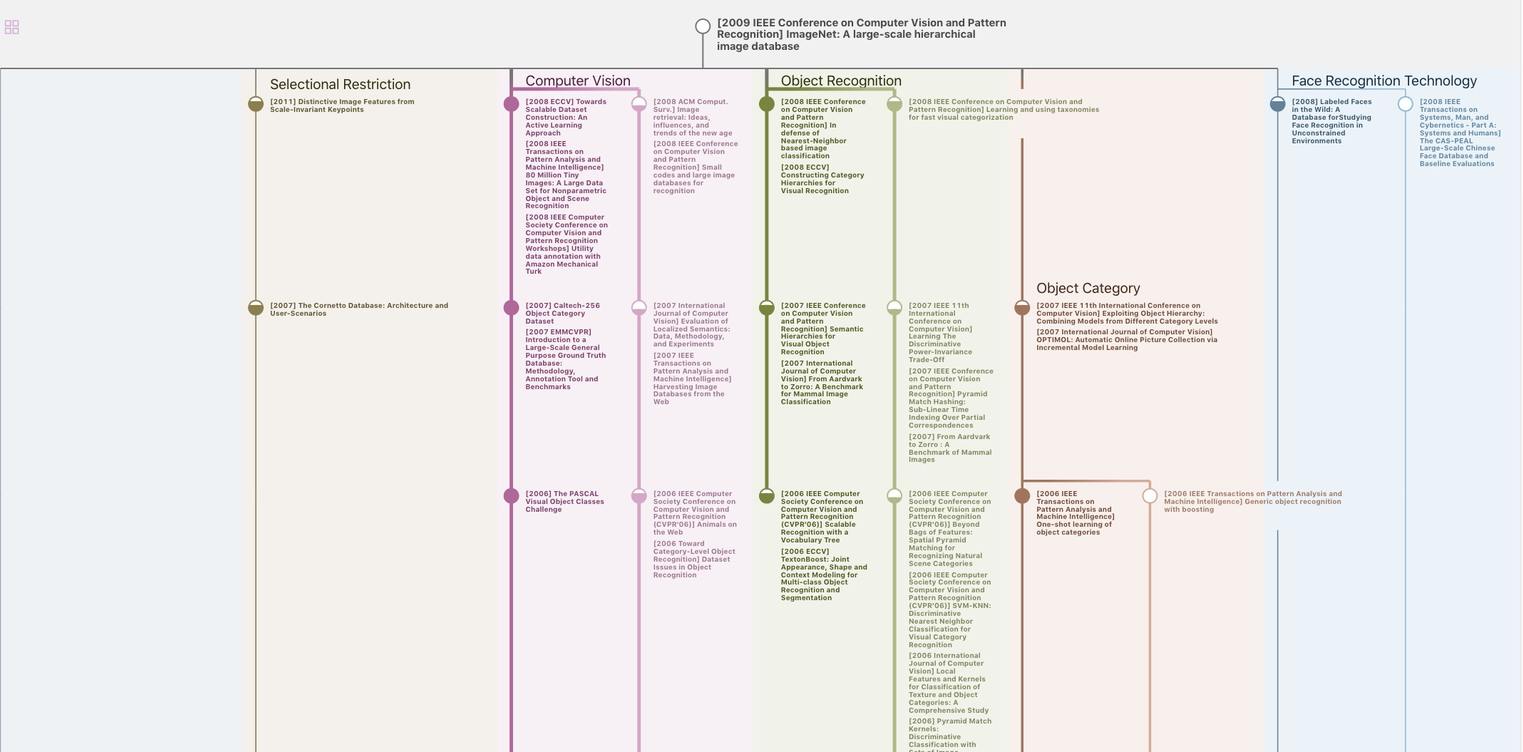
生成溯源树,研究论文发展脉络
Chat Paper
正在生成论文摘要