Neural forecasting at scale
arxiv(2021)
摘要
We study the problem of efficiently scaling ensemble-based deep neural networks for time series (TS) forecasting on a large set of time series. Current state-of-the-art deep ensemble models have high memory and computational requirements, hampering their use to forecast millions of TS in practical scenarios. We propose N-BEATS(P), a global multivariate variant of the N-BEATS model designed to allow simultaneous training of multiple univariate TS forecasting models. Our model addresses the practical limitations of related models, reducing the training time by half and memory requirement by a factor of 5, while keeping the same level of accuracy. We have performed multiple experiments detailing the various ways to train our model and have obtained results that demonstrate its capacity to support zero-shot TS forecasting, i.e., to train a neural network on a source TS dataset and deploy it on a different target TS dataset without retraining, which provides an efficient and reliable solution to forecast at scale even in difficult forecasting conditions.
更多查看译文
关键词
neural forecasting,scale
AI 理解论文
溯源树
样例
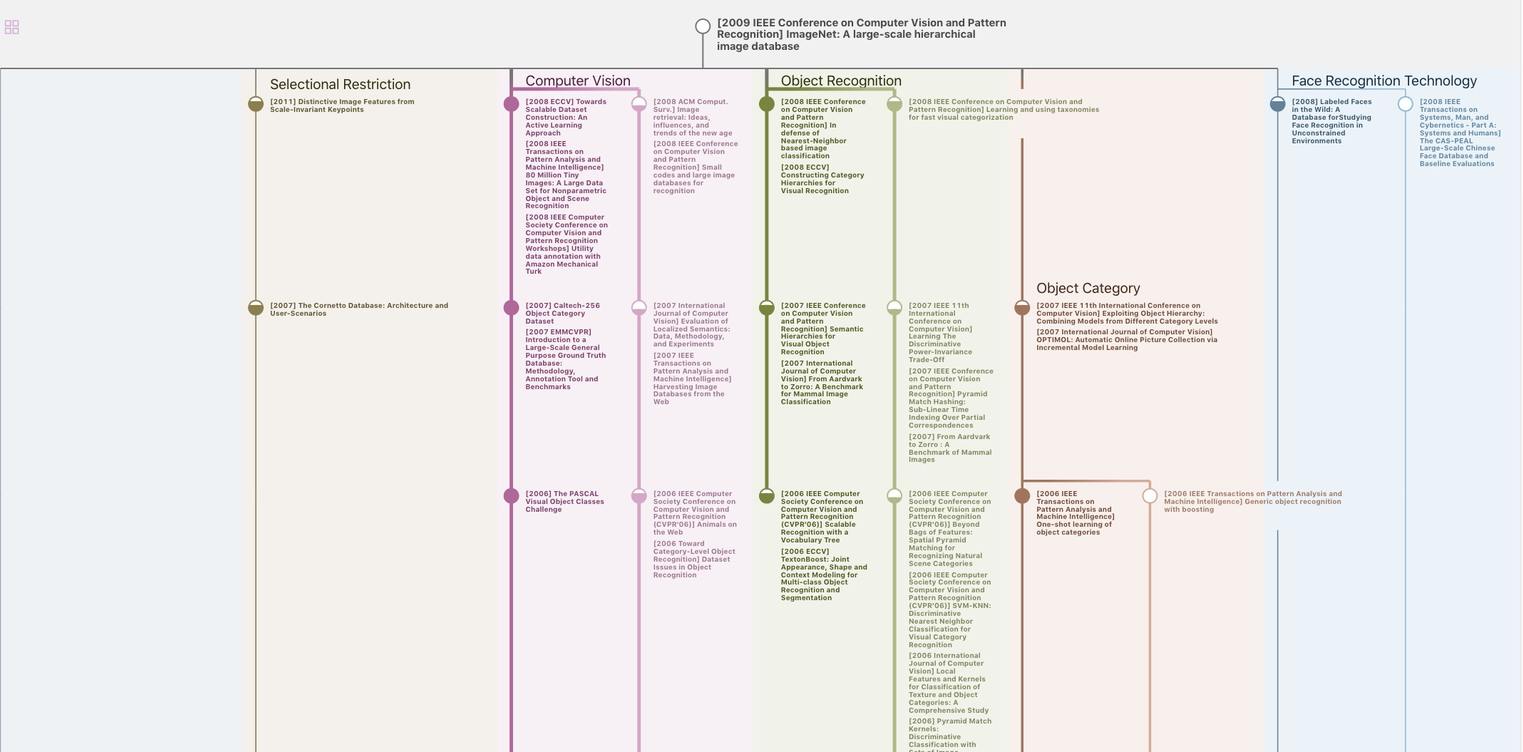
生成溯源树,研究论文发展脉络
Chat Paper
正在生成论文摘要