Scaling Enterprise Recommender Systems for Decentralization
RECSYS(2021)
Abstract
ABSTRACT Within decentralized organizations, the local demand for recommender systems to support business processes grows. The diversity in data sources and infrastructure challenges central engineering teams. Achieving a high delivery velocity without technical debt requires a scalable approach in the development and operations of recommender systems. At the HEINEKEN Company, we execute a machine learning operations method with five best practices: pipeline automation, data availability, exchangeable artifacts, observability, and policy-based security. Creating a culture of self-service, automation, and collaboration to scale recommender systems for decentralization. We demonstrate a practical use case of a self-service ML workspace deployment and a recommender system, that scale faster to subsidiaries and with less technical debt. This enables HEINEKEN to globally support applications that generate insights with local business impact.
MoreTranslated text
Key words
automation, collaboration, software engineering, mlops, self-service
AI Read Science
Must-Reading Tree
Example
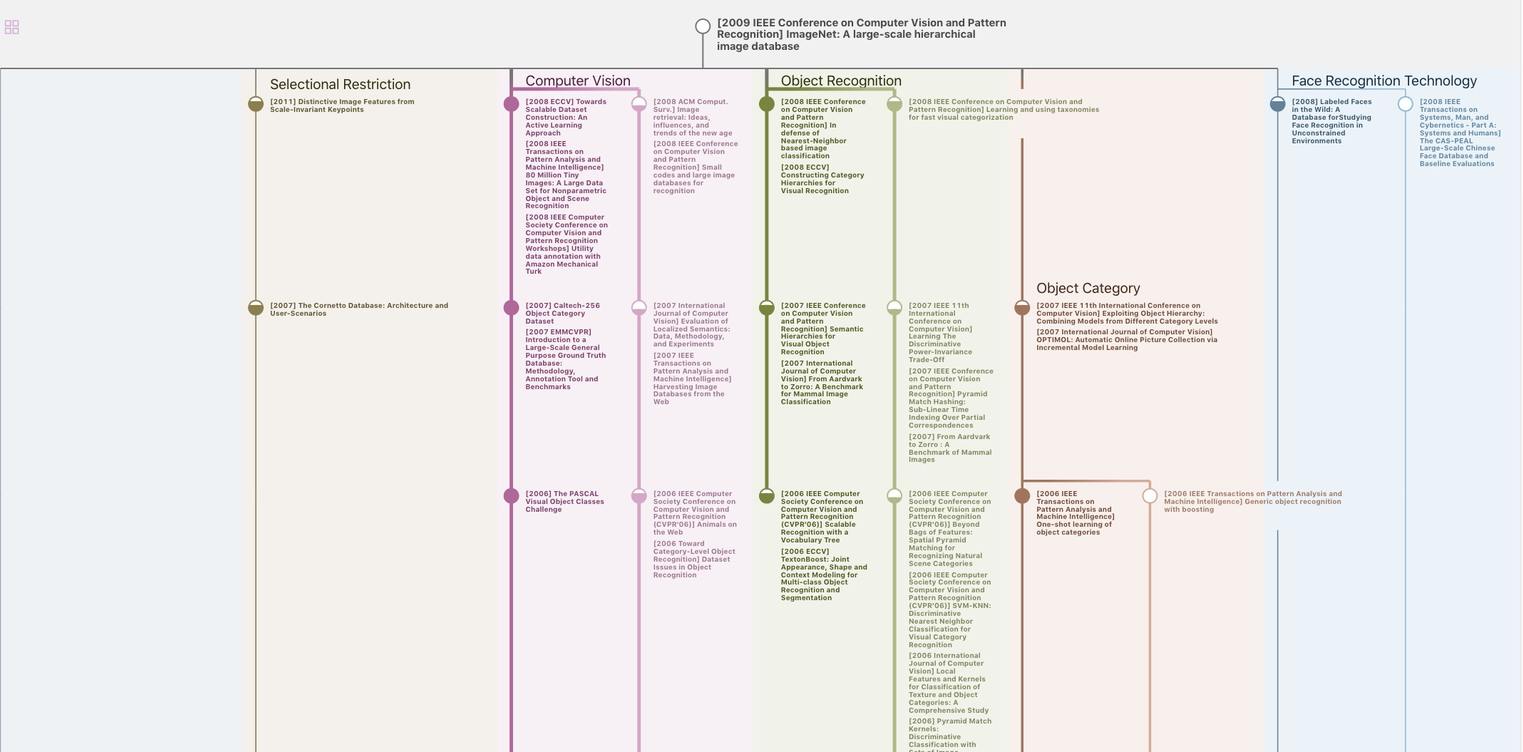
Generate MRT to find the research sequence of this paper
Chat Paper
Summary is being generated by the instructions you defined