Hierarchical Policy for Non-prehensile Multi-object Rearrangement with Deep Reinforcement Learning and Monte Carlo Tree Search
CoRR(2021)
Abstract
Non-prehensile multi-object rearrangement is a robotic task of planning feasible paths and transferring multiple objects to their predefined target poses without grasping. It needs to consider how each object reaches the target and the order of object movement, which significantly deepens the complexity of the problem. To address these challenges, we propose a hierarchical policy to divide and conquer for non-prehensile multi-object rearrangement. In the high-level policy, guided by a designed policy network, the Monte Carlo Tree Search efficiently searches for the optimal rearrangement sequence among multiple objects, which benefits from imitation and reinforcement. In the low-level policy, the robot plans the paths according to the order of path primitives and manipulates the objects to approach the goal poses one by one. We verify through experiments that the proposed method can achieve a higher success rate, fewer steps, and shorter path length compared with the state-of-the-art.
MoreTranslated text
Key words
hierarchical policy,deep reinforcement learning,non-prehensile,multi-object
AI Read Science
Must-Reading Tree
Example
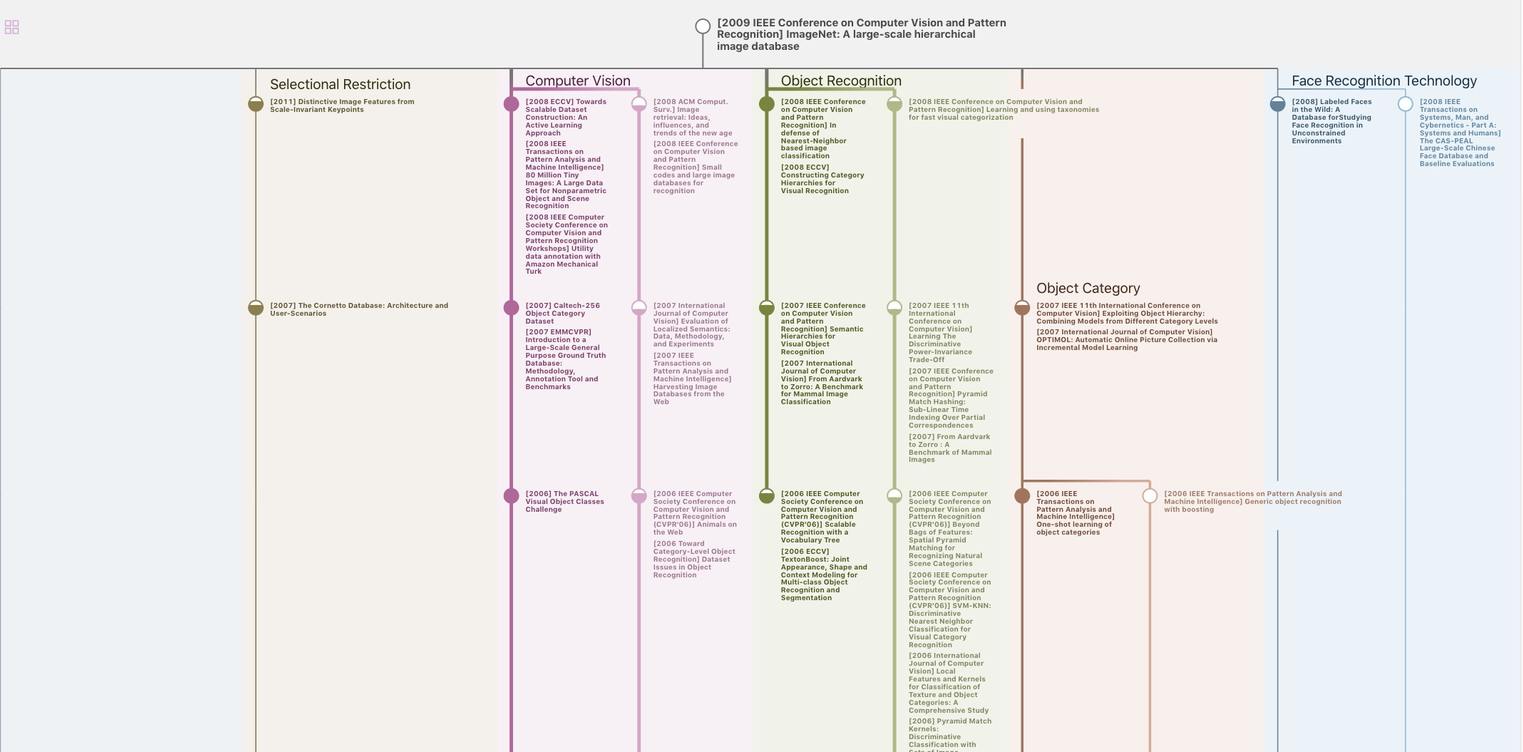
Generate MRT to find the research sequence of this paper
Chat Paper
Summary is being generated by the instructions you defined