KNN Learning Techniques for Proportional Myocontrol in Prosthetics
arxiv(2021)
摘要
This work has been conducted in the context of pattern-recognition-based control for electromyographic prostheses. It presents a k-nearest neighbour (kNN) classification technique for gesture recognition, extended by a proportionality scheme. The methods proposed are practically implemented and validated. Datasets are captured by means of a state-of-the-art 8-channel electromyography (EMG) armband positioned on the forearm. Based on this data, the influence of kNN's parameters is analyzed in pilot experiments. Moreover, the effect of proportionality scaling and rest thresholding schemes is investigated. A randomized, double-blind user study is conducted to compare the implemented method with the state-of-research algorithm Ridge Regression with Random Fourier Features (RR-RFF) for different levels of gesture exertion. The results from these experiments show a statistically significant improvement in favour of the kNN-based algorithm.
更多查看译文
关键词
proportional myocontrol
AI 理解论文
溯源树
样例
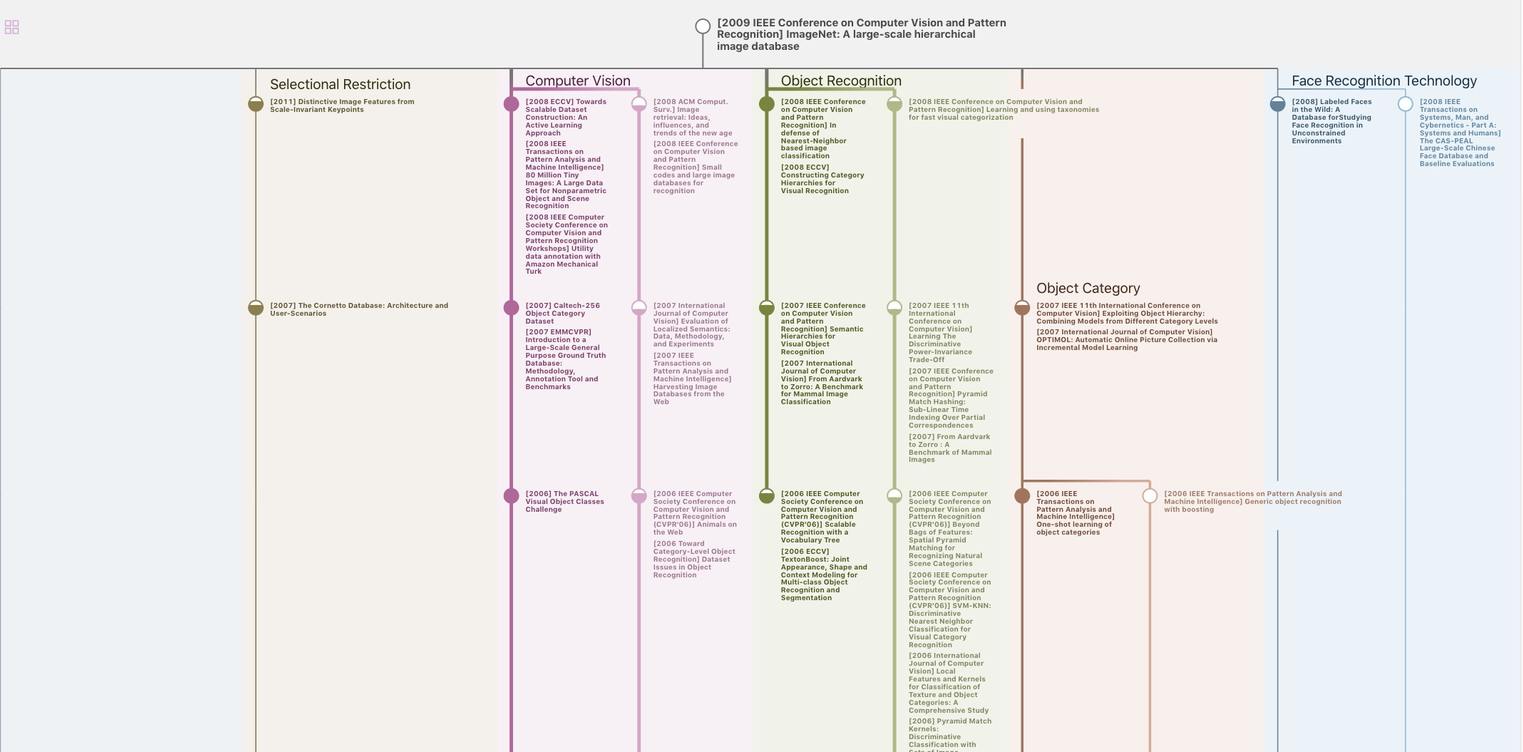
生成溯源树,研究论文发展脉络
Chat Paper
正在生成论文摘要