Analyzing the Habitable Zones of Circumbinary Planets Using Machine Learning
ASTROPHYSICAL JOURNAL(2022)
摘要
Exoplanet detection in the past decade by efforts including NASA's Kepler and TESS missions has revealed many worlds that differ substantially from planets in our own solar system, including more than 150 exoplanets orbiting binary or multi-star systems. This not only broadens our understanding of the diversity of exoplanets, but also promotes our study of exoplanets in the complex binary systems and provides motivation to explore their habitability. In this study, we investigate the habitable zones of circumbinary planets (P-type) based on planetary trajectory and dynamically informed habitable zones. Our results indicate that the mass ratio and orbital eccentricity of binary stars are important factors affecting the orbital stability and habitability of planetary systems. Moreover, planetary trajectory and dynamically informed habitable zones divide planetary habitability into three categories: habitable, periodic habitable, and non-habitable. Therefore, we successfully train a machine-learning model to quickly and efficiently classify these planetary systems, which provides more useful constraints.
更多查看译文
关键词
circumbinary planets,habitable zones,machine learning
AI 理解论文
溯源树
样例
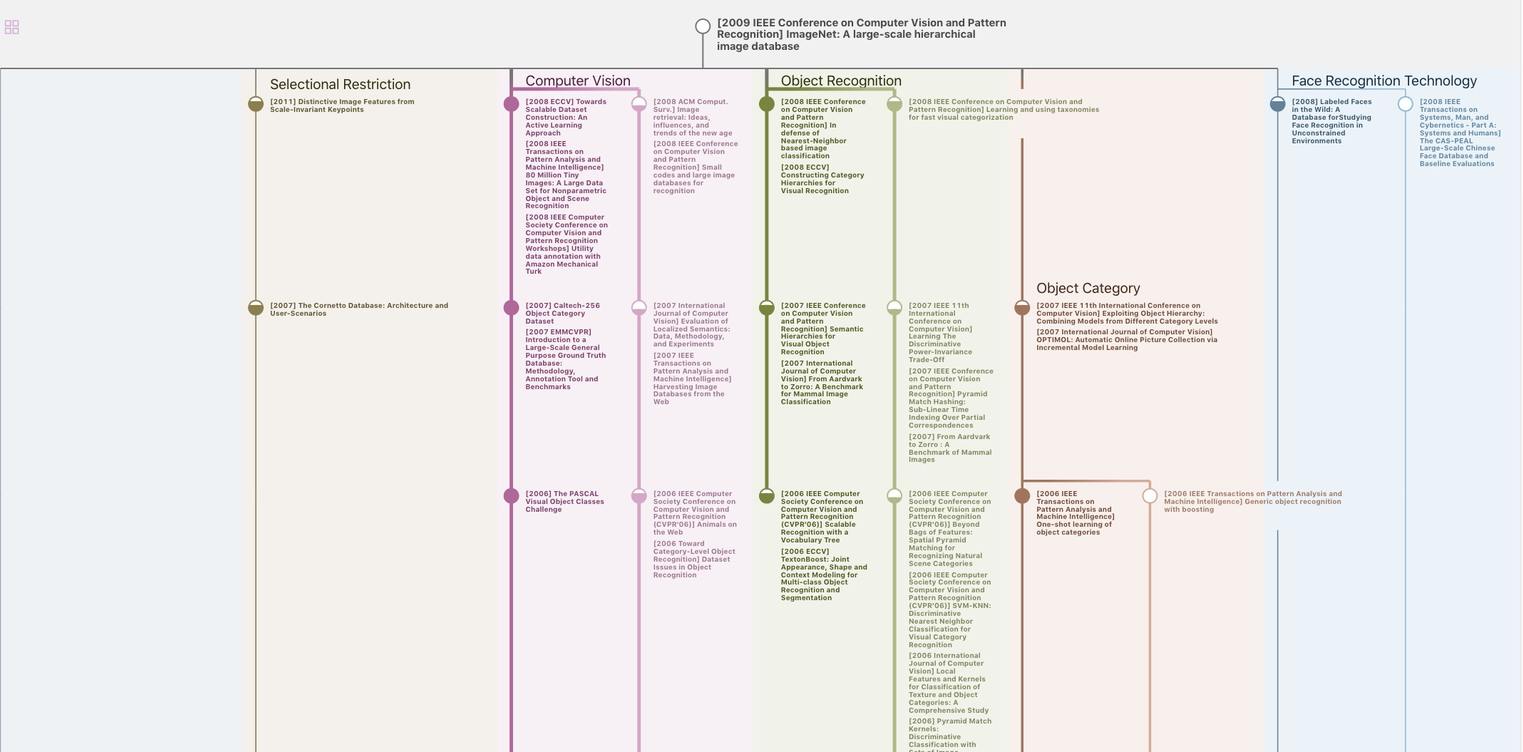
生成溯源树,研究论文发展脉络
Chat Paper
正在生成论文摘要