Continuous Streaming Multi-Talker ASR with Dual-Path Transducers.
IEEE International Conference on Acoustics, Speech, and Signal Processing (ICASSP)(2022)
摘要
Streaming recognition of multi-talker conversations has so far been evaluated only for 2-speaker single-turn sessions. In this paper, we investigate it for multi-turn meetings containing multiple speakers using the Streaming Unmixing and Recognition Transducer (SURT) model, and show that naively extending the single-turn model to this harder setting incurs a performance penalty. As a solution, we propose the dual-path (DP) modeling strategy first used for time-domain speech separation. We experiment with LSTM and Transformer based DP models, and show that they improve word error rate (WER) performance while yielding faster convergence. We also explore training strategies such as chunk width randomization and curriculum learning for these models, and demonstrate their importance through ablation studies. Finally, we evaluate our models on the LibriCSS meeting data, where they perform competitively with offline separation-based methods.
更多查看译文
关键词
Multi-talker ASR,long-form meeting transcription,dual-path RNN,transducer
AI 理解论文
溯源树
样例
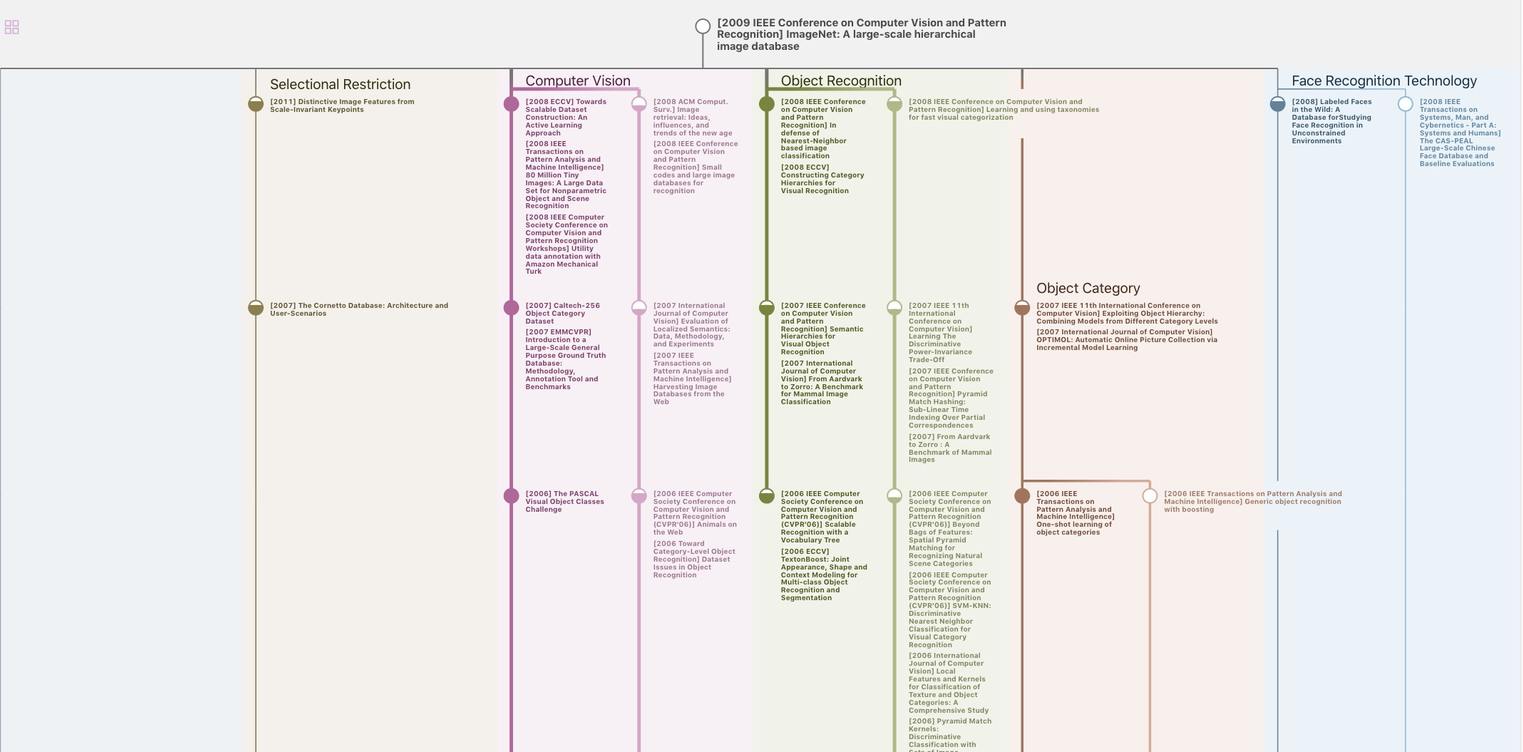
生成溯源树,研究论文发展脉络
Chat Paper
正在生成论文摘要