TS-MULE: Local Interpretable Model-Agnostic Explanations for Time Series Forecast Models
MACHINE LEARNING AND PRINCIPLES AND PRACTICE OF KNOWLEDGE DISCOVERY IN DATABASES, ECML PKDD 2021, PT I(2021)
摘要
Time series forecasting is a demanding task ranging from weather to failure forecasting with black-box models achieving state-of-the-art performances. However, understanding and debugging are not guaranteed. We propose TS-MULE, a local surrogate model explanation method specialized for time series extending the LIME approach. Our extended LIME works with various ways to segment and perturb the time series data. In our extension, we present six sampling segmentation approaches for time series to improve the quality of surrogate attributions and demonstrate their performances on three deep learning model architectures and three common multivariate time series datasets.
更多查看译文
关键词
Explainable AI, LIME, Time series
AI 理解论文
溯源树
样例
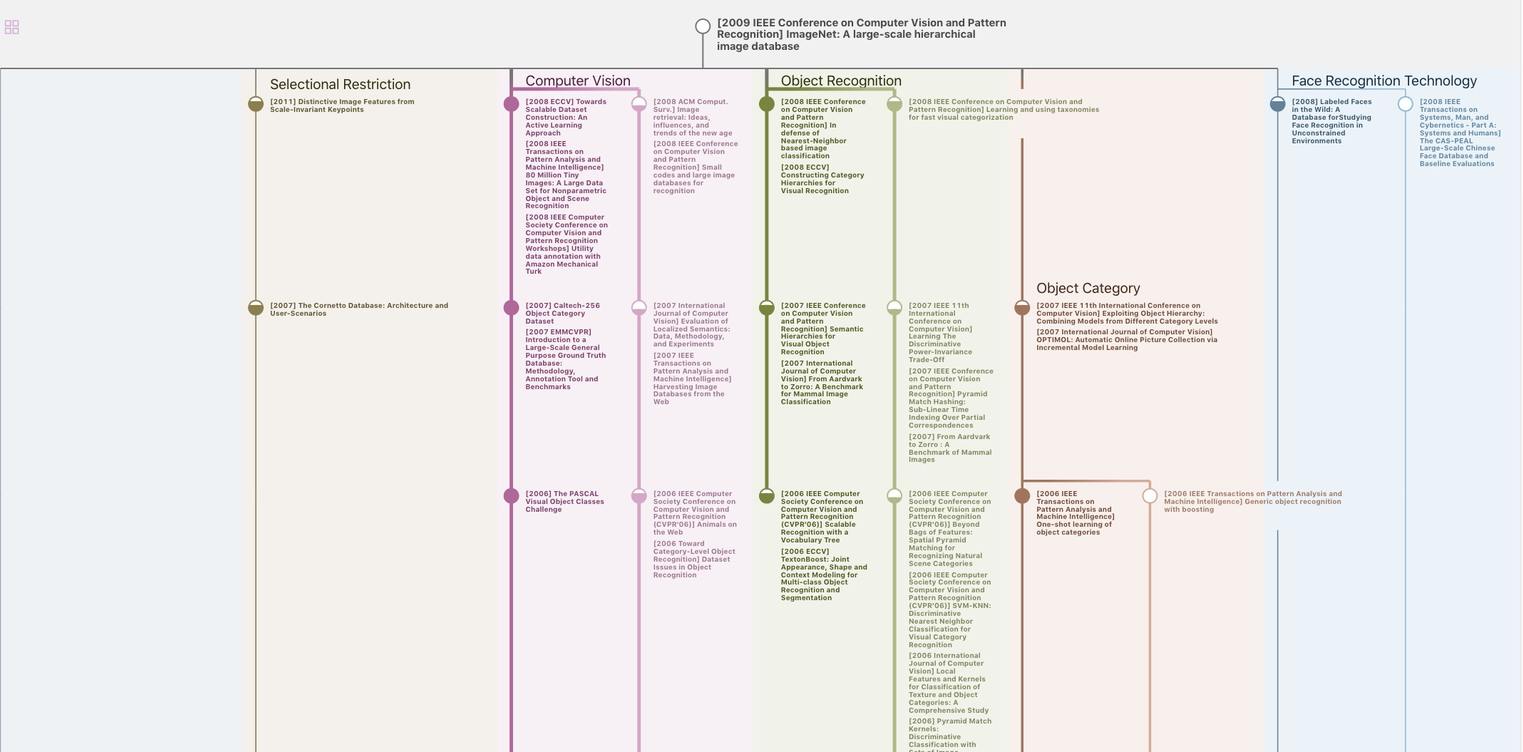
生成溯源树,研究论文发展脉络
Chat Paper
正在生成论文摘要