A Machine Learning Approach for Mapping Forest Categories: An Application of Google Earth Engine for the Case Study of Monte Sant'Angelo, Central Italy
COMPUTATIONAL SCIENCE AND ITS APPLICATIONS, ICCSA 2021, PT VII(2021)
摘要
Remote Sensing plays a critical role in forest tree species identification. Regarding the current debate on earth observation and monitoring, many see this valuable technology useful for a wide range of purposes, including forest conservation and management. This paper focuses on a workflow for mapping forest tree species from satellite images, by statistic algorithms and machine learning. Among the world satellite platforms, the Sentinel-2 program was selected to investigate the mixed forest area of Monte Sant'Angelo in Central Italy. A list of monthly images from 2018 to 2020 have been processed using the Google Earth Engine geospatial processing service. The process includes the computation of vegetation indexes like the Normalized Difference Vegetation Index (NDVI), Transformed Difference Vegetation Index (TDVI), the Enhanced Vegetation Index (EVI) and the Green Normalized Difference Vegetation Index (GNDVI). A forest class time series was generated for each index. The Artificial Intelligence algorithm models (Machine Learning) were trained identifying accurate ground truths. Principal Component Analysis (PCA) was performed to reduce variables redundancy in Random Forest classifications. Four forest categories have been identified: holm oak woodlands, conifer reforestation, Ostryo Carpinion alliance and mixed hardwood forest. Due to the phenological differences among species, the classification global accuracy ranges from 70% to 80%.
更多查看译文
关键词
Google Earth Engine, PCA, Random forest, Sentinel-2, Time-series, Tree species classification
AI 理解论文
溯源树
样例
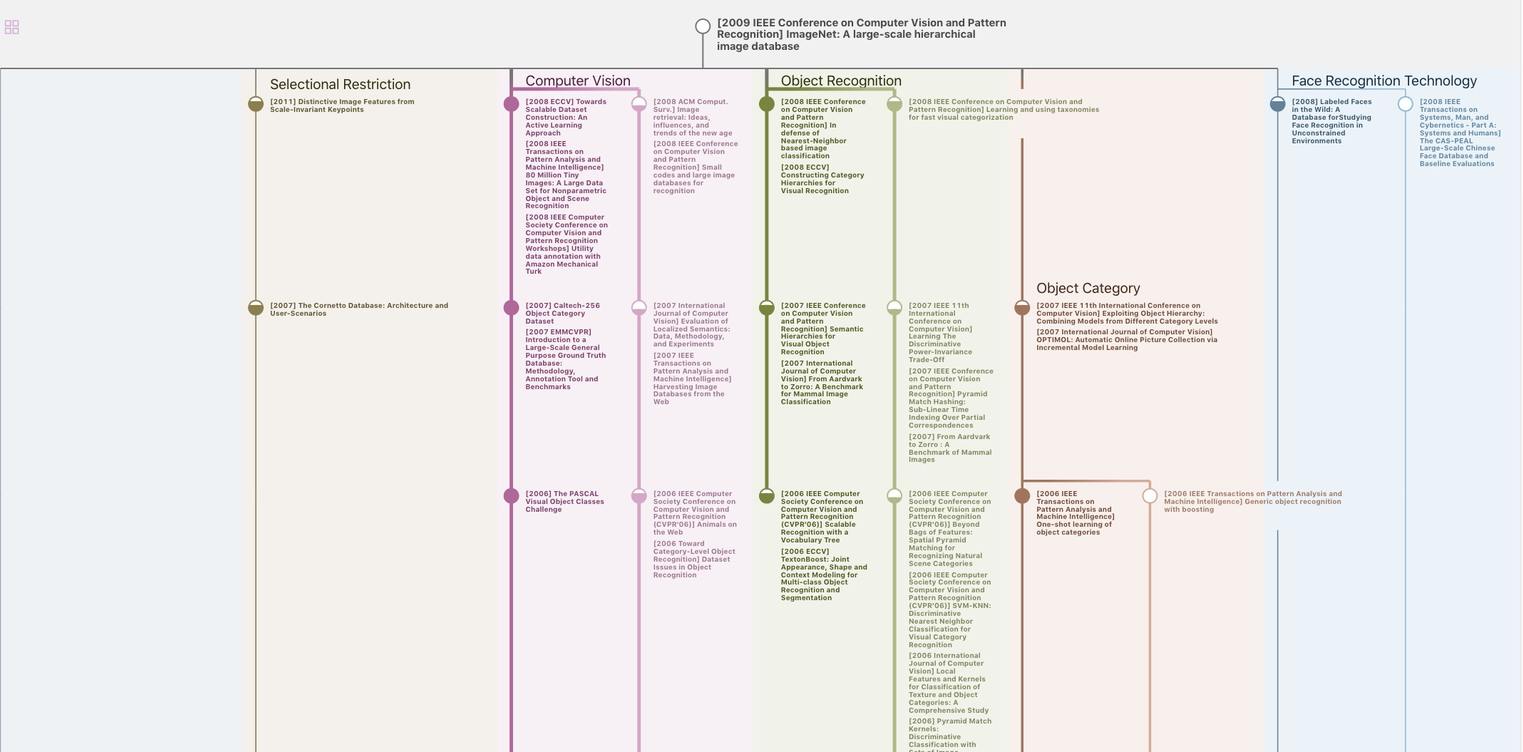
生成溯源树,研究论文发展脉络
Chat Paper
正在生成论文摘要