Deep Reinforcement Learning Based Dynamic Content Placement and Bandwidth Allocation in Internet of Vehicles
WIRELESS ALGORITHMS, SYSTEMS, AND APPLICATIONS, WASA 2021, PT III(2021)
摘要
With the rapid development of Internet of vehicles (IoV), the emerging in-vehicle applications meet people's demand for intelligent experience, while also increasing the cost of network communication. Driven by the fact that a few popular content accounted for most of the traffic load, caching popular content at the wireless edge has become a trend of content delivery. However, the limitation of cache resources and the ever-changing mobile environment bring a severe challenge to content delivery cost optimization. In this paper, we first investigate the joint optimization of content placement and bandwidth allocation (JOCB) in IoV. In order to minimize the long-term system cost in the mobile scenario, we first establish an innovative vehicle mobility model based on the probability distribution. Then we use the Lagrangian multiplier method to solve the bandwidth allocation sub-problem to reduce the algorithm complexity. Considering the high-dimensional continuity of action and state spaces, the original problem is transformed into a Markov Decision Process (MDP), and we propose a Deep reinforcement learning based Dynamic Content Placement (DDCP) algorithm. DDCP algorithm has low algorithm complexity and can handle high-dimensional continuous state and action space. The simulation results show that compare with other three benchmark algorithms, DDCP algorithm can reduce the system cost effectively.
更多查看译文
关键词
Internet of Vehicles, Edge cache, Content placement, Bandwidth allocation, Deep reinforcement learning
AI 理解论文
溯源树
样例
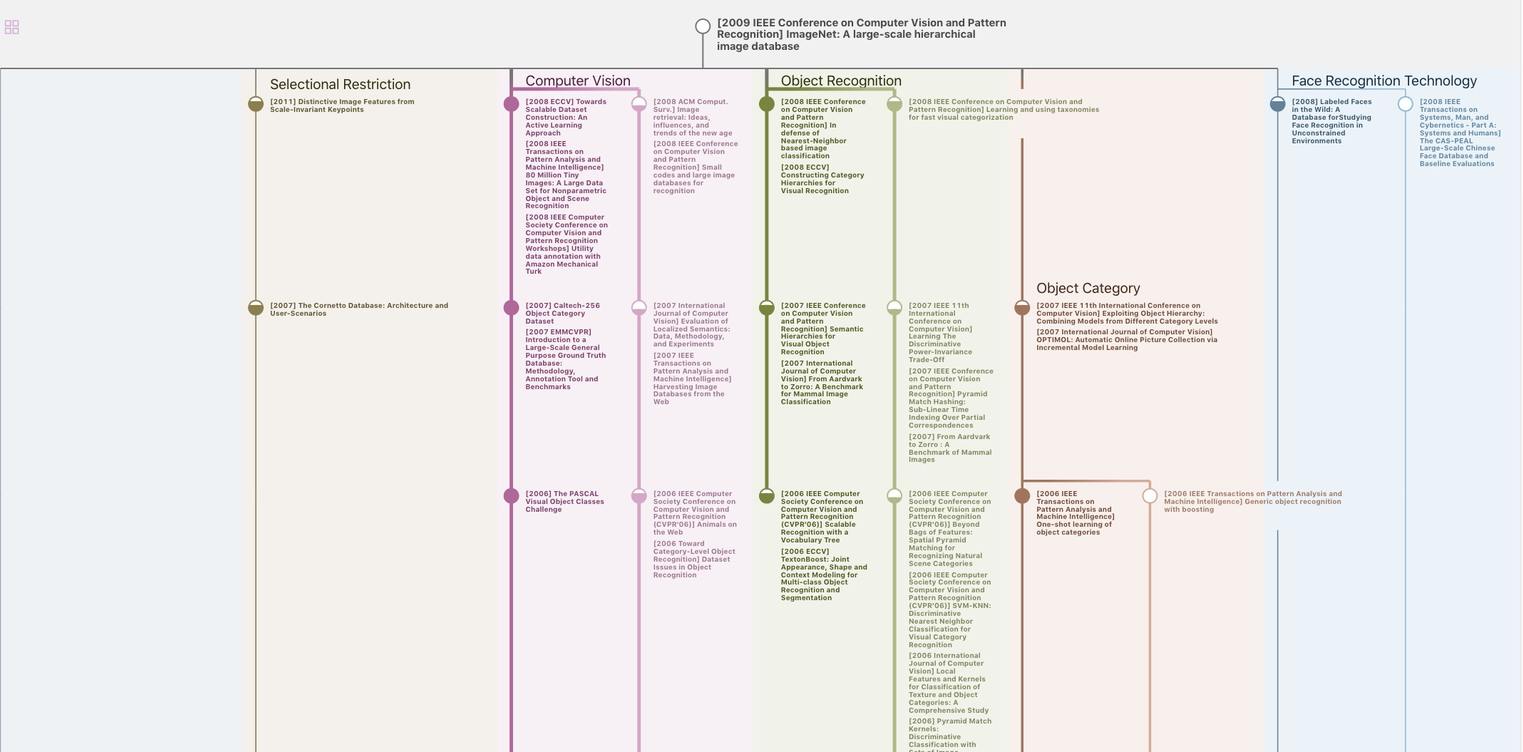
生成溯源树,研究论文发展脉络
Chat Paper
正在生成论文摘要