Learning Observation-Based Certifiable Safe Policy for Decentralized Multi-Robot Navigation
IEEE International Conference on Robotics and Automation(2022)
摘要
Safety is of great importance in multi-robot navigation problems. In this paper, we propose a control barrier function (CBF) based optimizer that ensures robot safety with both high probability and flexibility, using only sensor measurement. The optimizer takes action commands from the policy network as initial values and provides refinement to drive the potentially dangerous ones back into safe regions. With the help of a deep world model that predicts the evolution of surrounding dynamics and the consequences of different actions, the CBF module can guide the optimization within a reasonable time horizon. We also present a novel joint training framework that improves the cooperation between the Reinforcement Learning (RL) based policy and the CBF-based optimizer by utilizing reward feedback from the CBF module. We observe that our policy can achieve a higher success rate while maintaining the safety of multiple robots in significantly fewer episodes. Experiments are conducted in multiple scenarios both in simulation and the real world, the results demonstrate the effectiveness of our method in maintaining the safety of multiple robots. Code is available at https://github.com/YuxiangCui/MARL-OCBF.
更多查看译文
关键词
utilizing reward feedback,CBF module,multiple robots,observation-based certifiable safe policy,decentralized multirobot navigation,multirobot navigation problems,control barrier function based optimizer,robot safety,flexibility,sensor measurement,policy network,initial values,potentially dangerous ones,safe regions,deep world model,reasonable time horizon,joint training framework,CBF-based optimizer
AI 理解论文
溯源树
样例
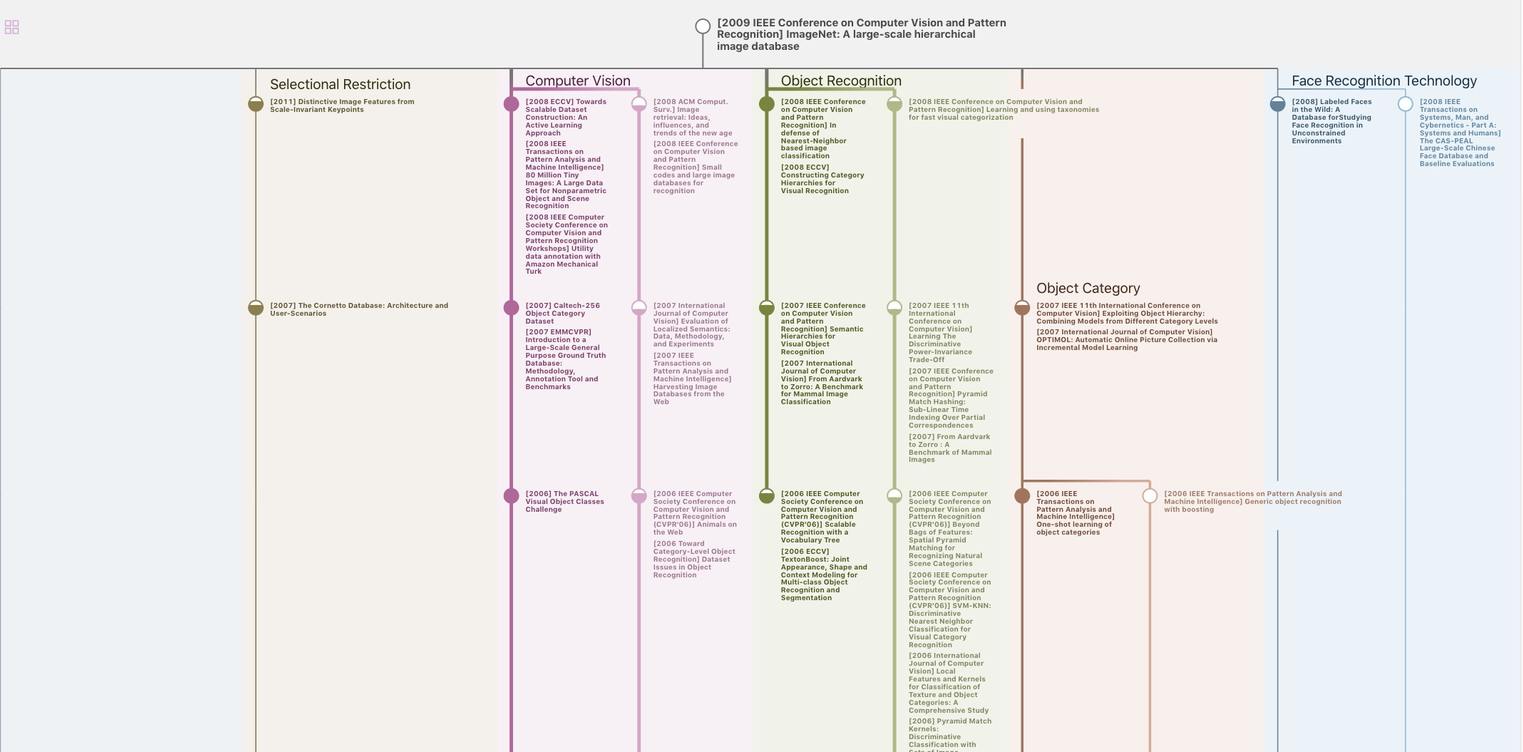
生成溯源树,研究论文发展脉络
Chat Paper
正在生成论文摘要