Learning Noisy Hedonic Games
arxiv(2021)
摘要
We consider the learning task of prediction of formation of core stable coalition structure in hedonic games based on agents' noisy preferences. We have considered two cases: complete information (noisy preferences of all the agents are entirely known) and partial information (noisy preferences over some coalitions are only known). We introduce a noise model that probabilistically scales the valuations of coalitions. The performance metric is the probability of our prediction conditioned on all or few noisy preferences of the agents be correct. The nature of our results is that this prediction probability is relatively low, including being zero, and rarely it is one. In the complete information two-agent model, in which each agent `retains' or `inflates' the values of its coalitions, we identify the expressions of the prediction probabilities in terms of the noise probability. We identify the interval of the noise probability such that the prediction probability is at least a user-given threshold. It turned out that, for some noisy games, the noise probability interval does not exist for a threshold as low as 0.1481, thus demonstrating that the prediction probabilities are generally low even in this model. In the partial information setup, we consider $n$ agent games with $l$ support of noise values, and such noisy preferences are available for some coalitions only. We obtain the bounds on the prediction probability of a partition to be $\epsilon$-PAC stable in the noise-free game in the cases when the realized noisy game has or hasn't $\epsilon$-PAC stable outcome.
更多查看译文
关键词
noisy hedonic games,learning
AI 理解论文
溯源树
样例
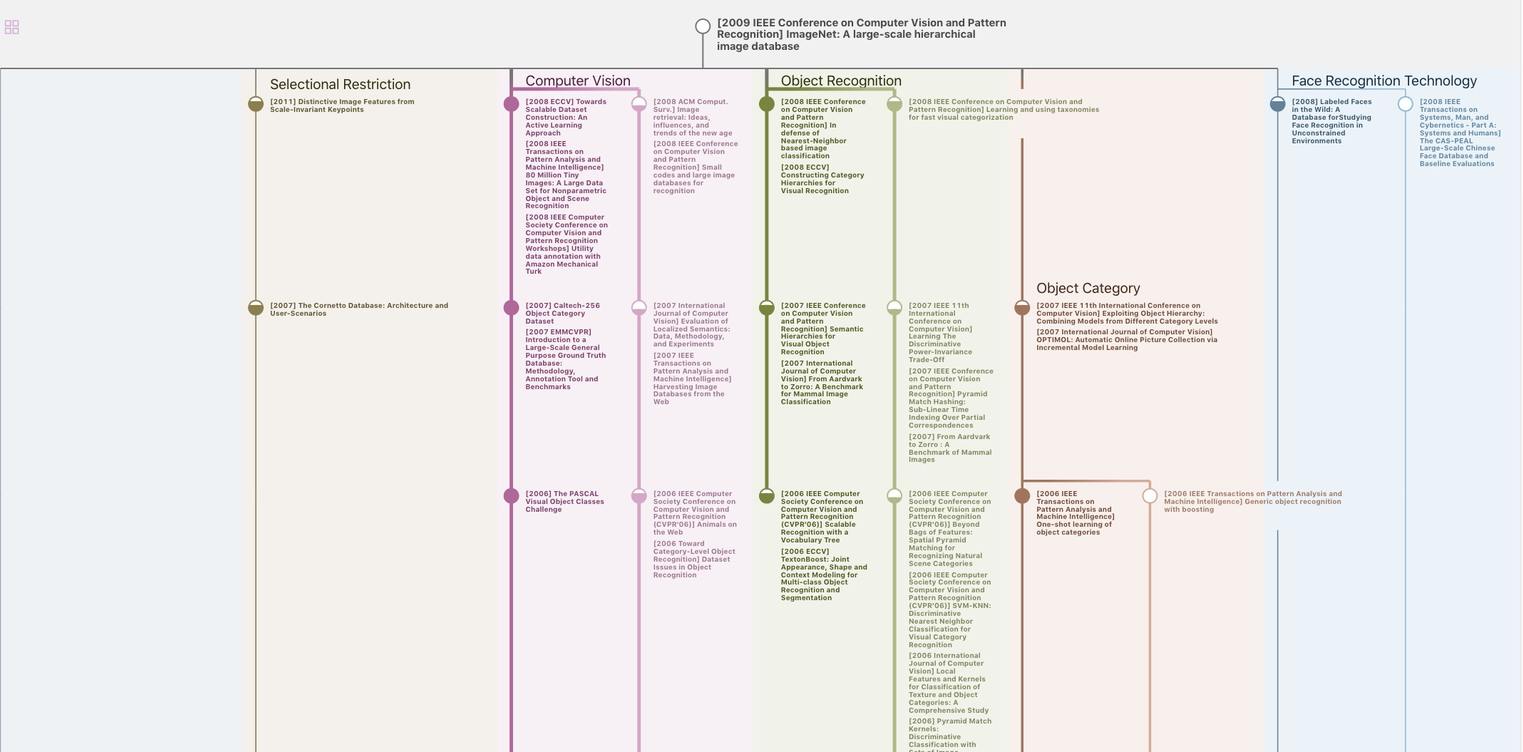
生成溯源树,研究论文发展脉络
Chat Paper
正在生成论文摘要