Iterative Error Removal for Time-of-Flight Depth Imaging
ARTIFICIAL NEURAL NETWORKS AND MACHINE LEARNING - ICANN 2021, PT II(2021)
摘要
Depth information plays an increasingly important role in computer vision tasks. As one of the most promising depth sensing techniques, Amplitude Modulated Continuous Wave (AMCW)-based indirect Time-of-Flight (ToF) has been widely used in recent years. Unfortunately, the depth acquired by ToF sensors is often corrupted by imaging noise, multi-path interference (MPI), and low intensity. Different methods have been proposed for tackling these issues. Nevertheless, they failed to exploit the characteristics of the ToF depth map to propose a targeted solution, and are unable to achieve various error removal. We present a new iterative method for removing various errors simultaneously through cascaded Convolutional Neural Networks (CNNs). A Synthetic Dataset is created using computer graphics, and a Real-World Dataset is developed via RGBD-based 3D reconstruction, both contain the raw measurement acquired by a certain ToF camera and corresponding dense ground truth depth. Experimental results demonstrate the superior performance of the proposed iterative method in removing various ToF depth errors, compared to state-of-the-art methods, on both the newly developed datasets and existing public datasets.
更多查看译文
关键词
Time-of-flight, Convolutional neural networks, Three-dimensional vision, Depth sensor
AI 理解论文
溯源树
样例
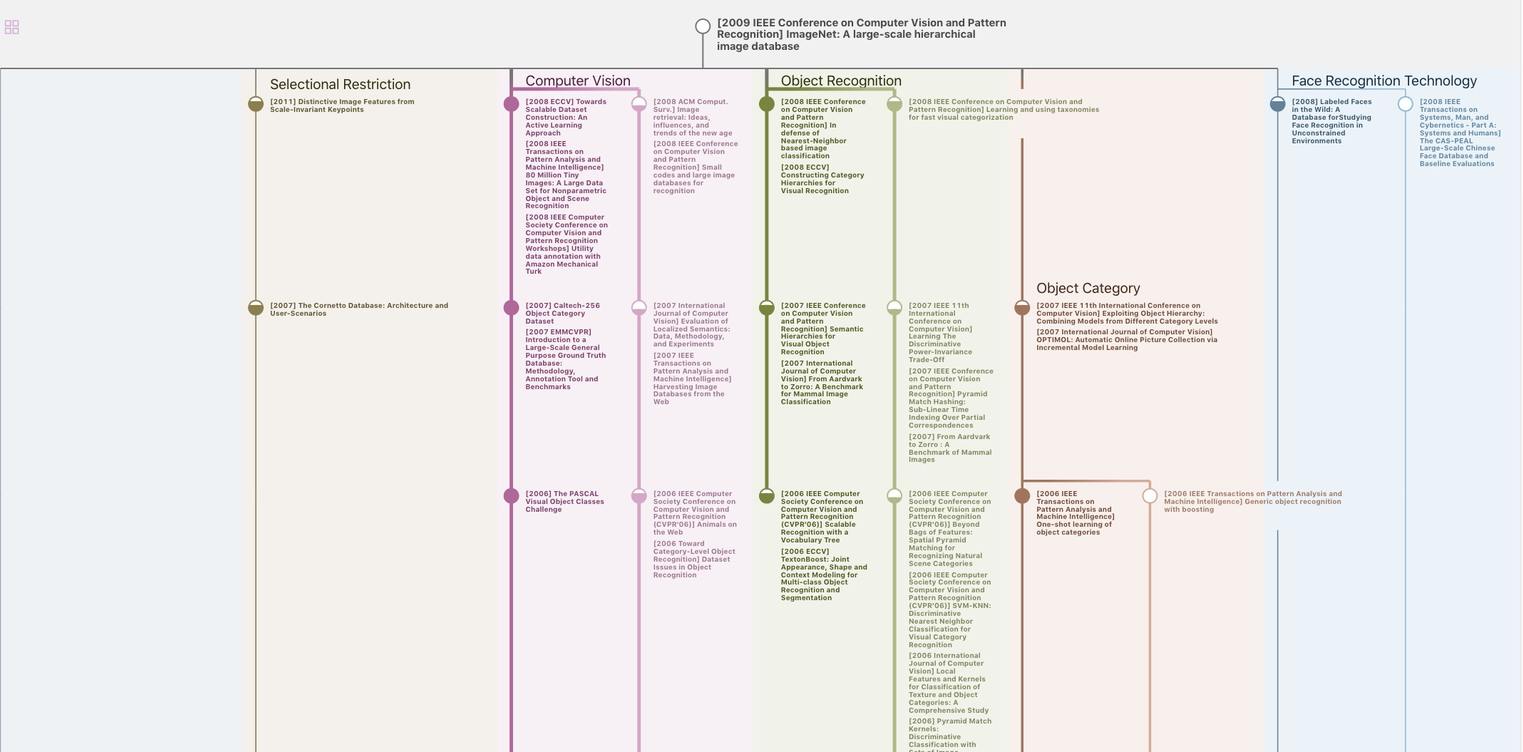
生成溯源树,研究论文发展脉络
Chat Paper
正在生成论文摘要