Generalised Controller Design Using Continual Learning
ARTIFICIAL NEURAL NETWORKS AND MACHINE LEARNING - ICANN 2021, PT II(2021)
摘要
In control systems applications, controllers for different plants are usually designed with different methods. Although plants may share common characteristics, these controllers are generally designed in isolation. The problem of continually learning a sequence of related tasks has been extensively studied recently. A challenge in continual learning is the phenomenon of catastrophic forgetting of knowledge of previous tasks which have been integrated into a neural network model. In this paper we evaluate the feasibility of modelling different controllers using continual learning. We explore regression versions of state-of-the-art methods and demonstrate that even the simplest continual learning approach decreases the overall Mean Average Error (MAE) by 39% of the MAE achieved by a non-continual strategy. Furthermore, a method based on dynamically expanding the network can achieve an overall MAE which is only 18% of the non-continual MAE. We also propose a set of new metrics that allow us to characterise the nature of catastrophic forgetting experienced while using different continual learning methods.
更多查看译文
关键词
Continual learning, Catastrophic forgetting
AI 理解论文
溯源树
样例
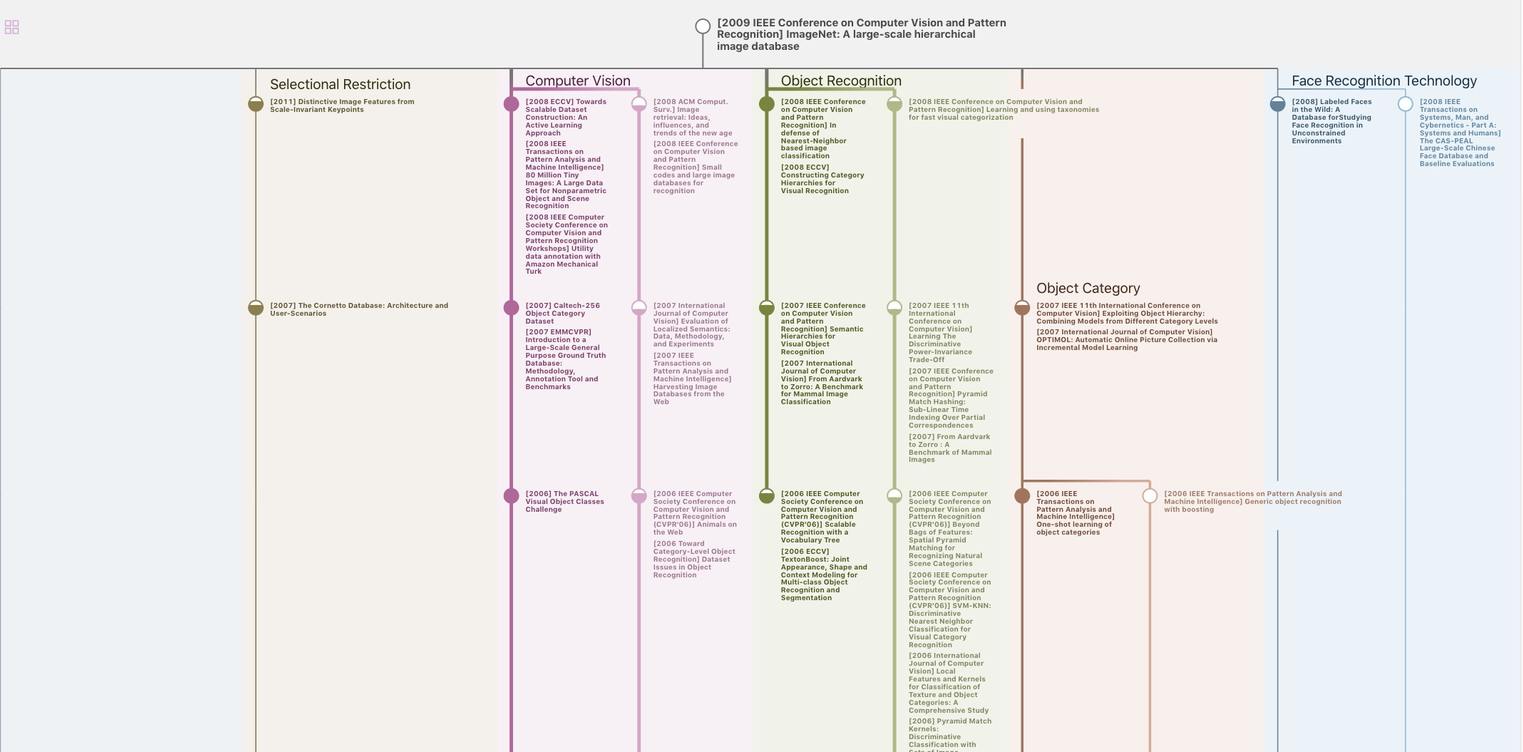
生成溯源树,研究论文发展脉络
Chat Paper
正在生成论文摘要