Attention-Based 3D Neural Architectures for Predicting Cracks in Designs
ARTIFICIAL NEURAL NETWORKS AND MACHINE LEARNING - ICANN 2021, PT I(2021)
Abstract
The rapid and accurate prediction of residual stresses in metal additive manufacturing (3D printing) processes is crucial to ensuring defect-free fabrication of parts used in critical industrial applications. This paper presents promising outcomes from applying attention-based neural architectures for predicting such 3D stress phenomena accurately, efficiently, and reliably. This capability is critical to drastically reducing the design maturation time for additively manufactured parts. High fidelity, physics-based numerical models of the additive melting process exist that can simulate the thermal gradients and consequent stresses produced during manufacturing, which can then be used to synthesize a 3D crack index field for the entire part volume, capturing the likelihood that a region in a part will crack upon heat treatment. However, these models are expensive and time-consuming to run. In response, a Deep Convolutional Neural Network (DCNN) model is explored as a surrogate for the physics-based model, so that it can be used to time-efficiently estimate the crack index for a given part-design. This requires careful design of the training regime and dataset for a given design problem. Using the U-Net architecture as the baseline, we expand the standard 2D application of this architecture for segmentation to the estimation of the full 3D, continuous valued, stress field. We illustrate the primary challenge faced by the standard U-Net architecture with L2-loss arising from sparsity in critical values of the crack index and show how augmenting the architecture with attention mechanisms helps address the issue as well as improve the overall accuracy of estimation.
MoreTranslated text
Key words
Attention mechanism, 3D segmentation, Regression, Additive manufacturing, 3D printing, Generative design
AI Read Science
Must-Reading Tree
Example
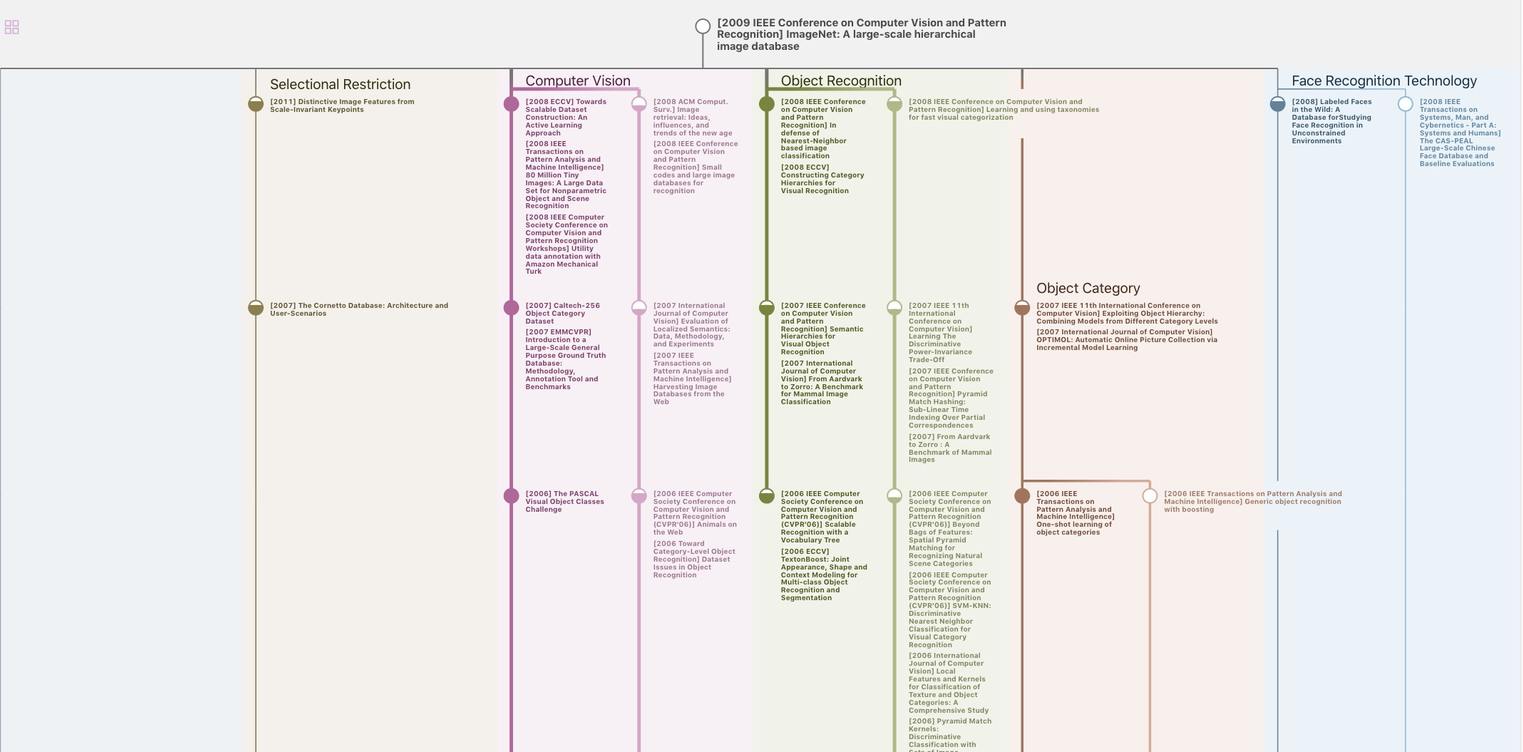
Generate MRT to find the research sequence of this paper
Chat Paper
Summary is being generated by the instructions you defined