Training Many-to-Many Recurrent Neural Networks with Target Propagation
ARTIFICIAL NEURAL NETWORKS AND MACHINE LEARNING - ICANN 2021, PT IV(2021)
摘要
Deep neural networks trained with back-propagation have been the driving force for the progress in fields such as computer vision, natural language processing. However, back-propagation has often been criticized for its biological implausibility. More biologically plausible alternatives to backpropagation such as target propagation and feedback alignment have been proposed. But most of these learning algorithms are originally designed and tested for feedforward networks, and their ability for training recurrent networks and arbitrary computation graphs is not fully studied nor understood. In this paper, we propose a learning procedure based on target propagation for training multi-output recurrent networks. It opens doors to extending such biologically plausible models as general learning algorithms for arbitrary graphs.
更多查看译文
关键词
Artificial neural networks, Recurrent neural networks, Biologically plausible learning, Target propagation, Backpropagation
AI 理解论文
溯源树
样例
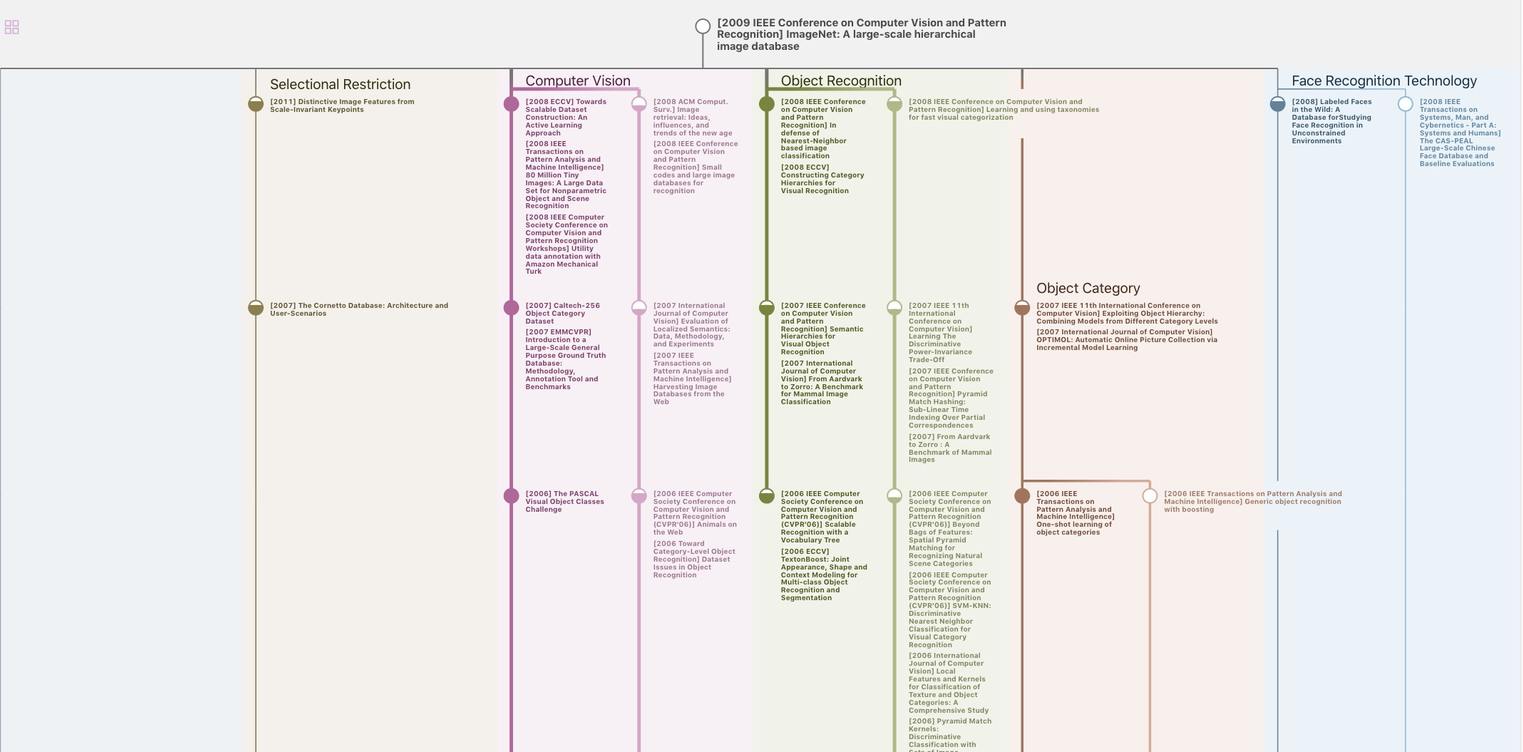
生成溯源树,研究论文发展脉络
Chat Paper
正在生成论文摘要