Allocating Large Vocabulary Capacity for Cross-Lingual Language Model Pre-Training.
EMNLP(2021)
Abstract
Compared to monolingual models, cross-lingual models usually require a more expressive vocabulary to represent all languages adequately. We find that many languages are under-represented in recent cross-lingual language models due to the limited vocabulary capacity. To this end, we propose an algorithm VoCap to determine the desired vocabulary capacity of each language. However, increasing the vocabulary size significantly slows down the pre-training speed. In order to address the issues, we propose k-NN-based target sampling to accelerate the expensive softmax. Our experiments show that the multilingual vocabulary learned with VoCap benefits cross-lingual language model pre-training. Moreover, k-NN-based target sampling mitigates the side-effects of increasing the vocabulary size while achieving comparable performance and faster pre-training speed. The code and the pretrained multilingual vocabularies are available at https://github.com/bozheng-hit/VoCapXLM.
MoreTranslated text
Key words
large vocabulary capacity,language,cross-lingual,pre-training
AI Read Science
Must-Reading Tree
Example
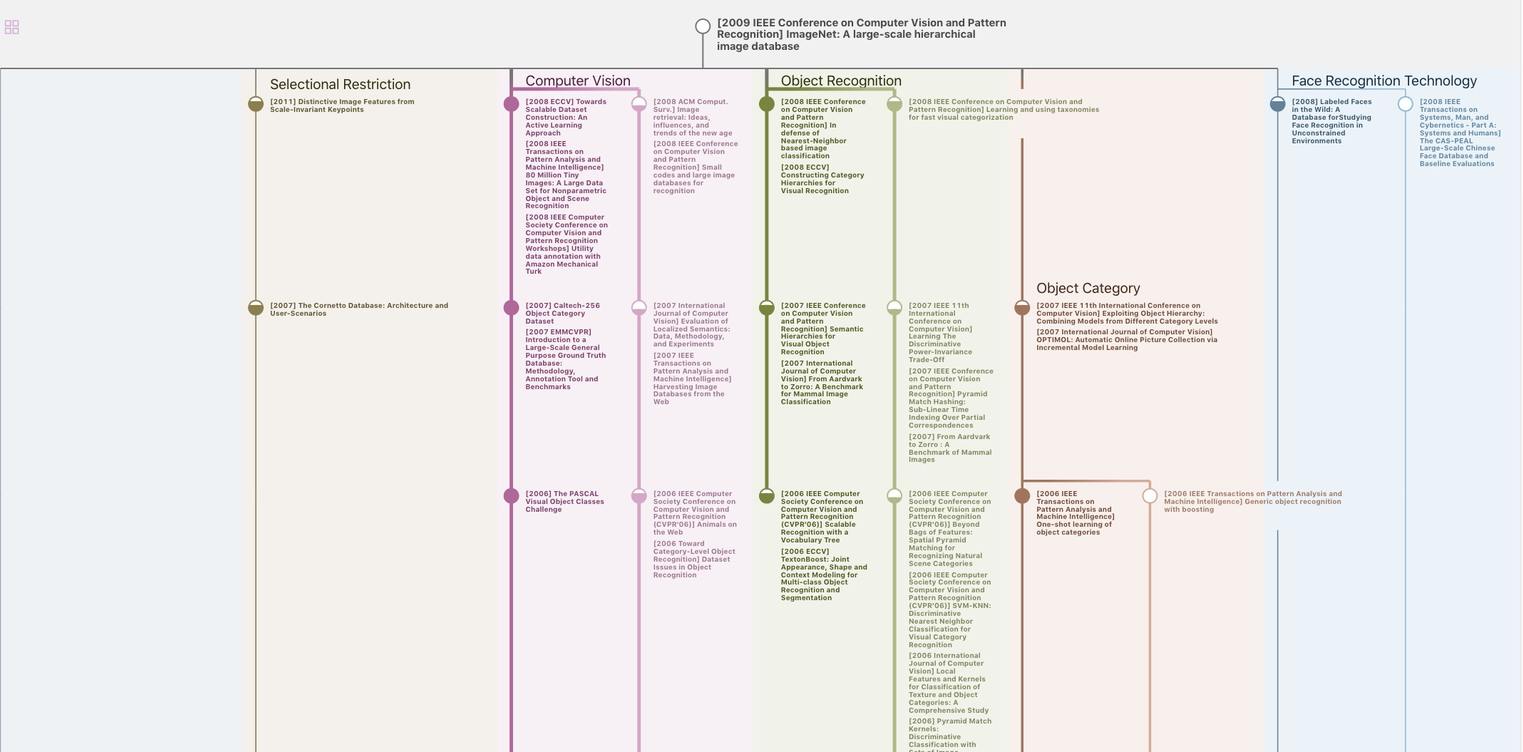
Generate MRT to find the research sequence of this paper
Chat Paper
Summary is being generated by the instructions you defined