Quantitative reconstruction of defects in multi-layered bonded composites using fully convolutional network-based ultrasonic inversion
Journal of Sound and Vibration(2023)
摘要
Ultrasonic methods have great potential applications to detect and characterize defects in multi-layered bonded composites. However, it remains challenging to quantitatively reconstruct defects, such as disbonds, that influence the integrity of adhesive bonds and seriously reduce the strength of assemblies. In this work, an ultrasonic method based on the supervised fully convolutional network (FCN) is proposed to quantitatively reconstruct high contrast defects hidden in multi-layered bonded composites. In the training process of this method, an FCN establishes a non-linear mapping from measured ultrasonic data to the corresponding longitudinal wave (L-wave) velocity models of multi-layered bonded composites. In the predicting process, the network obtained from the training process is used to directly reconstruct the L-wave velocity models from the new measured ultrasonic data of adhesively bonded composites. The presented FCN-based inversion method can automatically extract useful features in multi-layered composites. Although this method is computationally expensive in the training process, the prediction itself in the online phase takes only seconds. The numerical and experimental results show that the FCN-based ultrasonic inversion method is capable of accurately reconstructing ultrasonic L-wave velocity models of the high contrast defects, which has great potential for online detection of adhesively bonded composites.
更多查看译文
关键词
Quantitative reconstruction,Adhesively bonded composites,Deep learning inversion,Ultrasonic imaging,Defect detection
AI 理解论文
溯源树
样例
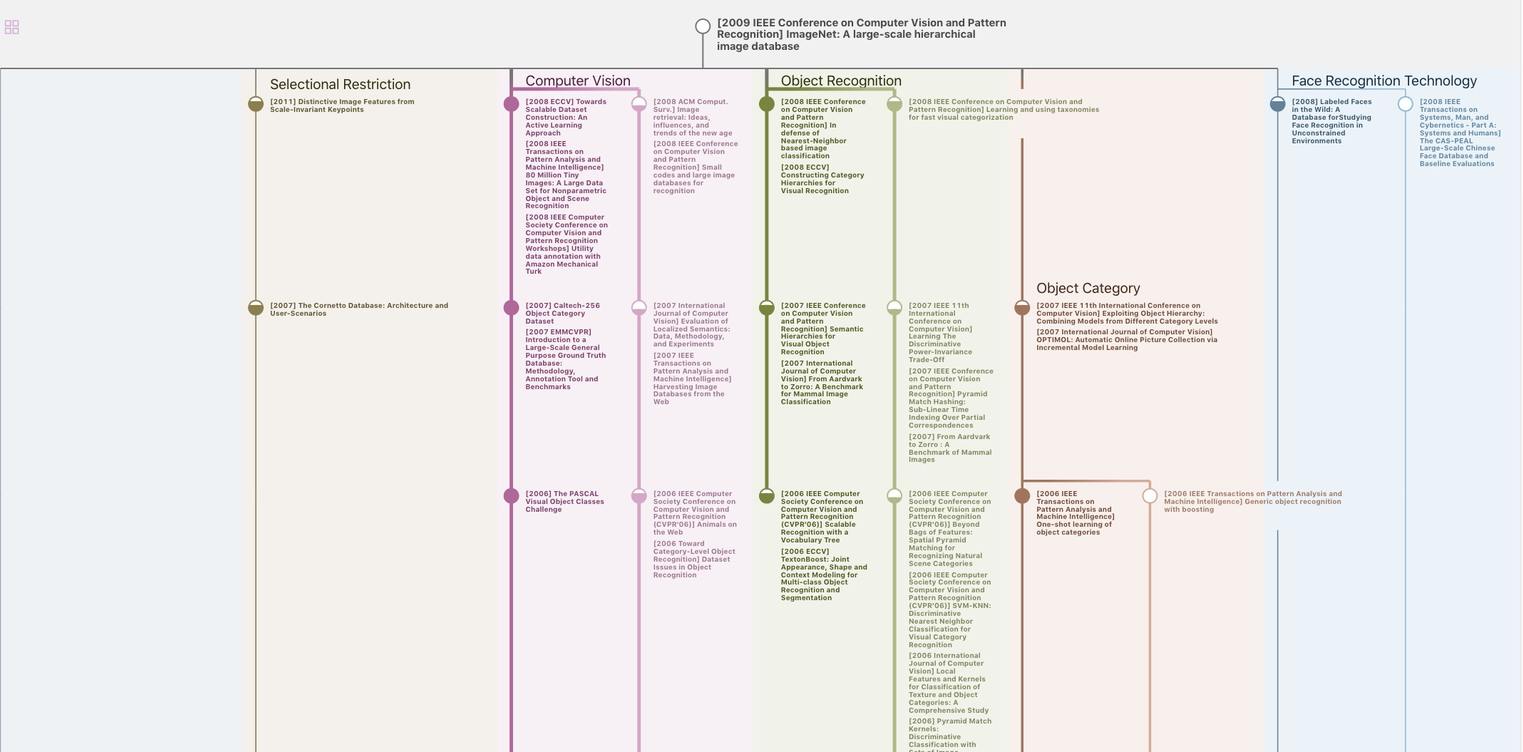
生成溯源树,研究论文发展脉络
Chat Paper
正在生成论文摘要