Robust Contrastive Active Learning with Feature-guided Query Strategies
arxiv(2022)
摘要
We introduce supervised contrastive active learning (SCAL) and propose efficient query strategies in active learning based on the feature similarity (featuresim) and principal component analysis based feature-reconstruction error (fre) to select informative data samples with diverse feature representations. We demonstrate our proposed method achieves state-of-the-art accuracy, model calibration and reduces sampling bias in an active learning setup for balanced and imbalanced datasets on image classification tasks. We also evaluate robustness of model to distributional shift derived from different query strategies in active learning setting. Using extensive experiments, we show that our proposed approach outperforms high performing compute-intensive methods by a big margin resulting in 9.9% lower mean corruption error, 7.2% lower expected calibration error under dataset shift and 8.9% higher AUROC for out-of-distribution detection.
更多查看译文
关键词
robust contrastive active learning,query,feature-guided
AI 理解论文
溯源树
样例
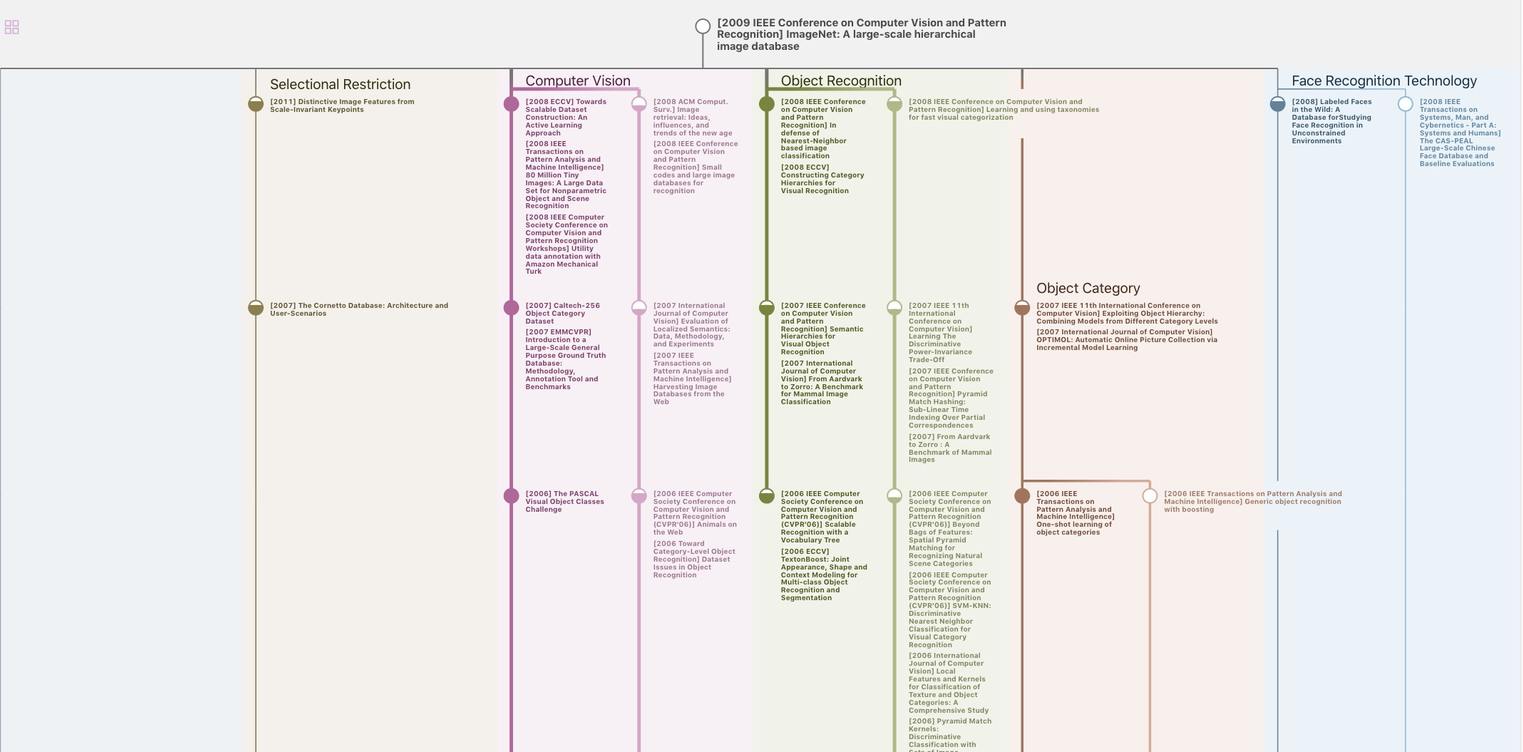
生成溯源树,研究论文发展脉络
Chat Paper
正在生成论文摘要