GALOPP: Multi-Agent Deep Reinforcement Learning For Persistent Monitoring With Localization Constraints
CoRR(2021)
Abstract
Persistently monitoring a region under localization and communication constraints is a challenging problem. In this paper, we consider a heterogenous robotic system consisting of two types of agents -- anchor agents that have accurate localization capability, and auxiliary agents that have low localization accuracy. The auxiliary agents must be within the communication range of an {anchor}, directly or indirectly to localize itself. The objective of the robotic team is to minimize the uncertainty in the environment through persistent monitoring. We propose a multi-agent deep reinforcement learning (MADRL) based architecture with graph attention called Graph Localized Proximal Policy Optimization (GALLOP), which incorporates the localization and communication constraints of the agents along with persistent monitoring objective to determine motion policies for each agent. We evaluate the performance of GALLOP on three different custom-built environments. The results show the agents are able to learn a stable policy and outperform greedy and random search baseline approaches.
MoreTranslated text
AI Read Science
Must-Reading Tree
Example
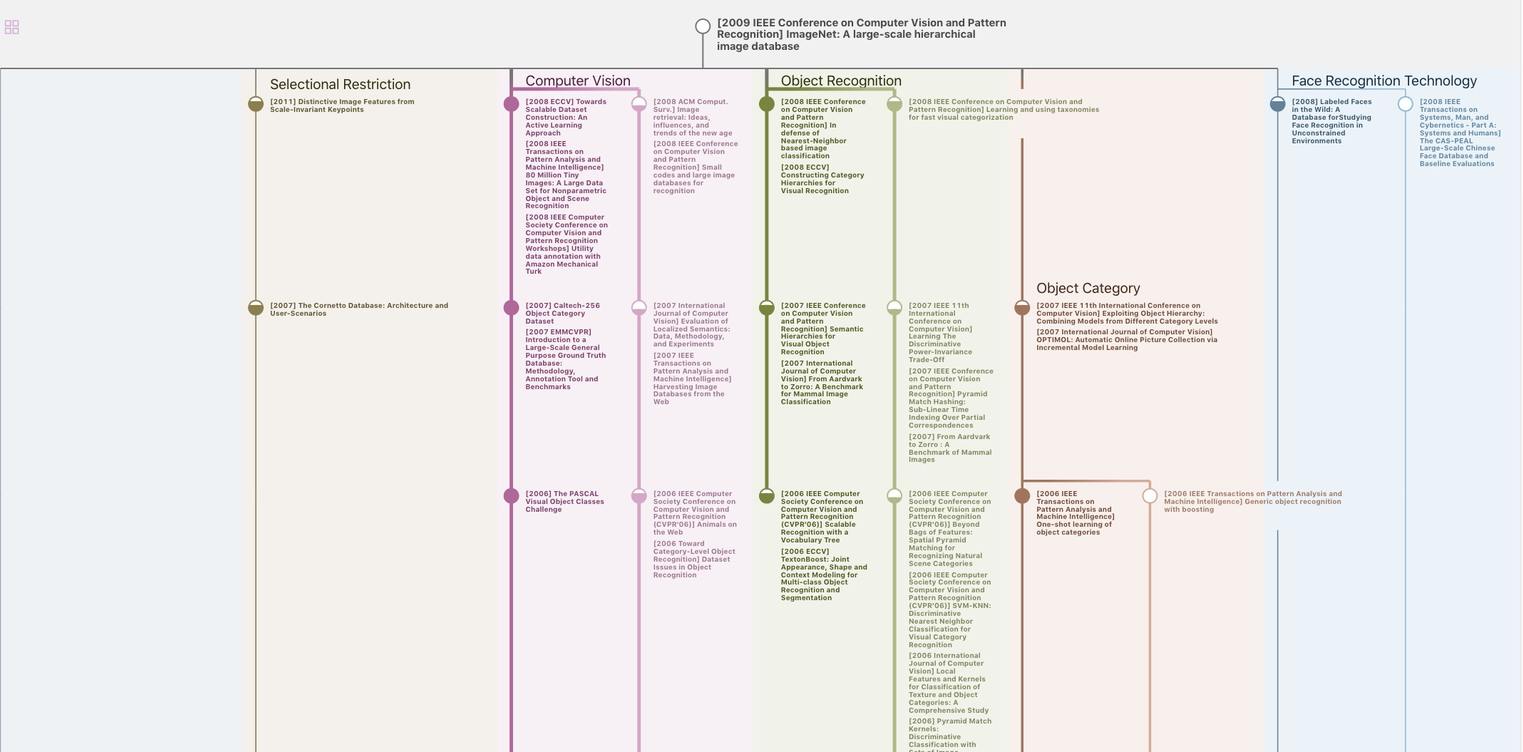
Generate MRT to find the research sequence of this paper
Chat Paper
Summary is being generated by the instructions you defined