Reactive and Safe Road User Simulations using Neural Barrier Certificates
2021 IEEE/RSJ INTERNATIONAL CONFERENCE ON INTELLIGENT ROBOTS AND SYSTEMS (IROS)(2021)
摘要
Reactive and safe agent modellings are important for nowadays traffic simulator designs and safe planning applications. In this work, we proposed a reactive agent model which can ensure safety without comprising the original purposes, by learning only high-level decisions from expert data and a low level decentralized controller guided by the jointly learned decentralized barrier certificates. Empirical results show that our learned road user simulation models can achieve a significant improvement in safety comparing to state-of-the-art imitation learning and pure control-based methods, while being similar to human agents by having smaller error to the expert data. Moreover, our learned reactive agents are shown to generalize better to unseen traffic conditions, and react better to other road users and therefore can help understand challenging planning problems pragmatically.
更多查看译文
关键词
reactive safe road user simulations,neural barrier certificates,reactive agent modellings,safe agent modellings,nowadays traffic simulator designs,safe planning applications,reactive agent model,original purposes,high-level decisions,expert data,low level decentralized controller,jointly learned decentralized barrier certificates,learned road user simulation models,safety comparing,state-of-the-art imitation learning,pure control-based methods,human agents,learned reactive agents,unseen traffic conditions,road users
AI 理解论文
溯源树
样例
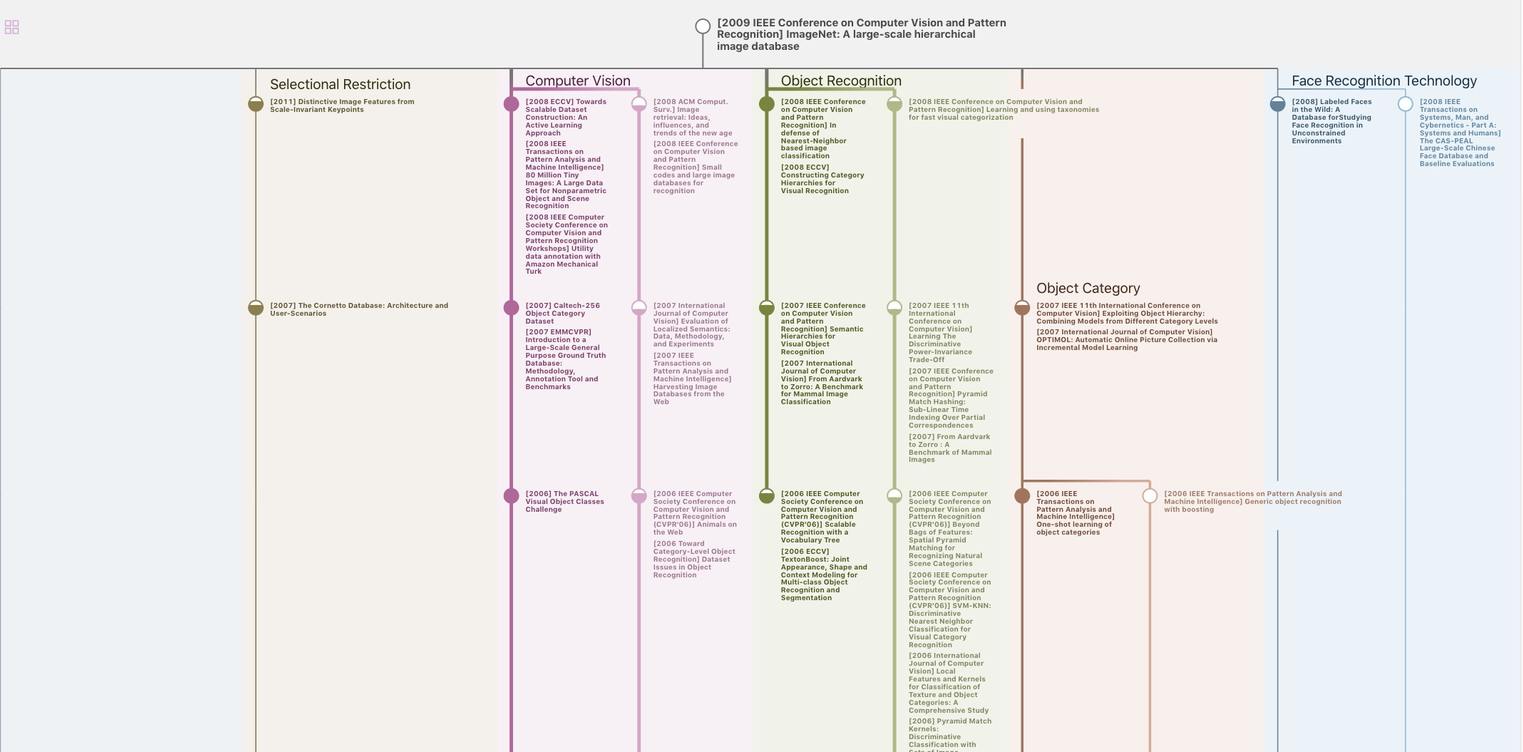
生成溯源树,研究论文发展脉络
Chat Paper
正在生成论文摘要