TREATED:Towards Universal Defense against Textual Adversarial Attacks
arxiv(2021)
摘要
Recent work shows that deep neural networks are vulnerable to adversarial examples. Much work studies adversarial example generation, while very little work focuses on more critical adversarial defense. Existing adversarial detection methods usually make assumptions about the adversarial example and attack method (e.g., the word frequency of the adversarial example, the perturbation level of the attack method). However, this limits the applicability of the detection method. To this end, we propose TREATED, a universal adversarial detection method that can defend against attacks of various perturbation levels without making any assumptions. TREATED identifies adversarial examples through a set of well-designed reference models. Extensive experiments on three competitive neural networks and two widely used datasets show that our method achieves better detection performance than baselines. We finally conduct ablation studies to verify the effectiveness of our method.
更多查看译文
关键词
textual adversarial attacks,universal defense
AI 理解论文
溯源树
样例
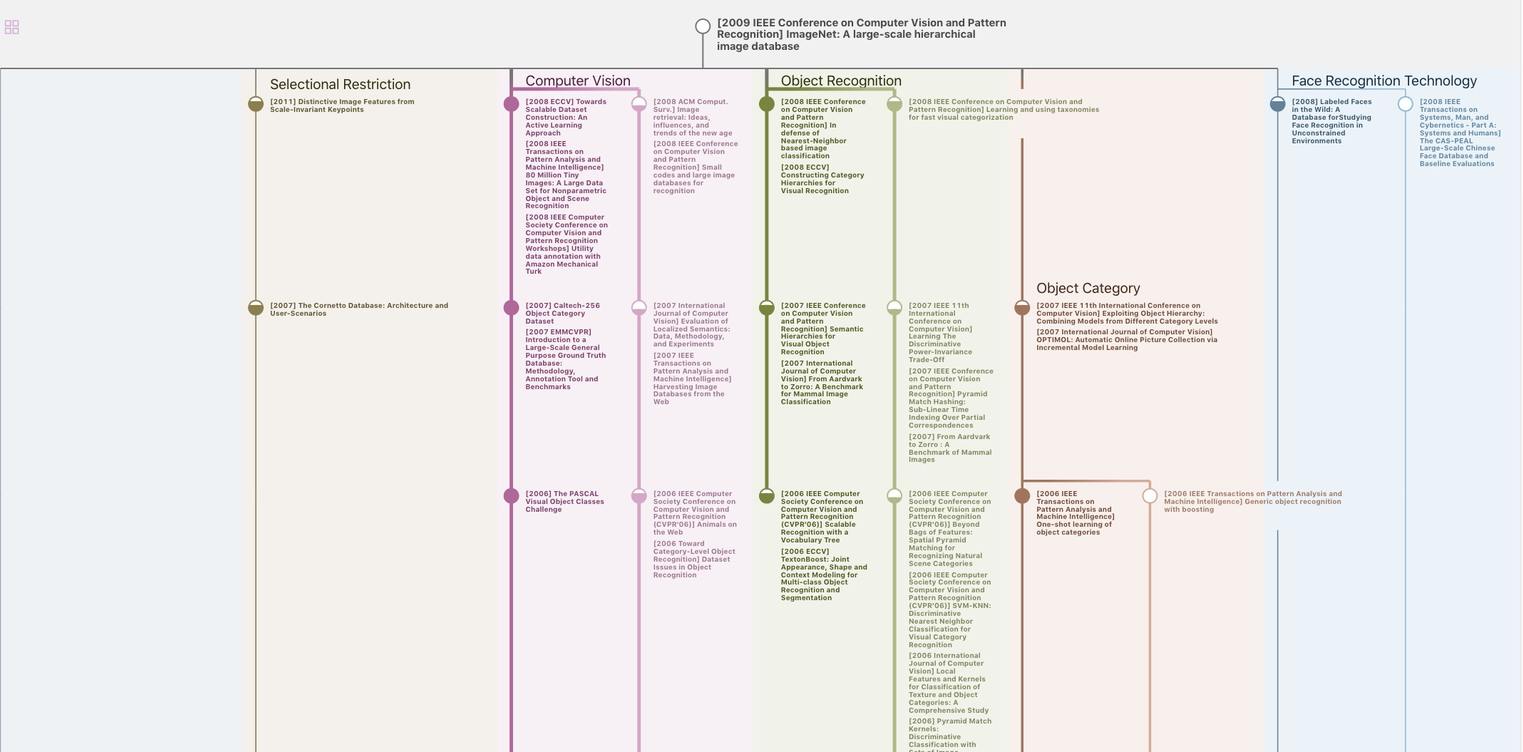
生成溯源树,研究论文发展脉络
Chat Paper
正在生成论文摘要