Scale adaptive and lightweight super-resolution with a selective hierarchical residual network
2021 5TH INTERNATIONAL CONFERENCE ON INNOVATION IN ARTIFICIAL INTELLIGENCE (ICIAI 2021)(2021)
摘要
Deep convolutional neural networks have made remarkable achievements in single-image super-resolution tasks in recent years. However, current methods do not consider the characteristics of super-resolution that the adjacent areas carry similar information. In this paper, we propose a scale adaptive and lightweight super-resolution with a selective hierarchical residual network (SHRN), which utilizes the repeated texture features. Specifically, SHRN is stacked by several selective hierarchical residual blocks (SHRB). The SHRB mainly contains a hierarchical feature fusion structure (HFFS) and a selective feature fusion structure (SFFS). The HFFS uses multiple branches to obtain multiscale features due to the varying texture size of objects. The SFFS fuses features of adjacent branches to select effective information. Plenty of experiments demonstrate that our lightweight model achieves better performance against other methods by extracting scale adaptive features and utilizing the repeated texture structure.
更多查看译文
关键词
Scale adaptive, Super-resolution, Repeated texture structure
AI 理解论文
溯源树
样例
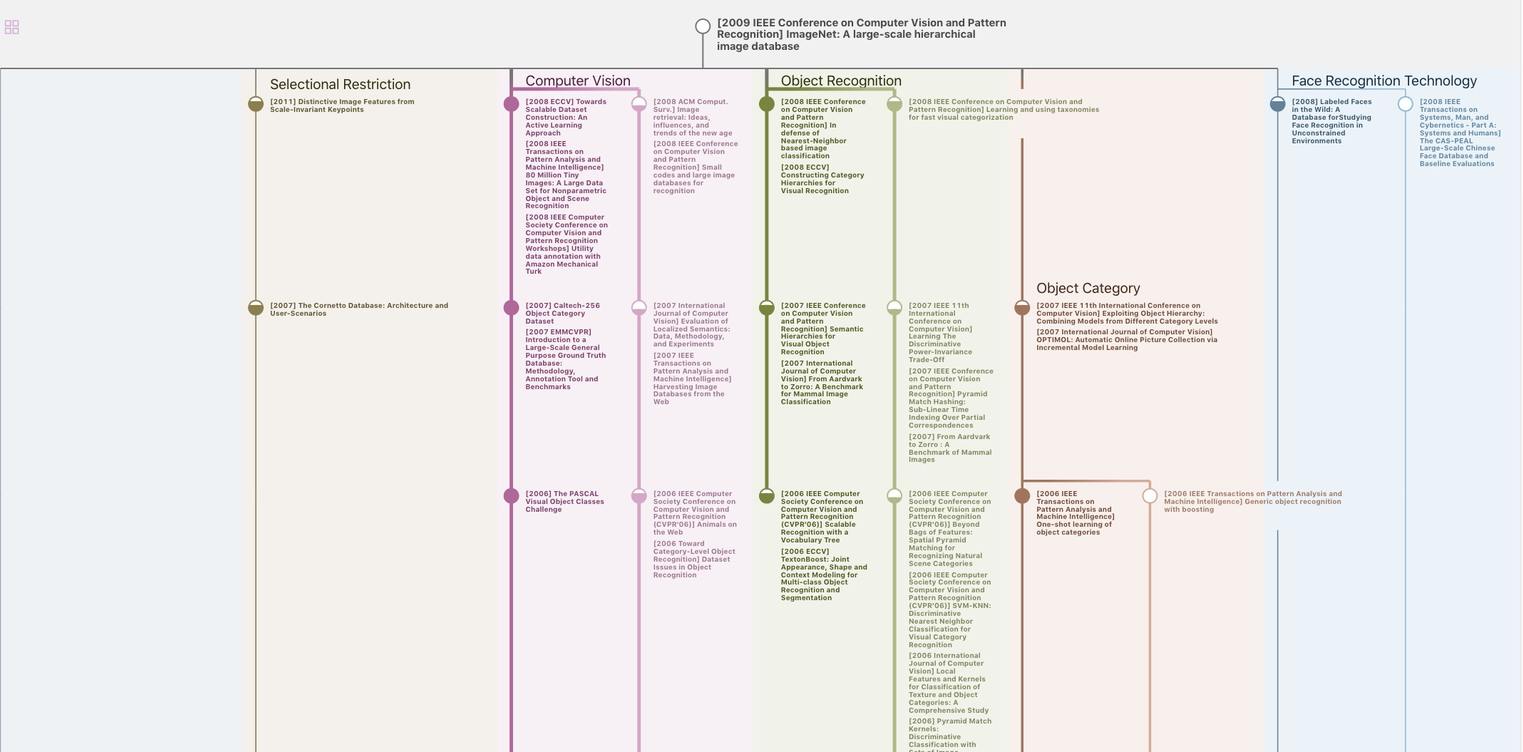
生成溯源树,研究论文发展脉络
Chat Paper
正在生成论文摘要