A Convolutional Neural Network Deep Learning Model Trained on CD Ulcers Images Accurately Identifies NSAID Ulcers
FRONTIERS IN MEDICINE(2021)
摘要
Background and Study Aims: Deep learning (DL) for video capsule endoscopy (VCE) is an emerging research field. It has shown high accuracy for the detection of Crohn's disease (CD) ulcers. Non-steroidal anti-inflammatory drugs (NSAIDS) are commonly used medications. In the small bowel, NSAIDs may cause a variety of gastrointestinal adverse events including NSAID-induced ulcers. These ulcers are the most important differential diagnosis for small bowel ulcers in patients evaluated for suspected CD. We evaluated a DL network that was trained using CD VCE ulcer images and evaluated its performance for NSAID ulcers. Patients and Methods: The network was trained using CD ulcers and normal mucosa from a large image bank created from VCE of diagnosed CD patients. NSAIDs-induced enteropathy images were extracted from the prospective Bifidobacterium breve (BIf95) trial dataset. All images were acquired from studies performed using PillCam SBIII. The area under the receiver operating curve (AUC) was used as a metric. We compared the network's AUC for detecting NSAID ulcers to that of detecting CD ulcers. Results: Overall, the CD training dataset included 17,640 CE images. The NSAIDs testing dataset included 1,605 CE images. The DL network exhibited an AUC of 0.97 (95% CI 0.97-0.98) for identifying images with NSAID mucosal ulcers. The diagnostic accuracy was similar to that obtained for CD related ulcers (AUC 0.94-0.99). Conclusions: A network trained on VCE CD ulcers similarly identified NSAID findings. As deep learning is transforming gastrointestinal endoscopy, this result should be taken into consideration in the future design and analysis of VCE deep learning applications.
更多查看译文
关键词
deep learning,capsule endoscopy,Crohn disease,anti-inflammatory agents,non-steroidal,NSAID
AI 理解论文
溯源树
样例
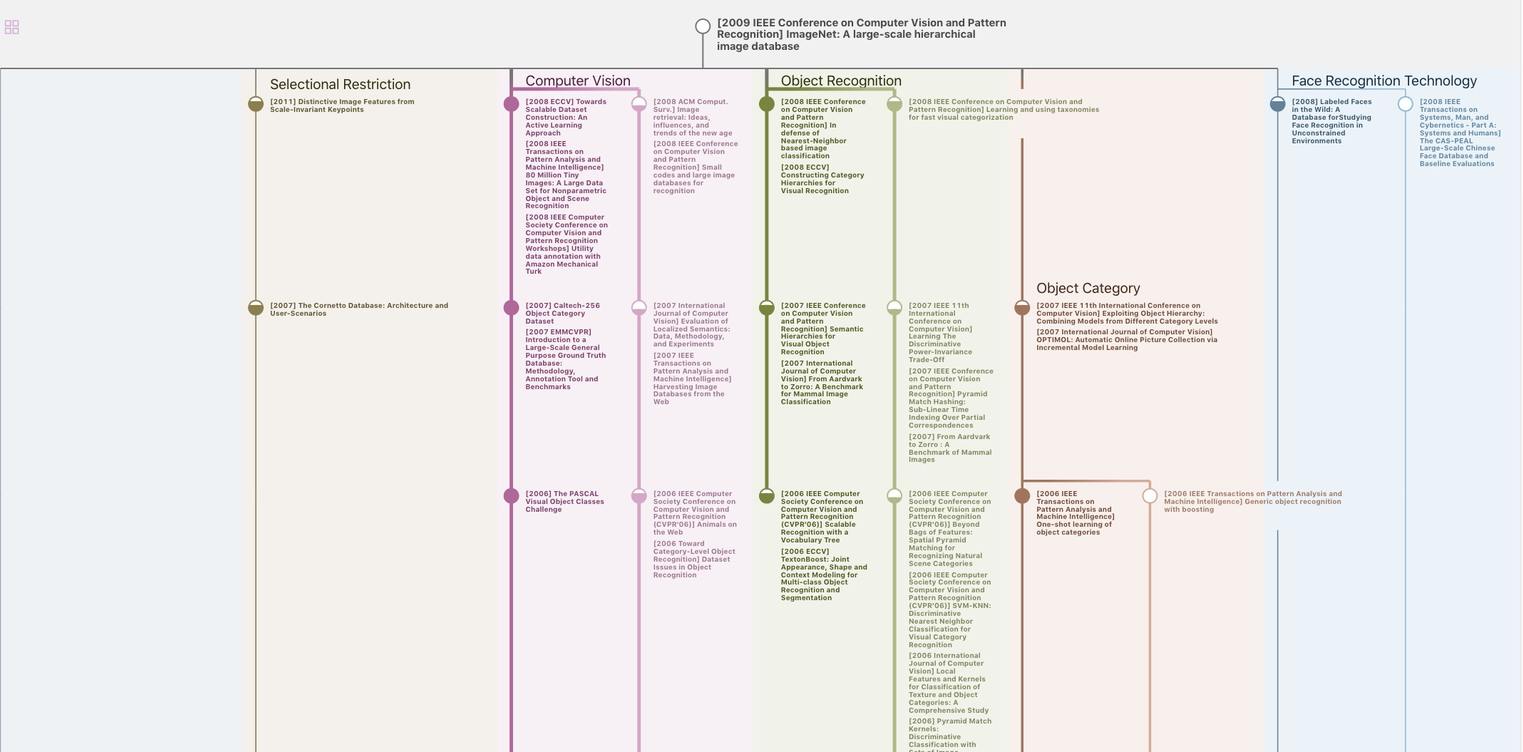
生成溯源树,研究论文发展脉络
Chat Paper
正在生成论文摘要