Relaxed Marginal Consistency for Differentially Private Query Answering.
Annual Conference on Neural Information Processing Systems(2021)
摘要
Many differentially private algorithms for answering database queries involve astep that reconstructs a discrete data distribution from noisy measurements. Thisprovides consistent query answers and reduces error, but often requires space thatgrows exponentially with dimension. PRIVATE-PGM is a recent approach that usesgraphical models to represent the data distribution, with complexity proportional tothat of exact marginal inference in a graphical model with structure determined bythe co-occurrence of variables in the noisy measurements. PRIVATE-PGM is highlyscalable for sparse measurements, but may fail to run in high dimensions with densemeasurements. We overcome the main scalability limitation of PRIVATE-PGMthrough a principled approach that relaxes consistency constraints in the estimationobjective. Our new approach works with many existing private query answeringalgorithms and improves scalability or accuracy with no privacy cost.
更多查看译文
关键词
private query
AI 理解论文
溯源树
样例
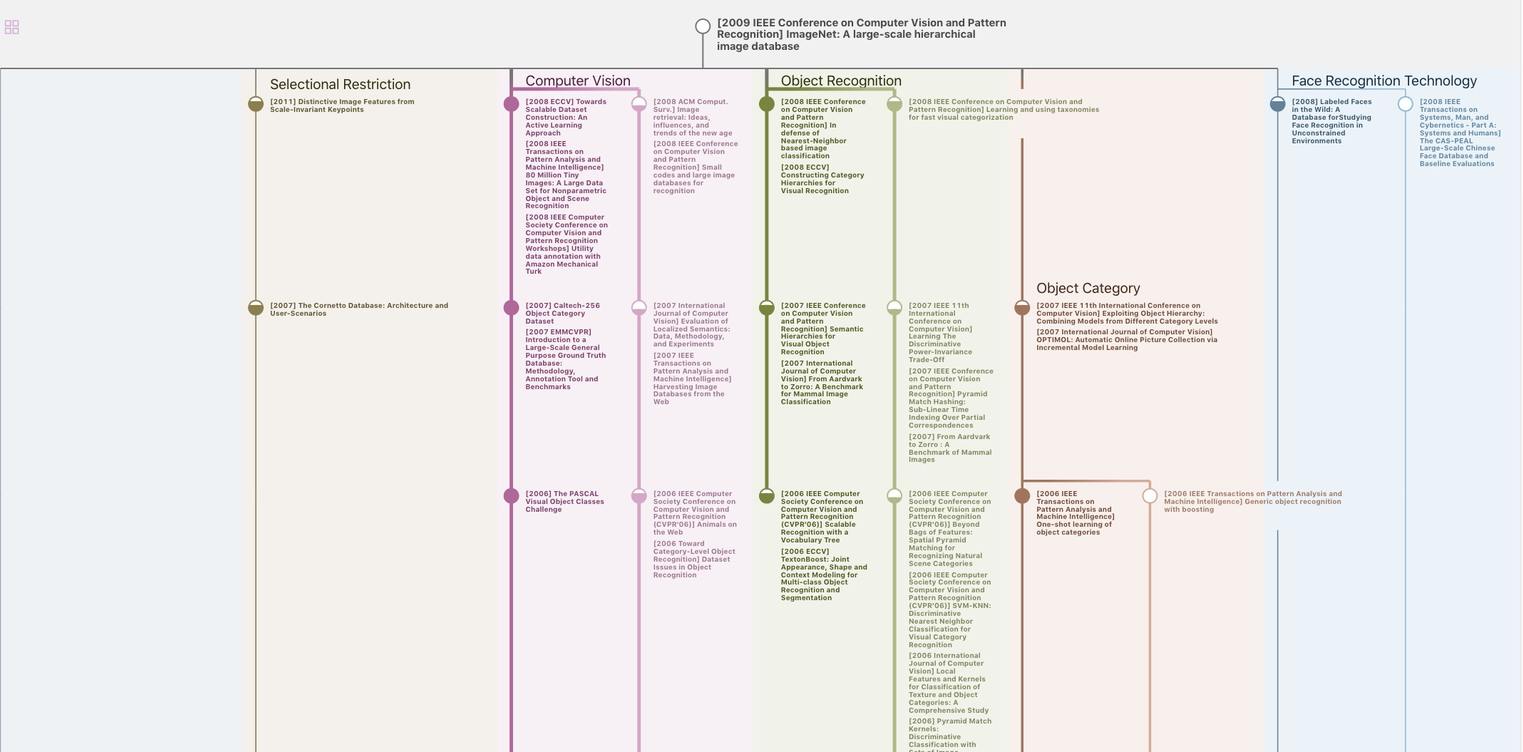
生成溯源树,研究论文发展脉络
Chat Paper
正在生成论文摘要