Large-scale local surrogate modeling of stochastic simulation experiments
COMPUTATIONAL STATISTICS & DATA ANALYSIS(2022)
摘要
Gaussian process (GP) surrogate modeling for large computer experiments is limited by cubic runtimes, especially with data from stochastic simulations with input-dependent noise. A popular workaround to reduce computational complexity involves local approximation (e.g., LAGP). However, LAGP has only been vetted in deterministic settings. A recent variation utilizing inducing points (LIGP) for additional sparsity improves upon LAGP on the speed-vs-accuracy frontier. The authors show that another benefit of LIGP over LAGP is that (local) nugget estimation for stochastic responses is more natural, especially when designs contain substantial replication as is common when attempting to separate signal from noise. Woodbury identities, extended in LIGP from inducing points to replicates, afford efficient computation in terms of unique design locations only. This increases the amount of local data (i.e., the neighborhood size) that may be incorporated without additional flops, thereby enhancing statistical efficiency. Performance of the authors' LIGP upgrades is illustrated on benchmark data and real-world stochastic simulation experiments, including an options pricing control framework. Results indicate that LIGP provides more accurate prediction and uncertainty quantification for varying data dimension and replication strategies versus modern alternatives. (C) 2022 Elsevier B.V. All rights reserved.
更多查看译文
关键词
Gaussian process approximation, Kriging, Divide-and-conquer, Input-dependent noise (heteroskedasticity), Replication, Woodbury formula
AI 理解论文
溯源树
样例
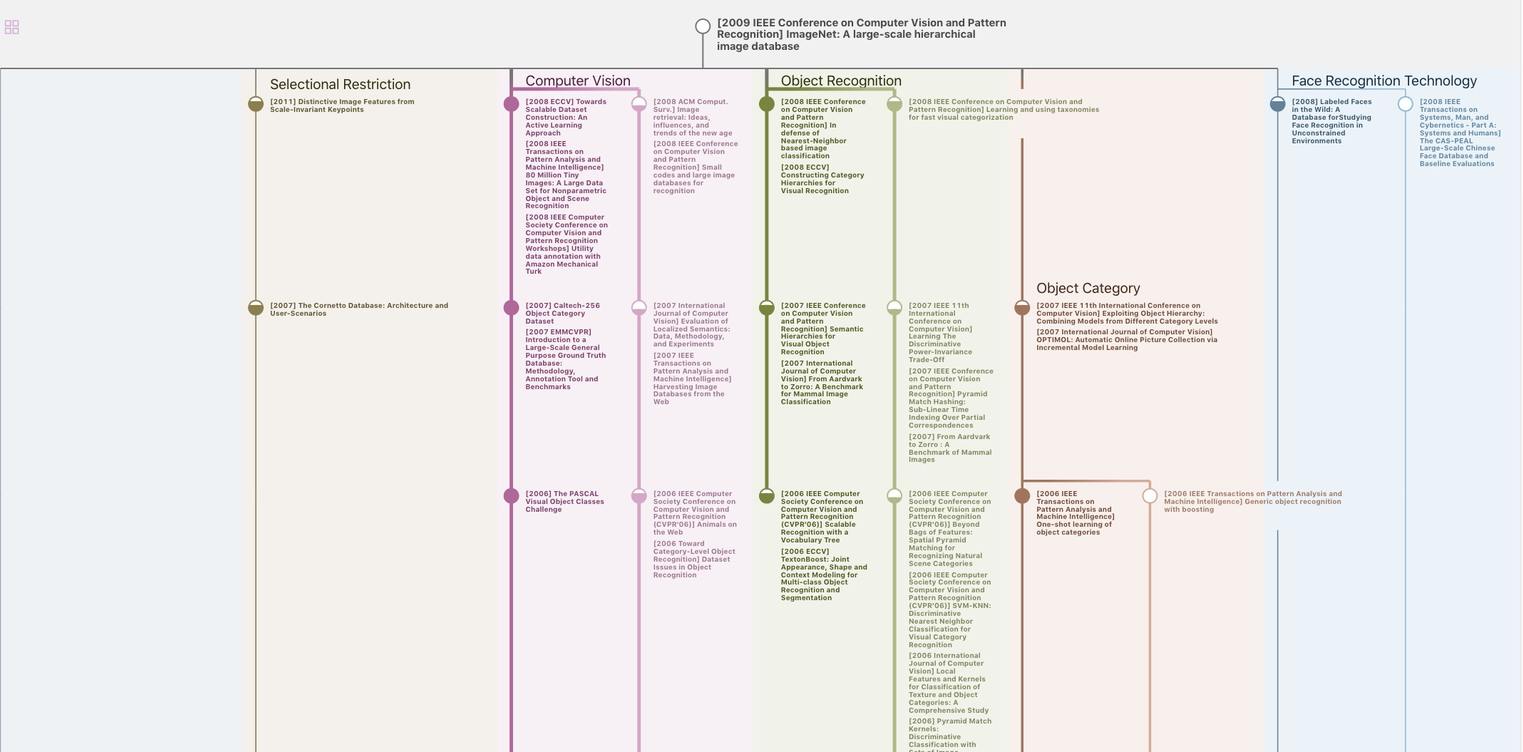
生成溯源树,研究论文发展脉络
Chat Paper
正在生成论文摘要