Graph-Based Object Detection Enhancement for Symbolic Engineering Drawings
DOCUMENT ANALYSIS AND RECOGNITION, ICDAR 2021 WORKSHOPS, PT I(2021)
摘要
The identification of graphic symbols and interconnections is a primary task in the digitization of symbolic engineering diagram images like circuit diagrams. Recent approaches propose the use of Convolutional Neural Networks to the identification of symbols in engineering diagrams. Although recall and precision from CNN based object recognition algorithms are high, false negatives result in some input symbols being missed or misclassified. The missed symbols induce errors in the circuit level features of the extracted circuit, which can be identified using graph level analysis. In this work, a custom annotated printed circuit image set, which is made publicly available in conjunction with the source code of the experiments of this paper, is used to fine-tune a Faster RCNN network to recognise component symbols and blob detection to identify inter-connections between symbols to generate a graph representation of the extracted circuit components. The graph structure is then analysed using graph convolutional neural networks and node degree comparison to identify graph anomalies potentially resulting from false negatives from the object recognition module. Anomaly predictions are then used to identify image regions with potential missed symbols, which are subject to image transforms and re-input to the Faster RCNN, which results in a significant improvement in component recall, which increases to 91% on the test set. The general tools used by the analysis pipeline can also be applied to other Engineering Diagrams with the availability of similar datasets.
更多查看译文
关键词
Graph convolutional network, Circuit diagram, Graph refinement
AI 理解论文
溯源树
样例
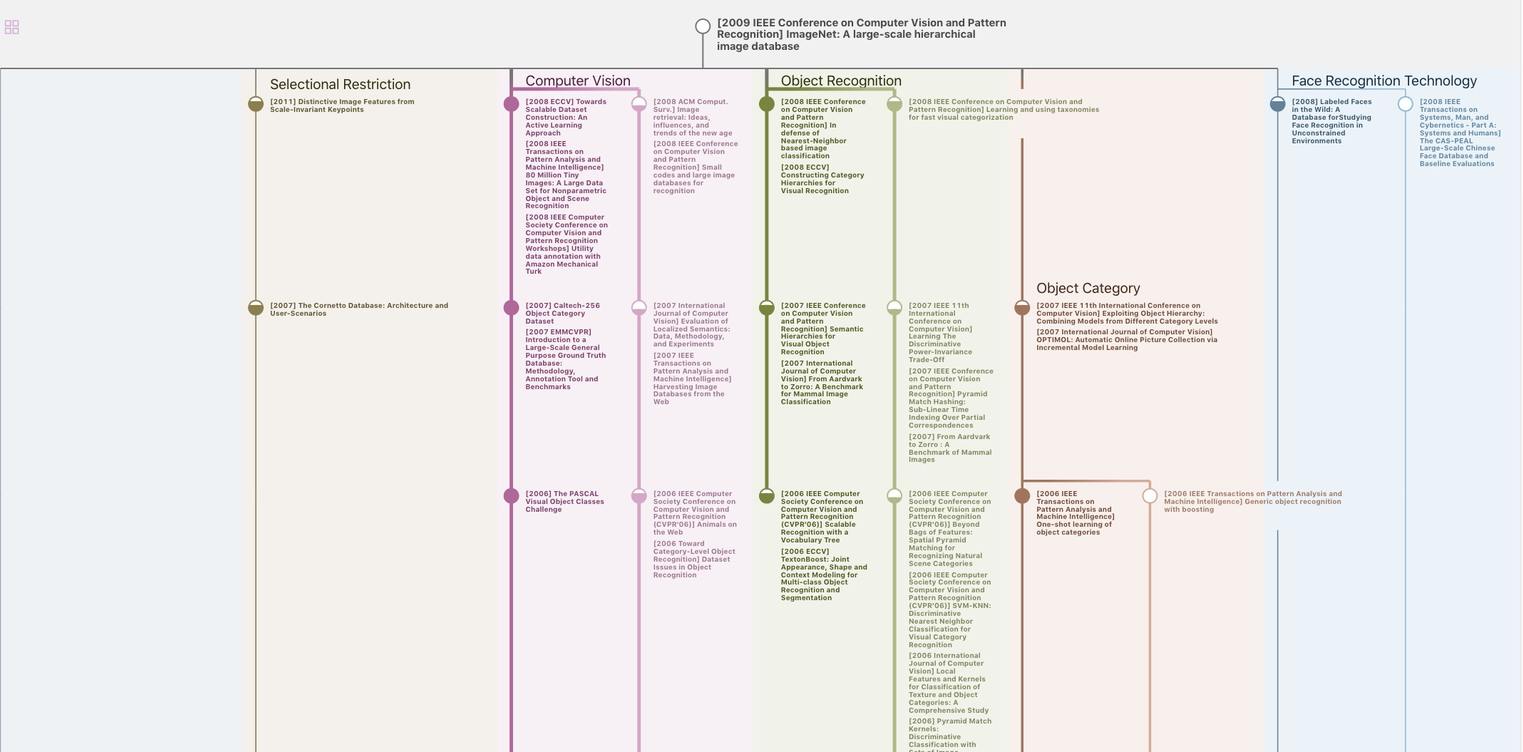
生成溯源树,研究论文发展脉络
Chat Paper
正在生成论文摘要