Histological Grade Of Endometrioid Endometrial Cancer And Relapse Risk Can Be Predicted With Machine Learning From Gene Expression Data
CANCERS(2021)
摘要
Simple Summary Implementing machine learning methods into the RNA-seq data analysis pipelines can further improve the efficiency of data utilization in clinical decision making. In this article, we present how machine learning methods can be used to go one step further in data analysis of the global gene expression datasets, namely, to develop models that are able to classify individual cancer samples based on well characterized reference samples. We used the publicly available endometrial cancer sample RNA-seq datasets of the TCGA project to develop a model that can separate G1 and G3 cancer samples with an accuracy of 85%. Our model could also further stratify G2 samples into high-risk and low-risk subgroups. Moreover, with an iterative retraining approach, we could subselect twelve genes that performed similarly in the stratification. Our results were validated by the survival data of the patients. The tumor grade of endometrioid endometrial cancer is used as an independent marker of prognosis and a key component in clinical decision making. It is reported that between grades 1 and 3, however, the intermediate grade 2 carries limited information; thus, patients with grade 2 tumors are at risk of both under- and overtreatment. We used RNA-sequencing data from the TCGA project and machine learning to develop a model which can correctly classify grade 1 and grade 3 samples. We used the trained model on grade 2 patients to subdivide them into low-risk and high-risk groups. With iterative retraining, we selected the most relevant 12 transcripts to build a simplified model without losing accuracy. Both models had a high AUC of 0.93. In both cases, there was a significant difference in the relapse-free survivals of the newly identified grade 2 subgroups. Both models could identify grade 2 patients that have a higher risk of relapse. Our approach overcomes the subjective components of the histological evaluation. The developed method can be automated to perform a prescreening of the samples before a final decision is made by pathologists. Our translational approach based on machine learning methods could allow for better therapeutic planning for grade 2 endometrial cancer patients.
更多查看译文
关键词
endometrium, biomarkers, endometrial cancer, machine learning, elastic-net, relapse-free survival, TCGA, RNA-seq, tumor grade, fertility preservation
AI 理解论文
溯源树
样例
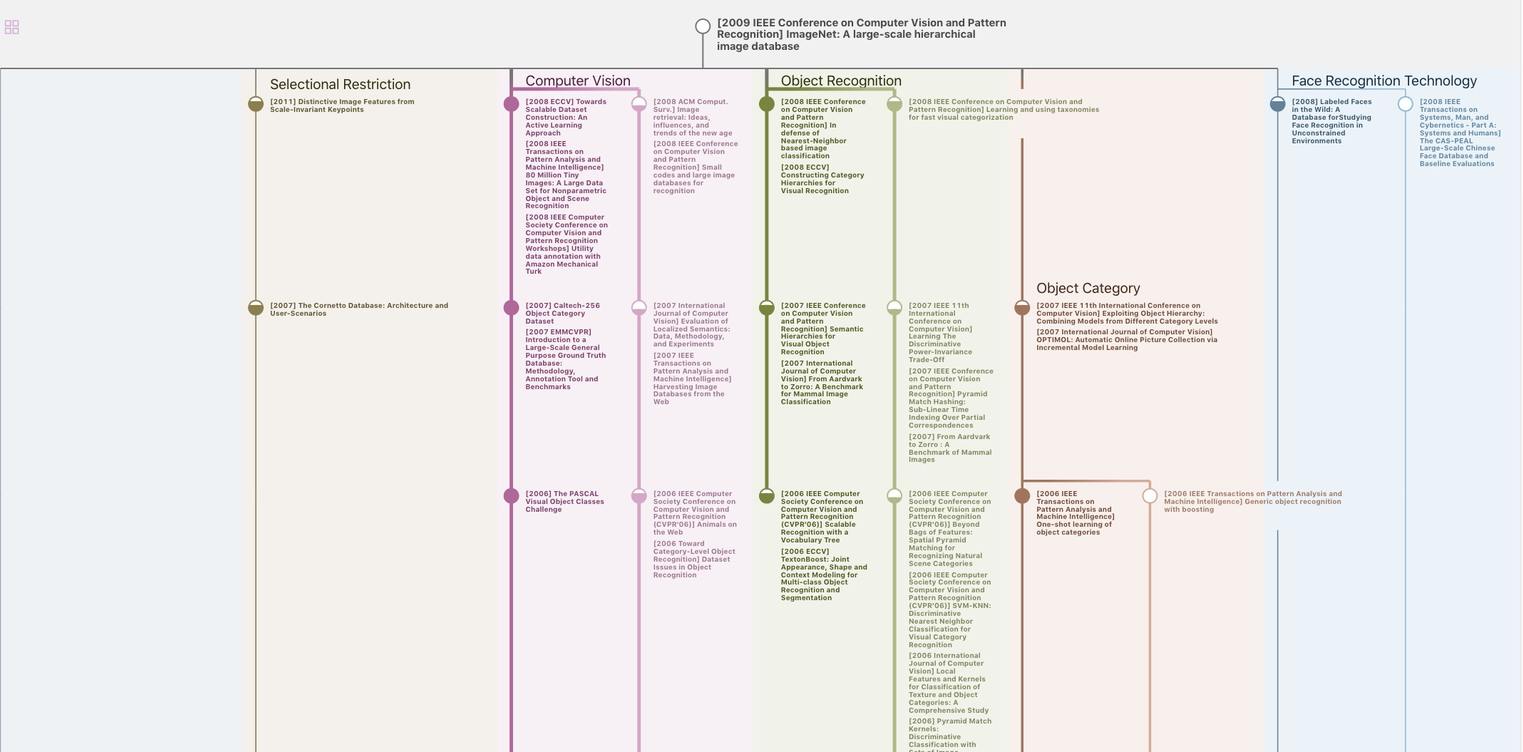
生成溯源树,研究论文发展脉络
Chat Paper
正在生成论文摘要