Neural networks based domain name generation
Journal of Information Security and Applications(2021)
摘要
Domain generation algorithm (DGA) is used by botnets to build a stealthy command and control (C&C) communication channel between the C&C server and the bots. A DGA can periodically produce a large number of pseudo-random algorithmically generated domains (AGDs), a few of which direct the bots to the C&C server. AGD detection algorithms provide a lightweight, promising solution in response to the existing DGA techniques. In the constantly evolving attacker–defender game, attackers may seek more advanced DGA techniques to gain a better chance of evading detection by defenders. In this paper, we propose a new DGA, namely a neural networks-based domain name generation (NDG) architecture. NDG is based on a variational autoencoder (VAE), where the encoder and decoder networks use stacked gated convolutional neural networks (GCNNs) to learn the contextual structure hierarchically. NDG is experimentally validated using a set of state-of-the-art AGD detection algorithms. The existing DGAs of different classes following a DGA taxonomy are used to benchmark NDG. NDG shows the best overall anti-detection performance among all tested DGAs. We also demonstrate that NDG is effective in benchmarking AGD detection algorithms.
更多查看译文
关键词
Domain generation algorithm,Malicious domain name,Classification,Variational autoencoder,Deep learning
AI 理解论文
溯源树
样例
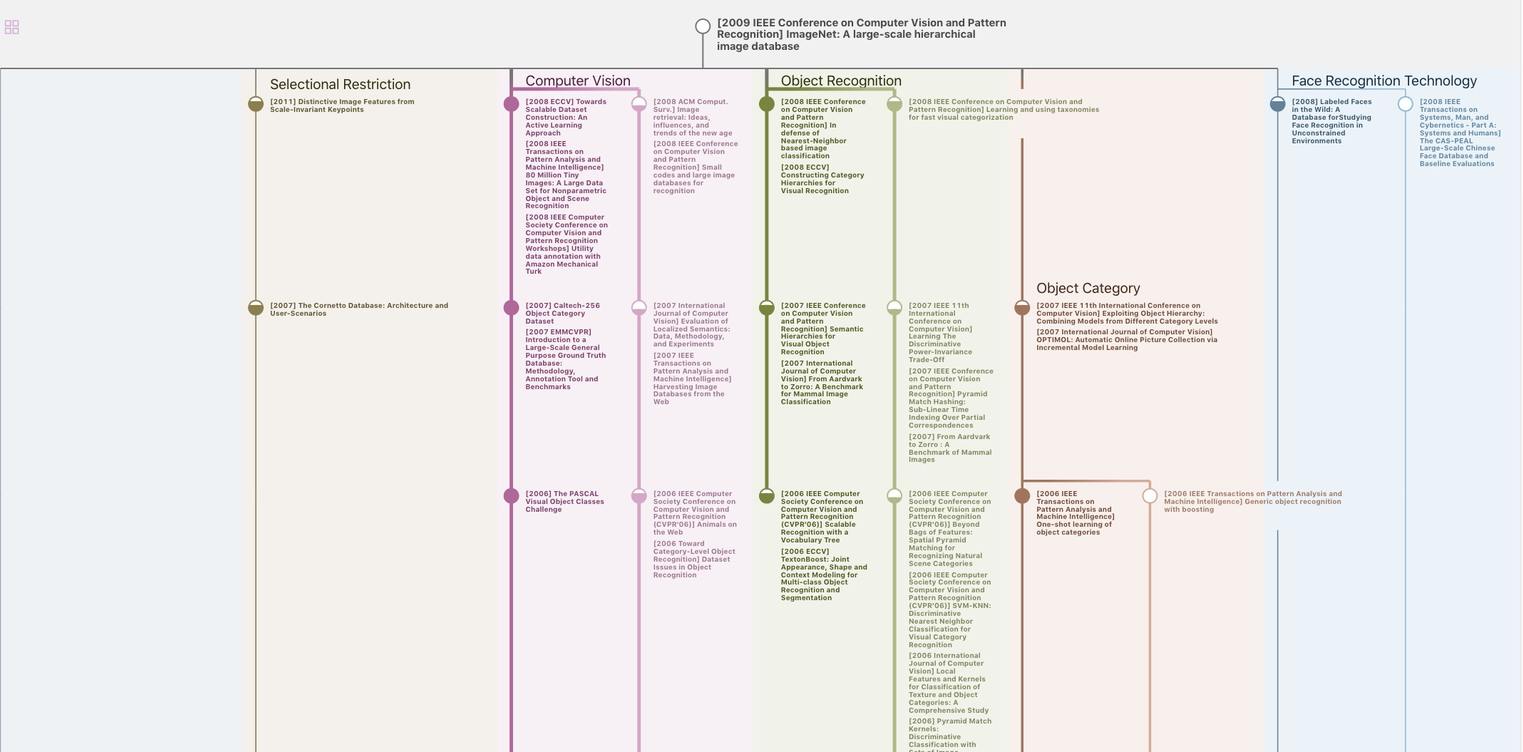
生成溯源树,研究论文发展脉络
Chat Paper
正在生成论文摘要