Energy Attack: On Transferring Adversarial Examples
arXiv (Cornell University)(2021)
Abstract
In this work we propose Energy Attack, a transfer-based black-box $L_\infty$-adversarial attack. The attack is parameter-free and does not require gradient approximation. In particular, we first obtain white-box adversarial perturbations of a surrogate model and divide these perturbations into small patches. Then we extract the unit component vectors and eigenvalues of these patches with principal component analysis (PCA). Base on the eigenvalues, we can model the energy distribution of adversarial perturbations. We then perform black-box attacks by sampling from the perturbation patches according to their energy distribution, and tiling the sampled patches to form a full-size adversarial perturbation. This can be done without the available access to victim models. Extensive experiments well demonstrate that the proposed Energy Attack achieves state-of-the-art performance in black-box attacks on various models and several datasets. Moreover, the extracted distribution is able to transfer among different model architectures and different datasets, and is therefore intrinsic to vision architectures.
MoreTranslated text
Key words
adversarial examples,attack,energy
AI Read Science
Must-Reading Tree
Example
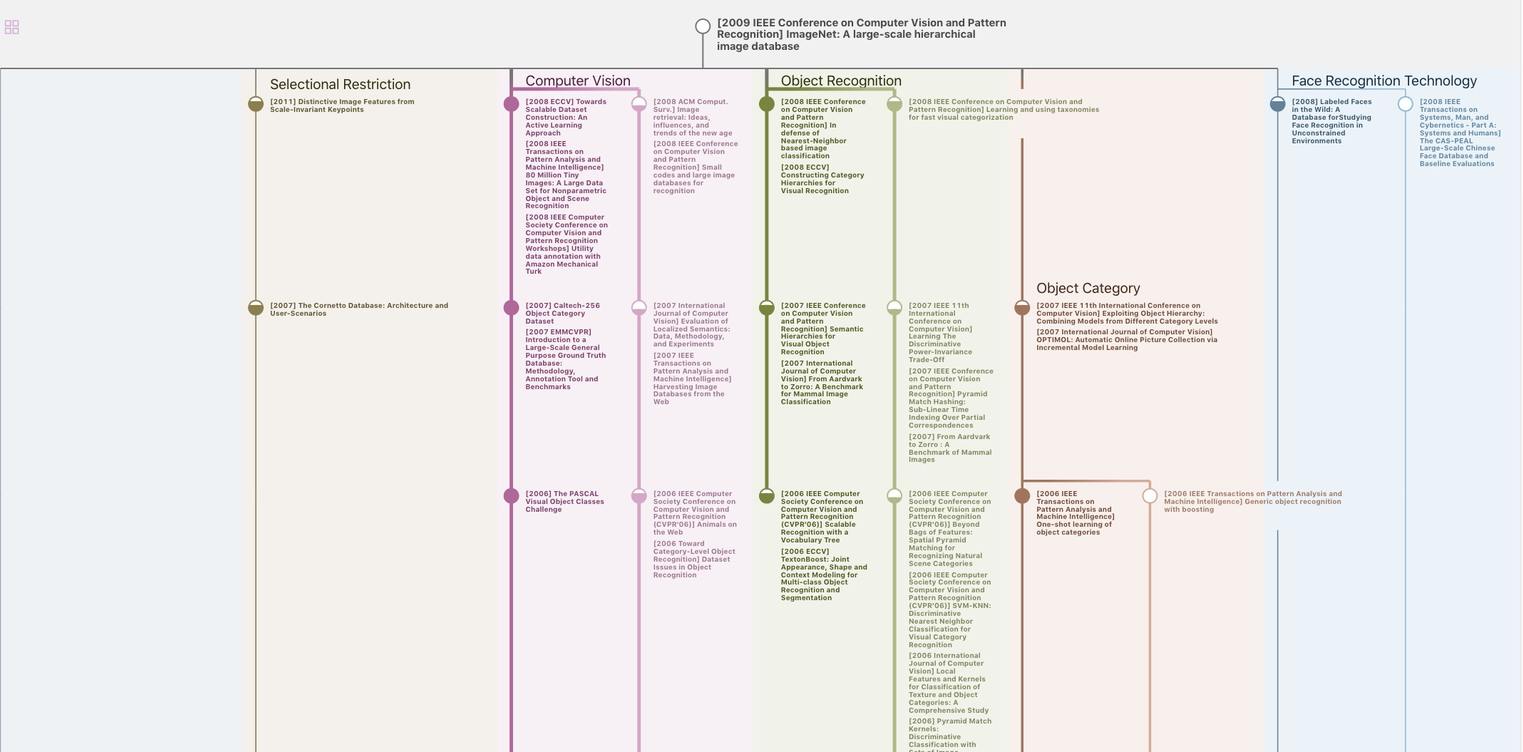
Generate MRT to find the research sequence of this paper
Chat Paper
Summary is being generated by the instructions you defined