Posterior Concentration Rates for Bayesian O'Sullivan Penalized Splines
arxiv(2021)
摘要
The O'Sullivan penalized splines approach is a popular frequentist approach for nonparametric regression. Thereby, the unknown regression function is expanded in a rich spline basis and a roughness penalty based on the integrated squared $q$th derivative is used for regularization. While the asymptotic properties of O'Sullivan penalized splines in a frequentist setting have been investigated extensively, the theoretical understanding of the Bayesian counterpart has been missing so far. In this paper, we close this gap and study the asymptotics of the Bayesian counterpart of the frequentist O-splines approach. We derive sufficient conditions for the entire posterior distribution to concentrate around the true regression function at near optimal rate. Our results show that posterior concentration at near optimal rate can be achieved with a faster rate for the number of spline knots than the slow regression spline rate that is commonly being used. Furthermore, posterior concentration at near optimal rate can be achieved with several different hyperpriors on the smoothing variance such as a Gamma and a Weibull hyperprior.
更多查看译文
关键词
posterior concentration rates,bayesian
AI 理解论文
溯源树
样例
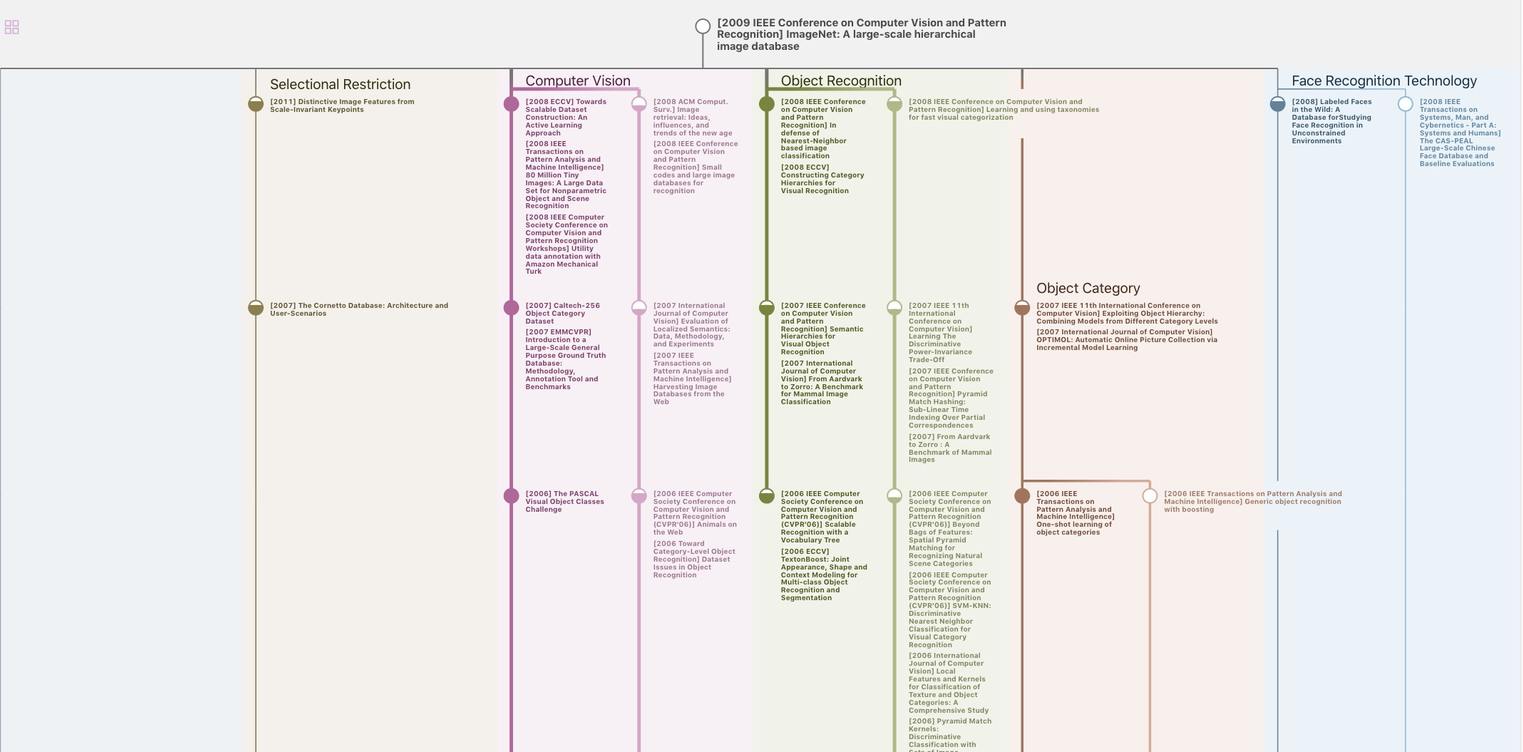
生成溯源树,研究论文发展脉络
Chat Paper
正在生成论文摘要