Iterated Vector Fields and Conservatism, with Applications to Federated Learning
International Conference on Algorithmic Learning Theory (ALT)(2022)
摘要
We study iterated vector fields and investigate whether they are conservative, in the sense that they are the gradient of some scalar-valued function. We analyze the conservatism of various iterated vector fields, including gradient vector fields associated to loss functions of generalized linear models. We relate this study to optimization and derive novel convergence results for federated learning algorithms. In particular, we show that for certain classes of functions (including non-convex functions), federated averaging is equivalent to gradient descent on a surrogate loss function. Finally, we discuss a variety of open questions spanning topics in geometry, dynamical systems, and optimization.
更多查看译文
关键词
federated learning,vector
AI 理解论文
溯源树
样例
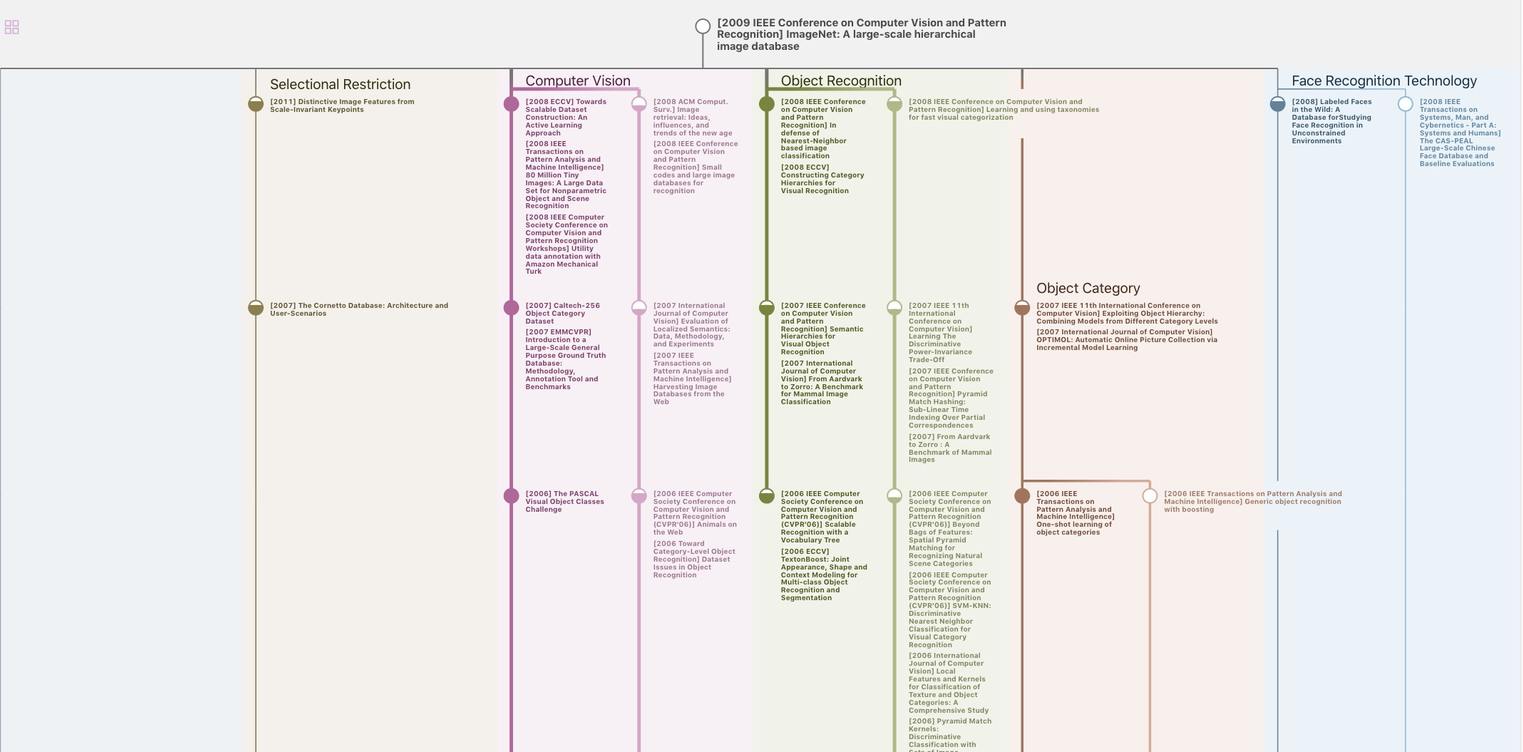
生成溯源树,研究论文发展脉络
Chat Paper
正在生成论文摘要