Training algorithm matters for the performance of neural network potential: A case study of Adam and the Kalman filter optimizers.
JOURNAL OF CHEMICAL PHYSICS(2021)
摘要
One hidden yet important issue for developing neural network potentials (NNPs) is the choice of training algorithm. In this article, we compare the performance of two popular training algorithms, the adaptive moment estimation algorithm (Adam) and the extended Kalman filter algorithm (EKF), using the Behler-Parrinello neural network and two publicly accessible datasets of liquid water [Morawietz et al., Proc. Natl. Acad. Sci. U. S. A. 113, 8368-8373, (2016) and Cheng et al., Proc. Natl. Acad. Sci. U. S. A. 116, 1110-1115, (2019)]. This is achieved by implementing EKF in TensorFlow. It is found that NNPs trained with EKF are more transferable and less sensitive to the value of the learning rate, as compared to Adam. In both cases, error metrics of the validation set do not always serve as a good indicator for the actual performance of NNPs. Instead, we show that their performance correlates well with a Fisher information based similarity measure.
更多查看译文
关键词
potential,algorithm matters,training,performance
AI 理解论文
溯源树
样例
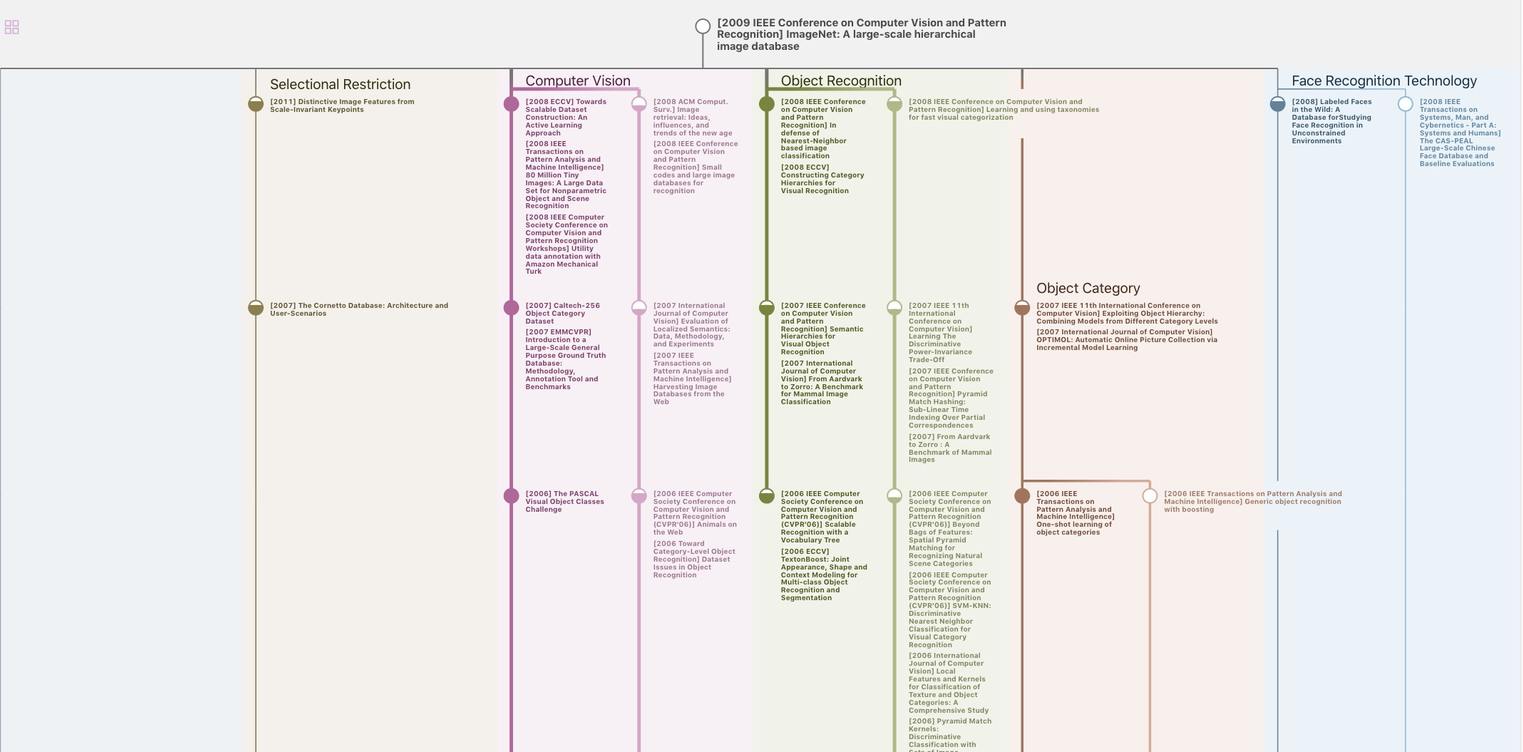
生成溯源树,研究论文发展脉络
Chat Paper
正在生成论文摘要