Signal-domain representation of symbolic music for learning embedding spaces
arxiv(2021)
摘要
A key aspect of machine learning models lies in their ability to learn efficient intermediate features. However, the input representation plays a crucial role in this process, and polyphonic musical scores remain a particularly complex type of information. In this paper, we introduce a novel representation of symbolic music data, which transforms a polyphonic score into a continuous signal. We evaluate the ability to learn meaningful features from this representation from a musical point of view. Hence, we introduce an evaluation method relying on principled generation of synthetic data. Finally, to test our proposed representation we conduct an extensive benchmark against recent polyphonic symbolic representations. We show that our signal-like representation leads to better reconstruction and disentangled features. This improvement is reflected in the metric properties and in the generation ability of the space learned from our signal-like representation according to music theory properties.
更多查看译文
关键词
symbolic music,spaces,learning,signal-domain
AI 理解论文
溯源树
样例
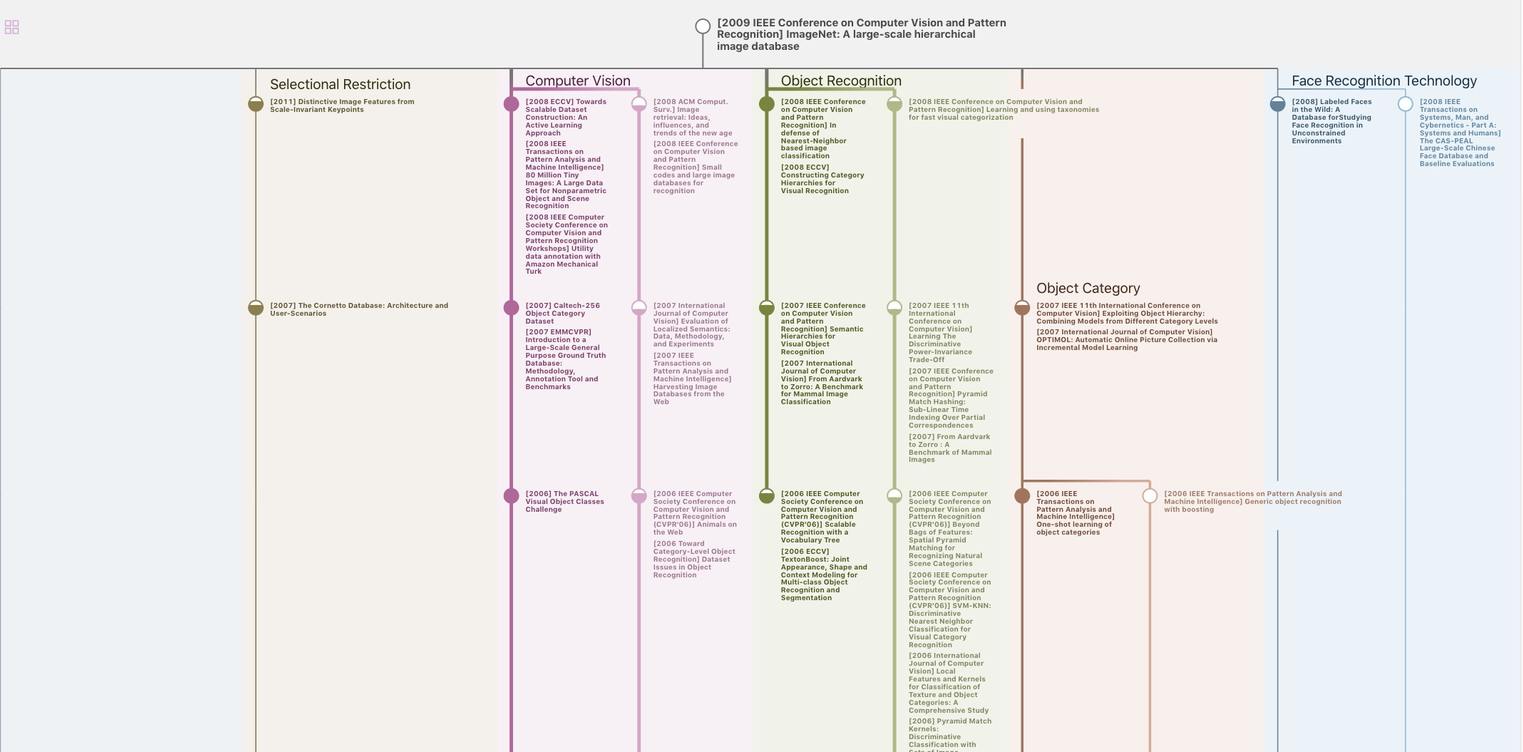
生成溯源树,研究论文发展脉络
Chat Paper
正在生成论文摘要