CIDER: An Expressive, Nonlocal Feature Set for Machine Learning Density Functionals with Exact Constraints br
JOURNAL OF CHEMICAL THEORY AND COMPUTATION(2022)
摘要
Machine learning (ML) has recently gained attention as a means to develop more accurate exchange-correlation (XC) functionals fordensity functional theory, but functionals developed thus far need to beimproved on several metrics, including accuracy, numerical stability, andtransferability across chemical space. In this work, we introduce a set ofnonlocal features of the density called the CIDER formalism, which we use totrain a Gaussian process model for the exchange energy that obeys the criticaluniform scaling rule for exchange. The resulting CIDER exchange functionalis significantly more accurate than any semilocal functional tested here, and ithas good transferability across main-group molecules. This work thereforeserves as an initial step toward more accurate exchange functionals, and it also introduces useful techniques for developing robust, physics-informed XC models via ML
更多查看译文
关键词
Computational Chemistry
AI 理解论文
溯源树
样例
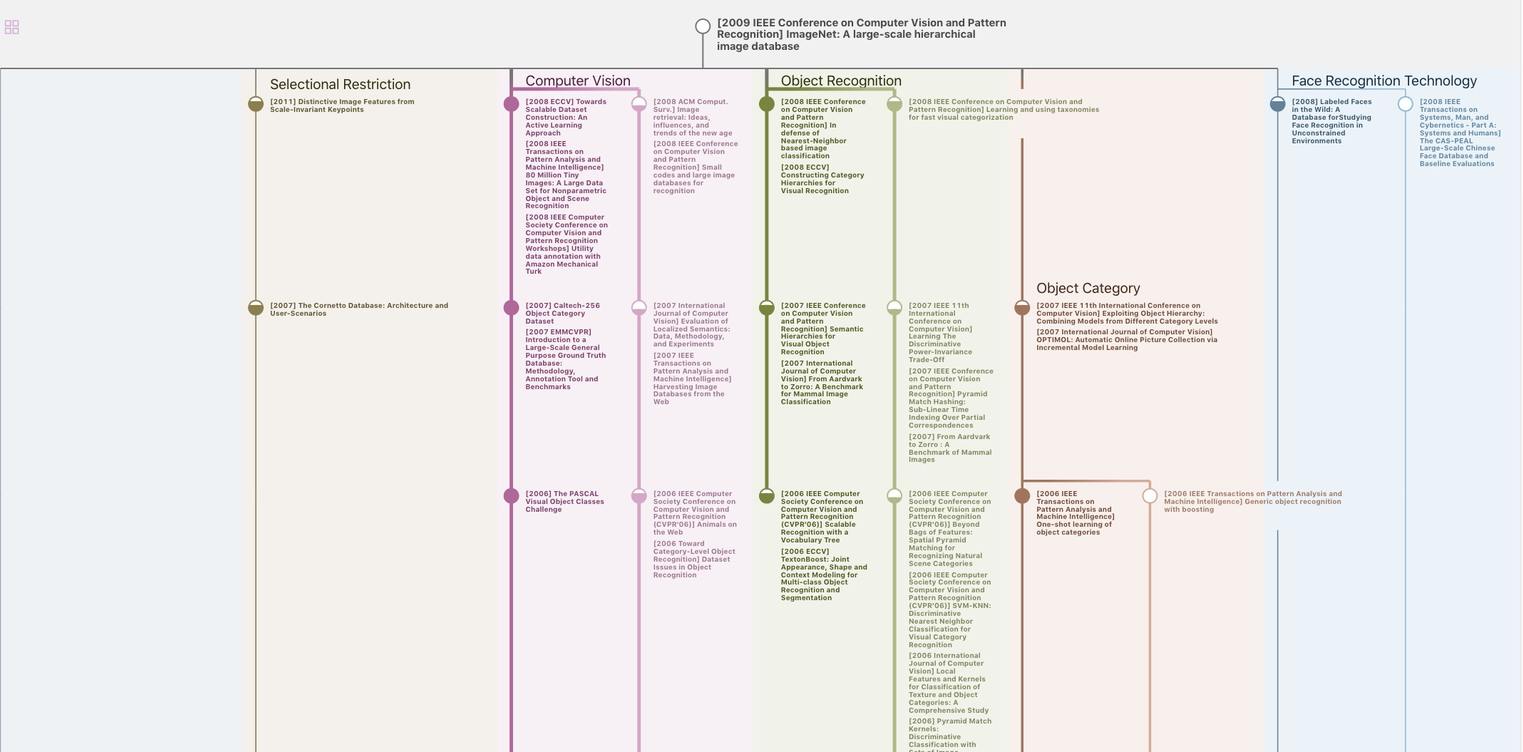
生成溯源树,研究论文发展脉络
Chat Paper
正在生成论文摘要