Computation Of A Probabilistic And Anisotropic Failure Metric On The Aortic Wall Using A Machine Learning-Based Surrogate Model
COMPUTERS IN BIOLOGY AND MEDICINE(2021)
摘要
Scalar-valued failure metrics are commonly used to assess the risk of aortic aneurysm rupture and dissection, which occurs under hypertensive blood pressures brought on by extreme emotional or physical stress. To compute failure metrics under an elevated blood pressure, a classical patient-specific computer model consists of multiple computation steps involving inverse and forward analyses. These classical procedures may be impractical for time-sensitive clinical applications that require prompt feedback to clinicians. In this study, we developed a machine learning-based surrogate model to directly predict a probabilistic and anisotropic failure metric, namely failure probability (FP), on the aortic wall using aorta geometries at the systolic and diastolic phases. Ascending thoracic aortic aneurysm (ATAA) geometries of 60 patients were obtained from their CT scans, and biaxial mechanical testing data of ATAA tissues from 79 patients were collected. Finite element simulations were used to generate datasets for training, validation, and testing of the ML-surrogate model. The testing results demonstrated that the ML-surrogate can compute the maximum FP failure metric, with 0.42% normalized mean absolute error, in 1 s. To compare the performance of the ML-predicted probabilistic FP metric with other isotropic or deterministic metrics, a numerical case study was performed using synthetic "baseline" data. Our results showed that the probabilistic FP metric had more discriminative power than the deterministic Tsai-Hill metric, isotropic maximum principal stress, and aortic diameter criterion.
更多查看译文
关键词
Failure metric, Surrogate model, Aortic aneurysm, Machine learning, Neural network
AI 理解论文
溯源树
样例
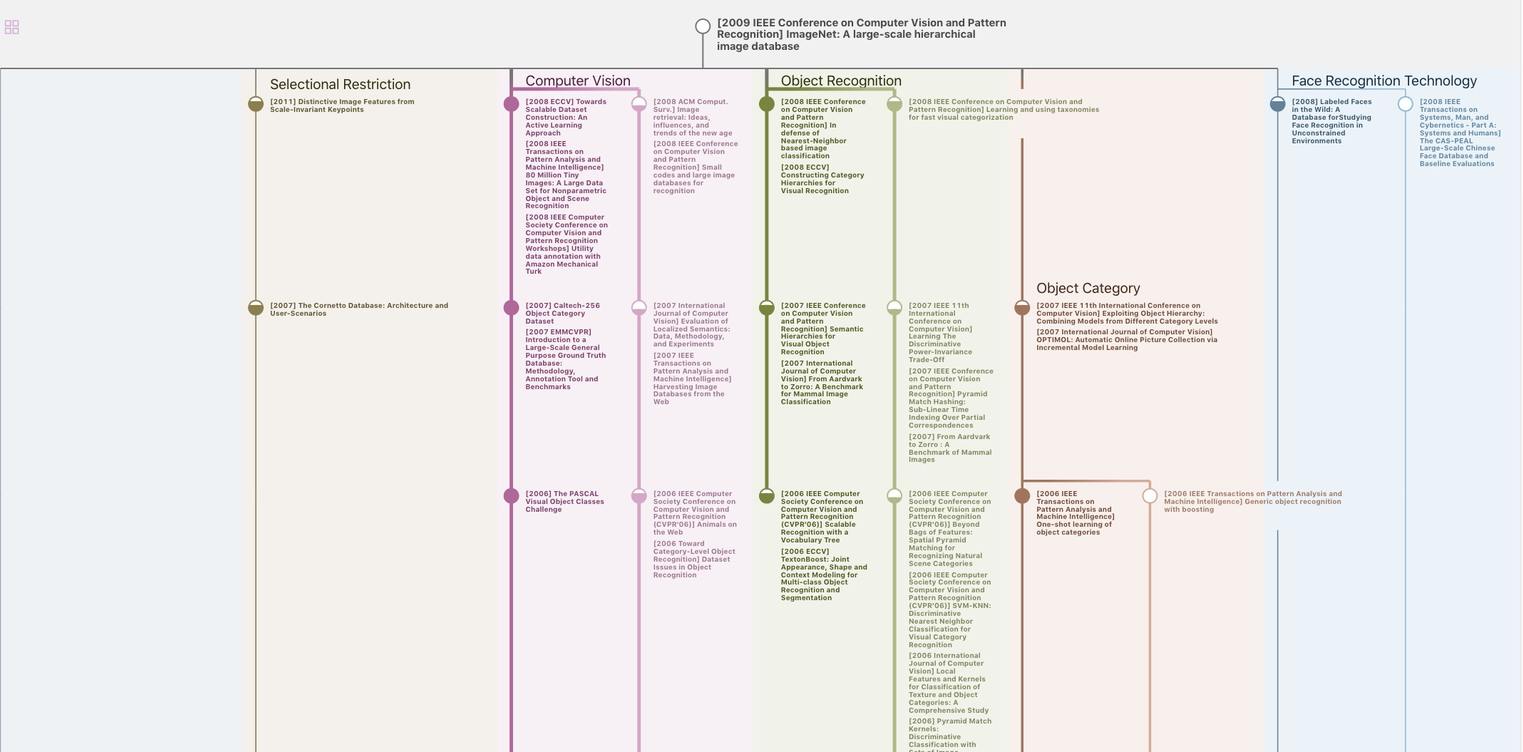
生成溯源树,研究论文发展脉络
Chat Paper
正在生成论文摘要